In 2017, when the journal IEEE Internet Computing was celebrating its 20th anniversary, its editorial board decided to identify the single paper from its publication history that had best withstood the “test of time”. The honor went to a 2003 paper called “Amazon.com Recommendations: Item-to-Item Collaborative Filtering”, by then Amazon researchers Greg Linden, Brent Smith, and Jeremy York.
Collaborative filtering is the most common way to do product recommendation online. It’s “collaborative” because it predicts a given customer’s preferences on the basis of other customers’.
“There was already a lot of interest and work in it,” says Smith, now the leader of Amazon’s Weblab, which does A/B testing (structured testing of variant offerings) at scale to enable data-driven business decisions. “The world was focused on user-based collaborative filtering. A user comes to the website: What other users are like them? We sort of turned it on its head and found a different way of doing it that had a lot better scaling and quality characteristics for online recommendations.”
The better way was to base product recommendations not on similarities between customers but on correlations between products. With user-based collaborative filtering, a visitor to Amazon.com would be matched with other customers who had similar purchase histories, and those purchase histories would suggest recommendations for the visitor.
With item-to-item collaborative filtering, on the other hand, the recommendation algorithm would review the visitor’s recent purchase history and, for each purchase, pull up a list of related items. Items that showed up repeatedly across all the lists were candidates for recommendation to the visitor. But those candidates were given greater or lesser weight depending on how related they were to the visitor's prior purchases.
That notion of relatedness is still derived from customers’ purchase histories: item B is related to item A if customers who buy A are unusually likely to buy B as well. But Amazon’s Personalization team found, empirically, that analyzing purchase histories at the item level yielded better recommendations than analyzing them at the customer level.
Family ties
Beyond improving recommendations, item-to-item collaborative filtering also offered significant computational advantages. Finding the group of customers whose purchase histories most closely resemble a given visitor’s would require comparing purchase histories across Amazon’s entire customer database. That would be prohibitively time consuming during a single site visit.
The alternatives are either to randomly sample other customers in real time and settle for the best matches found or to build a huge offline similarity index by comparing every customer to every other. Because Amazon customers’ purchase histories can change dramatically in the course of a single day, that index would have to be updated regularly. Even offline indexing presents a huge computational burden.
On average, however, a given product sold on the Amazom Store purchased by only a tiny subset of the site’s customers. That means that inspecting the recent-purchase histories of everyone who bought a given item requires far fewer lookups than identifying the customers who most resemble a given site visitor. Smith and his colleagues found that even with early-2000s technology, it was computationally feasible to produce an updated list of related items for every product on the Amazon site on a daily basis.
The crucial question: how to measure relatedness. Simply counting how often purchasers of item A also bought item B wouldn’t do; that would make a few bestsellers like Harry Potter books and trash bags the top recommendations for every customer on every purchase.
Instead, the Amazon researchers used a relatedness metric based on differential probabilities: item B is related to item A if purchasers of A are more likely to buy B than the average Amazon customer is. The greater the difference in probability, the greater the items’ relatedness.
When Linden, Smith, and York published their paper in IEEE Internet Computing, their item-based recommendation algorithm had already been in use for six years. But it took several more years to identify and correct a fundamental flaw in the relatedness measure.
Getting the math right
The problem: the algorithm was systematically underestimating the baseline likelihood that someone who bought A would also buy B. Since a customer who buys a lot of products is more likely to buy A than a customer who buys few products, A buyers are, on average, heavier buyers than the typical Amazon customer. But because they’re heavy buyers, they’re also unusually likely to buy B.
Smith and his colleagues realized that it wasn’t enough to assess the increased likelihood of buying product B given the purchase of product A; they had to assess the increased likelihood of buying product B with any given purchase. That is, they discounted heavy buyers’ increased likelihood of buying B according to the heaviness of their buying.
“That was a large improvement to recommendations quality, when we got the math right,” Smith says.
That was more than a decade ago. Since then, Amazon researchers have been investigating a wide variety of ways to make customer recommendations more useful: moving beyond collaborative filtering to factor in personal preferences such as brands or fashion styles; learning to time recommendations (you may want to order more diapers!); and learning to target recommendations to different users of the same account, among many other things.
In June 2019, during a keynote address at Amazon’s first re:MARS conference, Jeff Wilke, then the CEO of Amazon’s consumer division, highlighted one particular advance, in the algorithm for recommending movies to Amazon’s Prime Video customers. Amazon researchers’ innovations led to a twofold improvement in that algorithm’s performance, which Wilke described as a “once-in-a-decade leap”.
Entering the matrix
Recommendation is often modeled as a matrix completion problem. Imagine a huge grid, whose rows represent Prime Video customers and whose columns represent the movies in the Prime Video catalogue. If a customer has seen a particular movie, the corresponding cell in the grid contains a one; if not, it’s blank. The goal of matrix completion is to fill in the grid with the probabilities that any given customer will watch any given movie.
In 2014, Vijai Mohan’s team in the Personalization group — Avishkar Misra, Jane You, Rejith Joseph, Scott Le Grand, and Eric Nalisnick — was asked to design a new recommendation algorithm for Prime Video. At the time, the standard technique for generating personalized recommendations was matrix factorization, which identifies relatively small matrices that, multiplied together, will approximate a much larger matrix.
Inspired by work done by Ruslan Salakhutdinov — then an assistant professor of computer science at the University of Toronto — Mohan’s team instead decided to apply deep neural networks to the problem of matrix completion.
The typical deep neural network contains thousands or even millions of simple processing nodes, arranged into layers. Data is fed into the nodes of the bottom layer, which process it and pass their results to the next layer, and so on; the output of the top layer represents the result of some computation.
Training the network consists of feeding it lots of sample inputs and outputs. During training, the network’s settings are constantly adjusted, until they minimize the average discrepancy between the top layer’s output and the target outputs in the training examples.
Reconstruction
Matrix completion methods commonly use a type of neural network called an autoencoder. The autoencoder is trained simply to output the same data it takes as input. But in-between the input and output layers is a bottleneck, a layer with relatively few nodes — in this case, only 100, versus tens of thousands of input and output nodes.
We had to go and doublecheck and re-run the experiments multiple times, I was giving a hard time to the scientists. I was saying, ‘You probably made a mistake.’
As a consequence, the network can’t just copy inputs directly to outputs; it must learn a general procedure for compressing and then re-expanding every example in the training set. The re-expansion will be imperfect: in the movie recommendation setting, the network will guess that customers have seen movies they haven’t. But when, for a given customer-movie pair, it guesses wrong with high confidence, that’s a good sign that the customer would be interested in that movie.
To benchmark the autoencoder’s performance, the researchers compared it to two baseline systems. One was the latest version of Smith and his colleagues’ collaborative-filtering algorithm. The other was a simple listing of the most popular movie rentals of the previous two weeks. “In the recommendations world, there’s a cardinal rule,” Mohan says. “If I know nothing about you, then the best things to recommend to you are the most popular things in the world.”
To their mild surprise, the item-to-item collaborative-filtering algorithm outperformed the autoencoder. But to their much greater surprise, so did the simple bestseller list. The autoencoder’s performance was “so bad that we had to go and doublecheck and re-run the experiments multiple times,” Mohan says. “I was giving a hard time to the scientists. I was saying, ‘You probably made a mistake.’”
Once they were sure the results were valid, however, they were quick to see why. In a vacuum, matrix completion may give the best overview of a particular customer’s tastes. But at any given time, most movie watchers will probably opt for recent releases over neglected classics in their preferred genres.
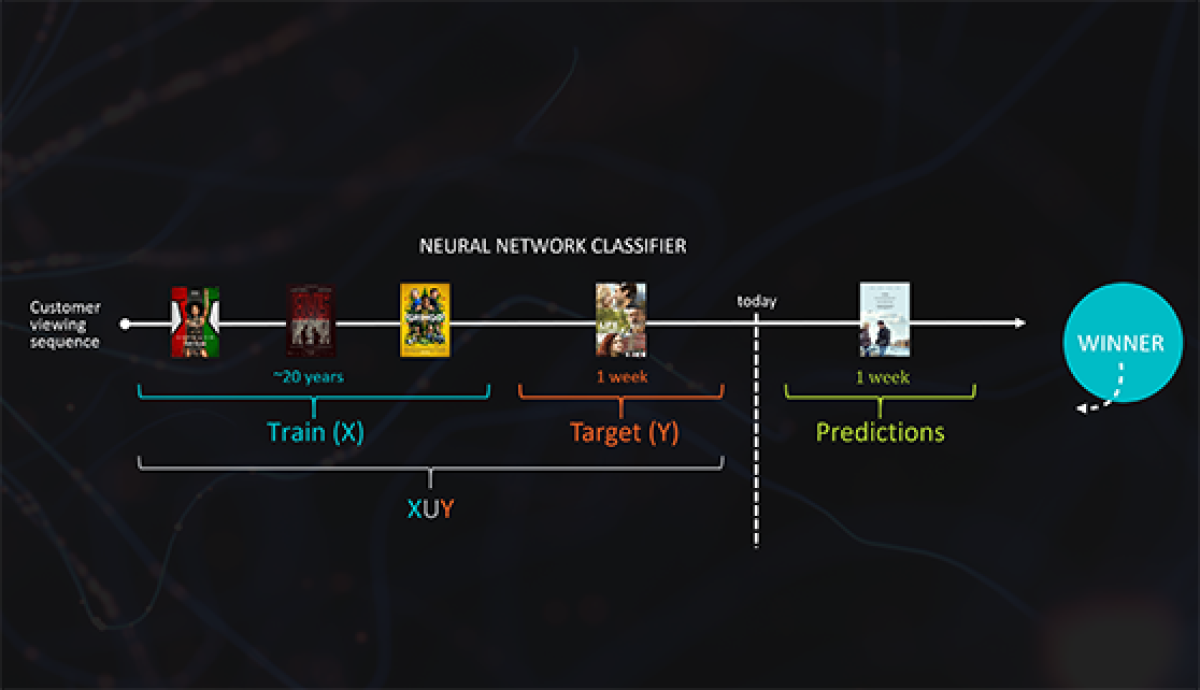
So Mohan’s team re-framed the problem. They still used an autoencoder, but they trained it on movie-viewing data that had been sorted chronologically. During training, the autoencoder saw data on movies that customers had watched before some cutoff time. But it was evaluated on how well it predicted the movies they had watched in the two-week period after the cutoff time.
Because Prime Video’s Web interface displays six movie recommendations on the page associated with each title in its catalogue, the researchers evaluated their system on whether at least one of its top six recommendations for a given customer was in fact a movie that that customer watched in the two-week period after the cutoff date. By that measure, not only did the autoencoder outperform the bestseller list, but it also outperformed item-to-item collaborative filtering, two to one. As Wilke put it at re:MARS, “We had a winner.”
Whether any of the work that Amazon researchers are doing now will win test-of-time awards two decades hence remains to be seen. But Smith, Mohan, and their colleagues will continue to pursue new approaches to designing recommendation algorithms, in the hope of making Amazon.com that much more useful for customers.