Amazon Research Awards (ARA) provides unrestricted funds and AWS Promotional Credits to academic researchers investigating various research topics in multiple disciplines. This cycle, ARA received many excellent research proposals from across the world and today is publicly announcing 105 award recipients who represent 51 universities in 15 countries.
This announcement includes awards funded under six call for proposals during the fall 2023 cycle: AI for Information Security, Automated Reasoning, AWS AI, AWS Cryptography and Privacy, AWS Database Services, and Sustainability. Proposals were reviewed for the quality of their scientific content and their potential to impact both the research community and society.
Additionally, Amazon encourages the publication of research results, presentations of research at Amazon offices worldwide, and the release of related code under open-source licenses.
Recipients have access to more than 300 Amazon public datasets and can utilize AWS AI/ML services and tools through their AWS Promotional Credits. Recipients also are assigned an Amazon research contact who offers consultation and advice, along with opportunities to participate in Amazon events and training sessions.
“We received a fantastic response to the cryptography and privacy engineering’s call for proposals. This was the first time we offered ARAs for cryptography and privacy, and the response far exceeded our expectations, in terms of both the number and quality of the proposals,” said Rod Chapman, senior principal applied scientist with AWS Cryptography. “Advanced cryptography plays a crucial role in building trust with our customers and regulators, especially in emerging domains such as cryptographic computing, generative AI, and privacy-preserving applications. We look forward to working with the new principal investigators to bring ever more impactful cryptographic technologies to fruition.”
“Given that data is central to Amazon’s core businesses, I am excited by this opportunity to collaborate with universities on cutting-edge technologies for modern database systems,” said Doug Terry, vice president and distinguished scientist in AWS Database and AI Leadership. “These Amazon Research Awards allow us to support projects that have the potential for substantial advancement in important areas from correctness testing of SQL queries to new data models for generative AI applications.”
ARA funds proposals throughout the year in a variety of research areas. Applicants are encouraged to visit the ARA call for proposals page for more information or send an email to be notified of future open calls.
The tables below list, in alphabetical order by last name, fall 2023 cycle call-for-proposal recipients, sorted by research area.
AI for Information Security
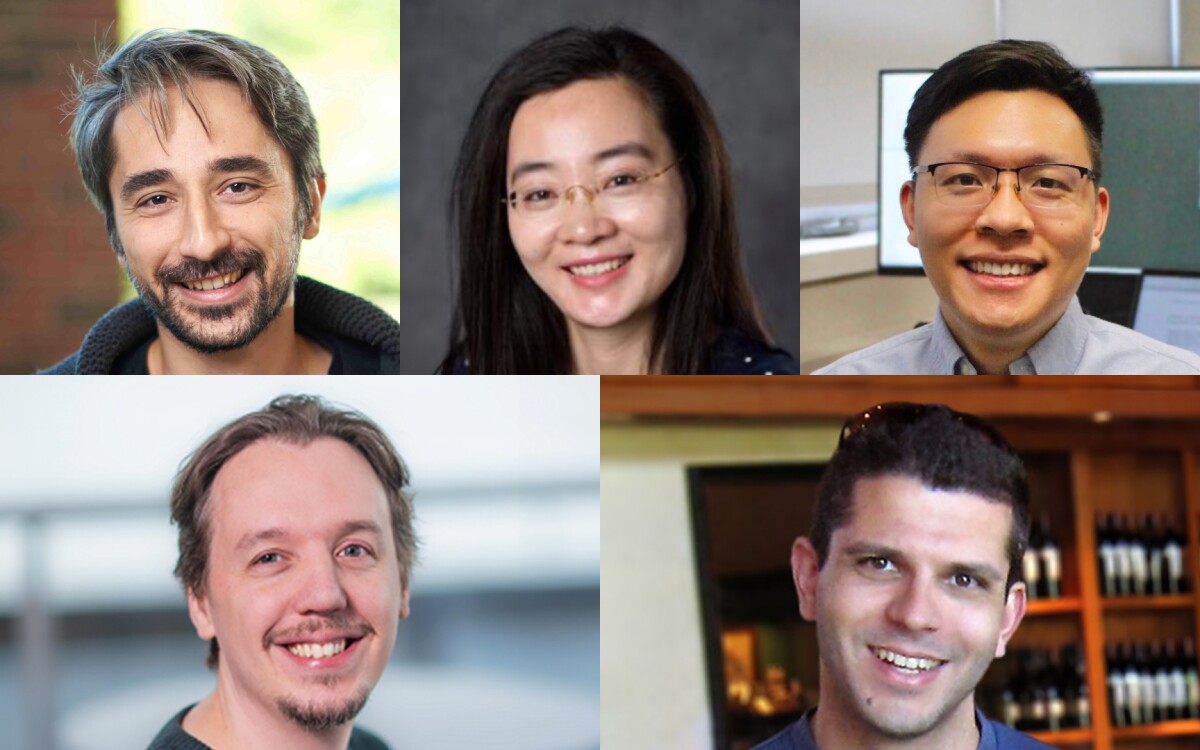
Recipient | University | Research title |
Murat Kocaoglu | Purdue University | Causal Anomaly Detection from Non-stationary Time-series in the Cloud |
Hui Liu | Michigan State University | Harnessing the Power of Weakly-Supervised Graph Representation Learning for Cybersecurity |
Xiaorui Liu | North Carolina State University | Harnessing the Power of Weakly-Supervised Graph Representation Learning for Cybersecurity |
Thomas Pasquier | University of British Columbia | Building Robust Provenance-based Intrusion Detection |
Michalis Polychronakis | Stony Brook University | SafeTrans: AI-assisted Transcompilation to Memory-safe Languages |
Automated Reasoning
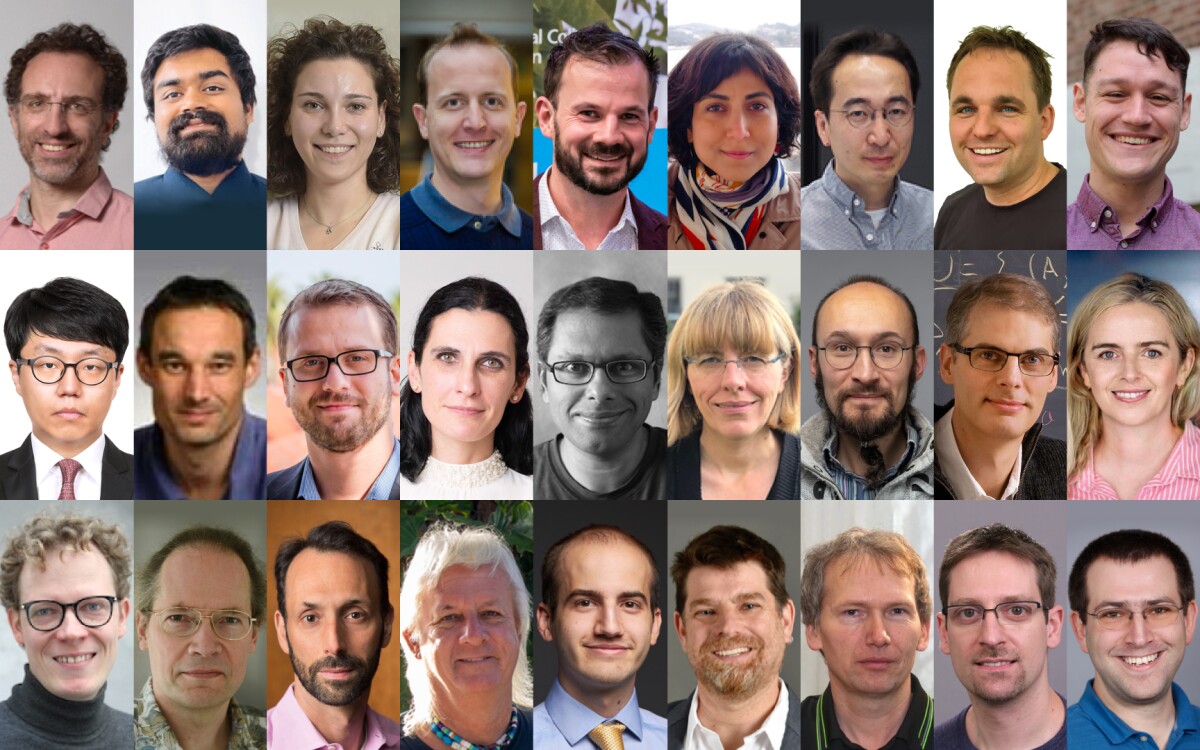
Recipient | University | Research title |
Armin Biere | University of Freiburg | From Mavericks to Teamplayers: Fostering Solver Cooperation in Distributed SAT Solving |
Victor Braberman | Universidad de Buenos Aires | Abstractions for Validating Distributed Protocol Reference Implementations |
Varun Chandrasekaran | University of Illinois Urbana-Champaign | Automating Privacy Compliance |
Maria Christakis | TU Wien | Testing Dafny for Unsoundness and Brittleness Bugs |
Werner Dietl | University of Waterloo | Optional Type Systems for Model-Implementation Consistency |
Alastair Donaldson | Imperial College London | Validating Compilers for the Dafny Verified Programming Language |
Azadeh Farzan | University of Toronto | Better Predictability in Dynamic Data Race Detection |
Sicun Gao | University Of California, San Diego | Proof Optimization and Generalization in dReal |
Tobias Grosser | University Of Cambridge | Correct and High-Performance Domain-Specific Compilation with Lean and MLIR |
Andrew Head | University Of Pennsylvania | TYCHE: An IDE for Property-Based Testing |
Kihong Heo | Korea Advanced Institute Of Science and Technology - KAIST | Generative Translation Validation for JIT Compiler in the V8 JavaScript Engine |
Frans Kaashoek | Massachusetts Institute of Technology | Flotilla: Compositional Formal Verification of Liveness of Distributed Systems Implementations |
Baris Kasikci | University of Washington - Seattle | Privacy-Conscious Failure Reproduction for Root Cause Diagnosis in Large-Scale Distributed Systems |
Laura Kovacs | TU Wien | QuAT: Quantifiers with Arithmetic Theories are Friends with Benefits |
Shriram Krishnamurthi | Brown University | Paralegal: Scalable Tooling to Find Privacy Bugs in Application Code |
Corina Pasareanu | Carnegie Mellon University | Proving the Absence of Timing Side Channels in Cryptographic Applications |
Jean Pichon-Pharabod | Aarhus University | Validating Isolation of Virtual Machines in the Cloud |
Benjamin Pierce | University Of Pennsylvania | TYCHE: An IDE for Property-Based Testing |
Ruzica Piskac | Yale University | Democratizing the Law - Using LLMs and Automated Reasoning for Legal Reasoning |
Malte Schwarzkopf | Brown University | Paralegal: Scalable Tooling to Find Privacy Bugs in Application Code |
Peter Sewell | University Of Cambridge | The Foundations of Cloud Virtual-machine Isolation |
Scott Shapiro | Yale University | Democratizing the Law - Using LLMs and Automated Reasoning for Legal Reasoning |
Geoffrey Sutcliffe | University Of Miami | Automated Theorem Proving Community Infrastructure in the AWS Cloud |
Joseph Tassarotti | New York University | Asynchronous Couplings for Probabilistic Relational Reasoning in Dafny |
Sebastian Uchitel | Universidad de Buenos Aires | Abstractions for Validating Distributed Protocol Reference Implementations |
Josef Urban | Czech Technical University | Learning Based Synthesis Meets Learning Guided Reasoning |
Thomas Wies | New York University | Automating Privacy Compliance |
Nickolai Zeldovich | Massachusetts Institute of Technology | Flotilla: Compositional Formal Verification of Liveness of Distributed Systems Implementations |
AWS AI
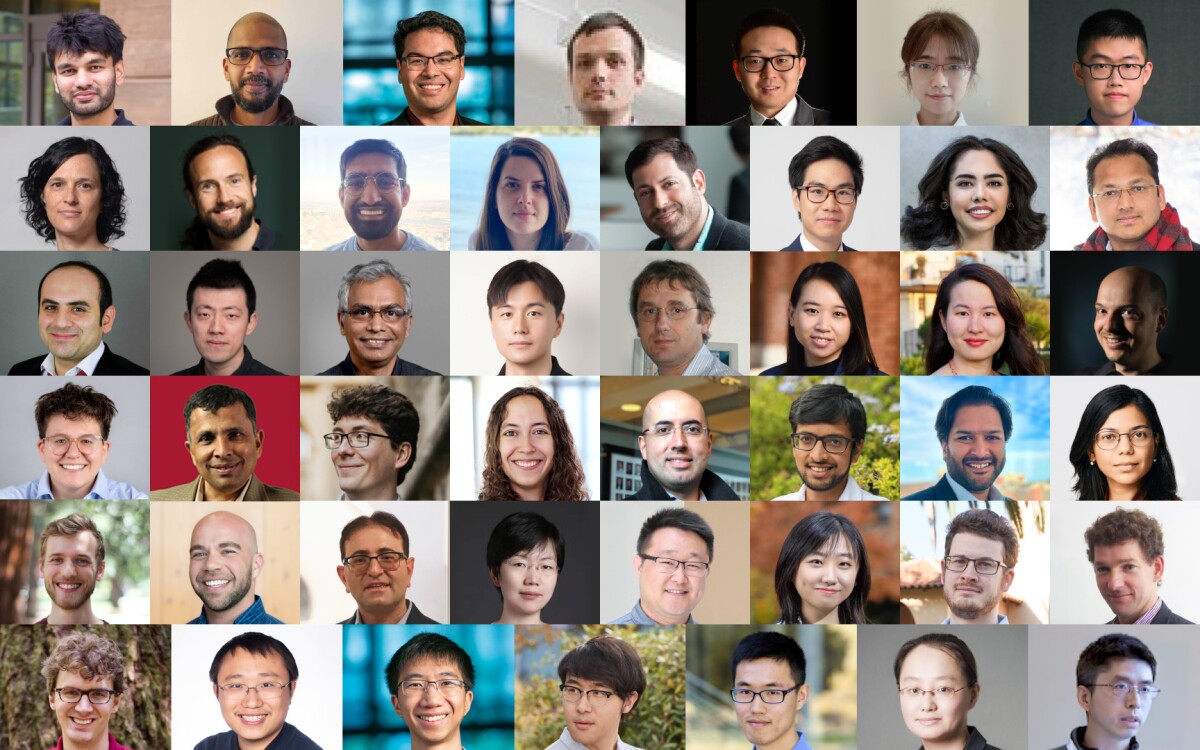
Recipient | University | Research title |
Pulkit Agrawal | Massachusetts Institute Of Technology | Adapting Foundation Models without Finetuning |
Niranjan Balasubramanian | Stony Brook University | An API Sandbox for Complex Tasks on Common Applications |
Osbert Bastani | University Of Pennsylvania | Uncertainty Quantification for Trustworthy Language Generation |
Matei Ciocarlie | Columbia University | Do You Speak EMG? Generative Pre-training on Electromyographic Signals for Controlling a Rehabilitation Robot after Stroke |
Caiwen Ding | University of Connecticut | Graph of Thought: Boosting Logical Reasoning in Large Language Models |
Yufei Ding | University Of California, San Diego | A Hollistic Compiler and Runtime System for Efficient and Scalable LLM Serving |
Xinya Du | University Of Texas At Dallas | Process-guided Fine-tuning for Answering Complex Questions |
Luciana Ferrer | University of Buenos Aires - CONICET | Efficient Adaptation of Generative Language Models through Unsupervised Calibration |
Jakob Foerster | University Of Oxford | Compute-only Scaling of Large Language Models |
Nikhil Garg | Cornell University | Recommendation systems in high-stakes settings |
Georgia Gkioxari | California Institute Of Technology | Towards a 3D Foundation Model: Recognize and Reconstruct Anything |
Tom Goldstein | University of Maryland | Building Safer Diffusion Models |
Aditya Grover | University of California, Los Angeles | Personalizing Multimodal Generative Models via In-Context Preference Modeling |
Albert Gu | Carnegie Mellon University | Scaling the Next Generation of Foundation Model Architectures |
Mahdi S. Hosseini | Concordia University | Toward Auto-Populating Synoptic Reports in Diagnostic Pathology |
Maliheh Izadi | Delft University Of Technology | Understanding and Regulating Memorization in Large Language Models for Code |
Vijay Janapa Reddi | Harvard University | Benchmarking the Safety of Generative AI Models with Data-centric AI Challenges |
Adel Javanmard | University of Southern California | Reliable AI for Generation of Medical Reports from MRI Scans |
Jianbo Jiao | University Of Birmingham | PCo3D: Physically Plausible Controllable 3D Generative Models |
Subbarao Kambhampati | Arizona State University | Understanding and Leveraging Planning, Reasoning & Self-Critiquing Capabilities of Large Language Models |
Kangwook Lee | University Of Wisconsin–Madison | Information and Coding Theory-Based Framework for Prompt Engineering |
Ales Leonardis | University Of Birmingham | PCo3D: Physically Plausible Controllable 3D Generative Models |
Anqi Liu | Johns Hopkins University | (Multi-)Calibrated Active Learning under Subpopulation Shift |
Lydia Liu | Princeton University | From Predictions to Positive Impact: Foundations of Responsible AI in Social Systems |
Song Mei | University Of California, Berkeley | Mathematical Foundations and Physical Principles of Foundation Models and Generative AI |
Pablo Piantanida | National Centre for Scientific Research (CNRS) | Efficient Adaptation of Generative Language Models through Unsupervised Calibration |
Chara Podimata | Massachusetts Institute Of Technology | Responsible AI through User Incentive-Awareness |
Bhiksha Raj | Carnegie Mellon University | Text and Speech Large Language Models |
Christian Rupprecht | University Of Oxford | Viewset Diffusion for Probabilistic 3D Reconstruction |
Olga Russakovsky | Princeton University | Diffusion models: Generative models beyond data generation |
Vatsal Sharan | University Of Southern California | Debiasing ML-based Decision Making using Multicalibration |
Abhinav Shrivastava | University Of Maryland | Audio-conditioned Diffusion Models for Generating Lip-synchronized Videos |
Rachee Singh | Cornell University | Accelerating collective communication for distributed ML |
Vincent Sitzmann | Massachusetts Institute Of Technology | 2D and 3D Animation via Image-Conditional Generative Flow Models |
Justin Solomon | Massachusetts Institute Of Technology | Lightweight Algorithms for Generative AI |
Mahdi Soltanolkotabi | University of Southern California | Reliable AI for Generation of Medical Reports from MRI Scans |
Qian Tao | Delft University of Technology | Φ-Generative Medical Imaging by Physics and AI (PhAI) |
Yapeng Tian | University Of Texas At Dallas | Integrating Visual Alignment and Text Interaction for Multi-modal Audio Content Generation |
Sherry Tongshuang Wu | Carnegie Mellon University | Generating Deployable Models from Natural Language Instructions through Adaptive Data Curation |
Florian Tramer | Eth Zurich | Can Technology Protect us from Generative AI? |
Arie van Deursen | Delft University Of Technology | Understanding and Regulating Memorization in Large Language Models for Code |
Andrea Vedaldi | University Of Oxford | Viewset Diffusion for Probabilistic 3D Reconstruction |
Carl Vondrick | Columbia University | Viper: Visual Inference via Python Execution for Reasoning |
Xiaolong Wang | University of California, San Diego | Generating Compositional 3D Scenes and Embodied Tasks with Large Language Models |
Eric Wong | University Of Pennsylvania | Adversarial Manipulation of Prompting Interfaces |
Saining Xie | New York University | Image Sculpting: Precise Image Generation and Editing with Interactive Geometry Control |
Rex Ying | Yale University | Diff-H: Hyperbolic Text-to-Image Diffusion Generative Model |
Minlan Yu | Harvard University | Troubleshooting Distributed Training Systems |
Zhiru Zhang | Cornell University | A Unified Approach to Tensor Graph Optimization |
AWS Cryptography and Privacy
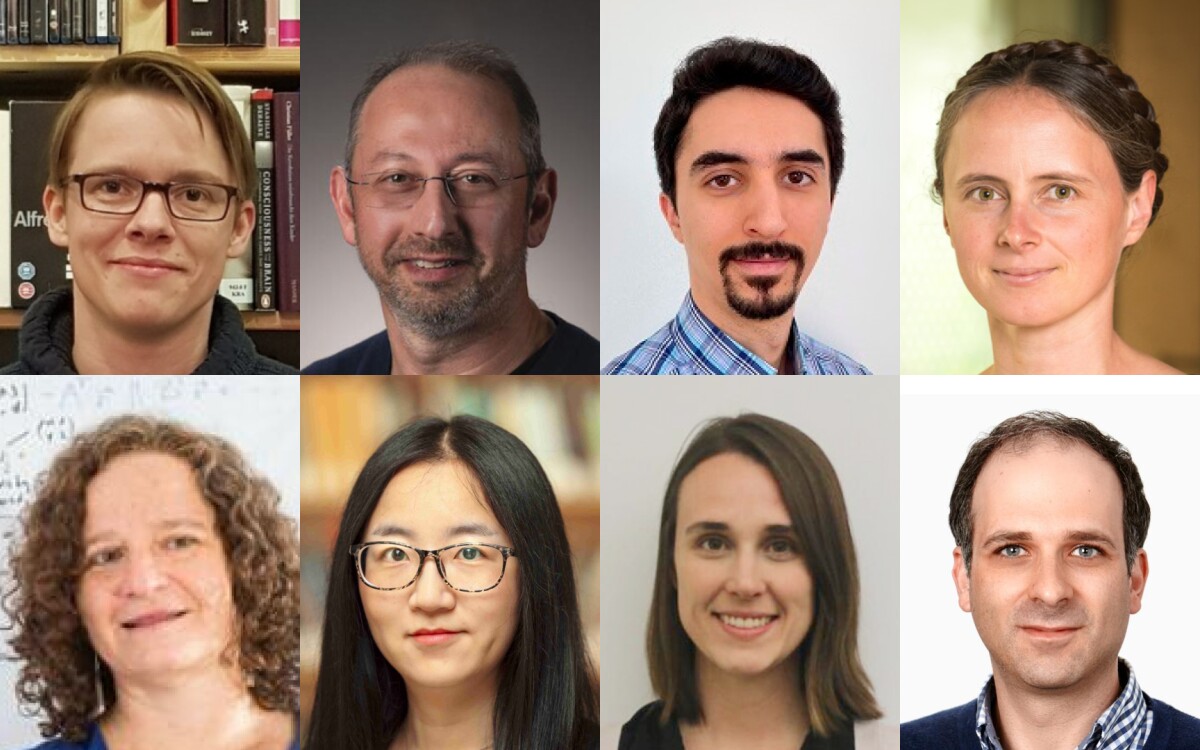
Recipient | University | Research title |
Christopher Brzuska | Aalto University | Secure Messaging: Updates Efficiency & Verification |
Tevfik Bultan | University of California, Santa Barbara | Detecting and Quantifying Information Leakages in Crypto Libraries |
Muhammed Esgin | Monash University | Practical Post-Quantum Oblivious Pseudorandom Functions Supporting Verifiability |
Nadia Heninger | University of California, San Diego | Bringing Modern Security Guarantees to End-to-End Encrypted Cloud Storage |
Tal Malkin | Columbia University | Cryptographic Techniques for Machine Learning |
Peihan Miao | Brown University | Advancing Private Set Intersection for Wider Industrial Adoption |
Virginia Smith | Carnegie Mellon University | Rethinking Watermark Embedding and Detection for LLMs |
Ron Steinfeld | Monash University | Practical Post-Quantum Oblivious Pseudorandom Functions Supporting Verifiability |
AWS Database Services
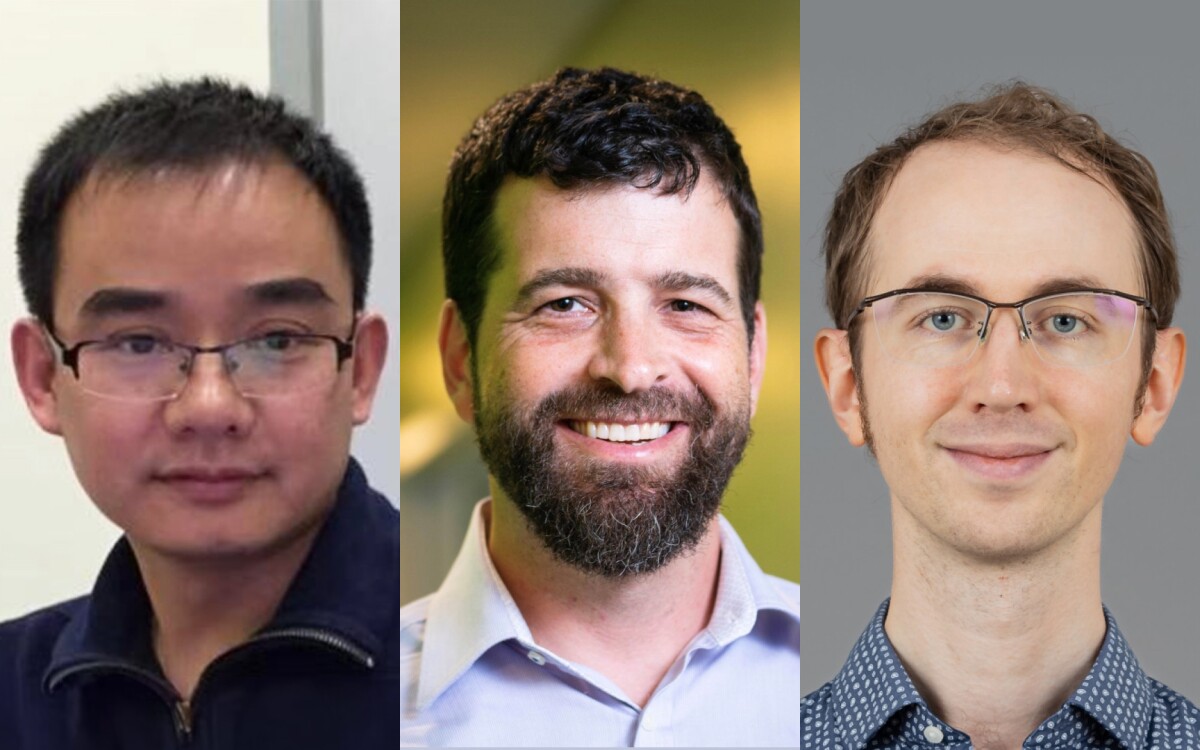
Recipient | University | Research title |
Lei Cao | University Of Arizona | SEED: Simple, Efficient, and Effective Data Management via Large Language Models |
Natacha Crooks | University Of California, Berkeley | Mammoths Are Slow: The Overlooked Transactions of Graph Data |
Samuel Madden | Massachusetts Institute Of Technology | SEED: Simple, Efficient, and Effective Data Management via Large Language Models |
Manuel Rigger | National University Of Singapore | Democratizing Database Fuzzing |
Georgia Institute Of Technology | Dynamic Data Layout Optimization with Worst-case Guarantees |
Sustainability
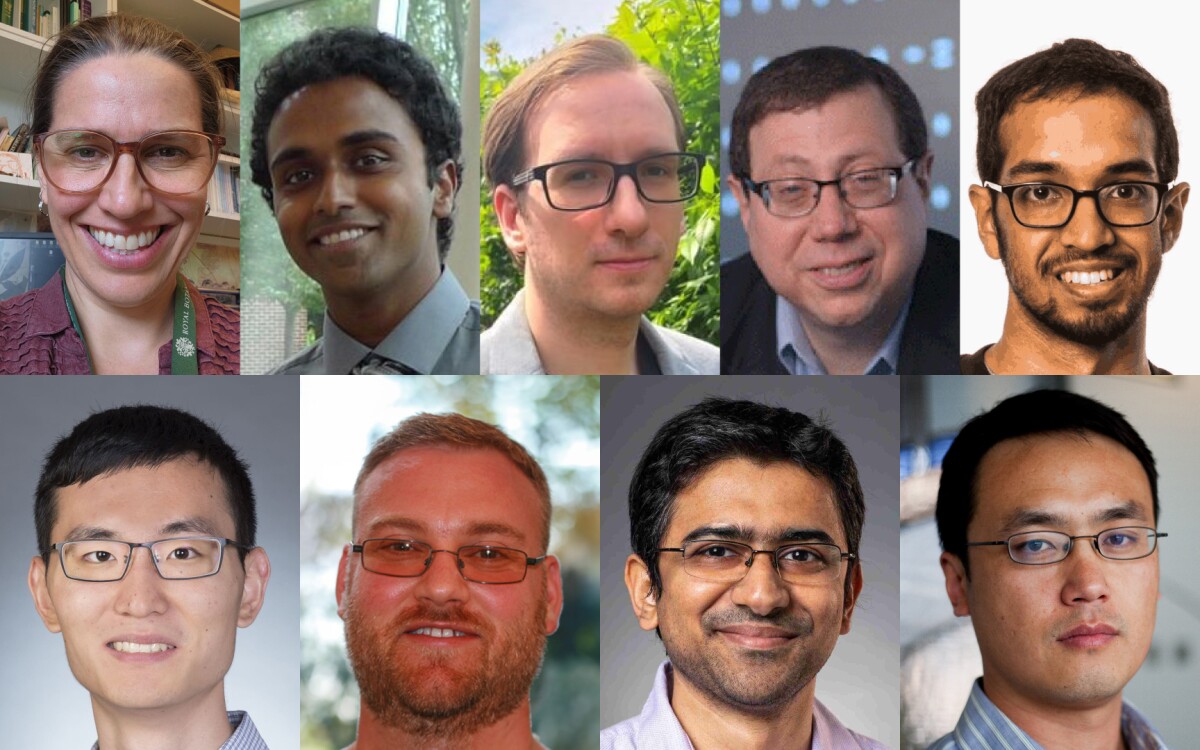
Recipient | University | Research title |
Kate Armstrong | New York Botanical Garden | VERDEX: remote sensing of plant biodiversity |
Praveen Bollini | University Of Houston | Data-driven design and optimization of selective nanoporous catalysts for biofuel conversion |
Brandon Bukowski | Johns Hopkins University | Data-driven design and optimization of selective nanoporous catalysts for biofuel conversion |
Alan Edelman | Massachusetts Institute of Technology | Scientific Machine Learning with Application to Probabilistic Climate Forecasting and Sustainability |
Kosa Goucher-Lambert | University of California, Berkeley | LCAssist: An Interactive System for Life-Cycle-Informed Sustainable Design Decision-Making |
Vikram Iyer | University of Washington - Seattle | Data-Driven Sustainable Polymer Design for Circuits, Packaging, and Actuators |
Can Li | Purdue University | Design and Analysis of Sustainable Supply Chains Using Optimization and Large Language Models |
Damon Little | New York Botanical Garden | VERDEX: remote sensing of plant biodiversity |
Aniruddh Vashisth | University of Washington - Seattle | Data-Driven Sustainable Polymer Design for Circuits, Packaging, and Actuators |
Ming Xu | Tsinghua University | Advancing Sustainable Practices in the AI Era: Integrating Large Language Models for Automated Life Cycle Assessment Modeling |