For every order placed on the Amazon Store, mathematical models developed by Amazon’s Supply Chain Optimization Technologies organization (SCOT) work behind the scenes to ensure that product inventories are best positioned to fulfill the order.
Forecasting models developed by SCOT predict the demand for every product. Buying systems determine the right level of product to purchase from different suppliers, while large-scale placement systems determine the optimal location for products across the hundreds of facilities belonging to Amazon’s global fulfillment network.
“With hundreds of millions of products sold across multiple geographies, developing automated models to make inventory planning decisions at Amazon scale is one of the most challenging and rewarding parts of our work,” said Deepak Bhatia, vice president of Supply Chain Optimization Technologies at Amazon.
We made the decision to redesign Amazon’s supply chain systems from the ground up.
In the first half of the past decade, Amazon transitioned from a largely manual supply chain management system to an automated one. However, when faced with the need to evolve Amazon’s supply chain to meet customer needs, and the introduction of same day delivery services like Prime Now, the team moved to replace that system with a new one that would better help Amazon fulfill delivery promises made to customers.
“As far back as 2016, we were able to see that the automated system we had at the time wouldn’t help us meet the ever-growing expectations of our customers,” Bhatia recalled. “As a result, we made the decision to redesign Amazon’s supply chain systems from the ground up.”
A global company catering to local needs
“In 2016, Amazon’s supply chain network was designed for scenarios where inventory from any fulfillment center could be shipped to any customer to meet a two-day promise,” said Salal Humair, senior principal research scientist at Amazon who has been with the company for seven years.
This design was inadequate for the new world in which Amazon was operating; one shaped by what Humair calls the “globalization-localization imperative.” Amazon’s expansion included an increasing number of international locations — at the time, the company had 175 fulfillment centers serving customers in 185 countries around the world.
“Meeting the needs of our customer base meant that we needed to serve those customers in multiple geographies,” Humair said.
As Amazon continued to expand internationally, the company also launched one-day and same day delivery windows in local regions for services like Amazon Prime and Amazon Prime Now.
“We quickly realized that in addition to serving customers around the globe, we also had to pivot from functioning as a national network to a local one, where we could position inventory close to our customers,” Humair says.
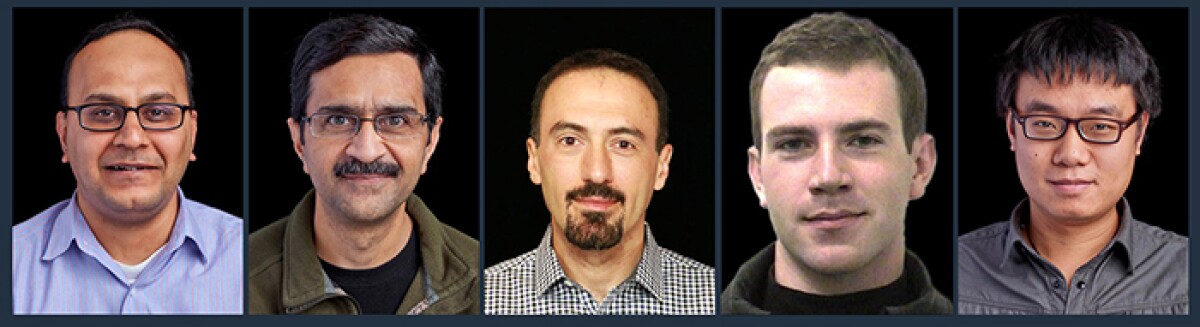
In addition to the ‘globalization-localization imperative,’ the growing complexity of Amazon’s supply chain network further complicated matters. To meet the increased customer demand for a diverse variety of shipping speeds, Amazon’s fulfillment network was expanding to include an increasing number of building types and sizes: from fulfillment centers (for everyday products) and non-sortable fulfillment centers (for larger items), to smaller fulfillment centers catering to same-day orders, and distribution centers that supplied products to downstream fulfillment centers. The network was increasingly becoming layered, and fulfillment centers in one layer (or echelon) were acting as suppliers to other layers.
“We had to reimagine every aspect of our system to account for this increasing number of echelons,” Humair said.
The science behind multi-echelon inventory planning
The sheer scale of Amazons operations posed a significant challenge from a scientific perspective. Amazon Store orders are fulfilled through complex dynamic optimization processes — where a real-time order assignment system can choose to fulfill an order from the optimal fulfillment center that can meet the customer promise. This real-time order assignment makes inventory planning an incredibly complex problem to solve.
Other inventory-related dependencies further complicate matters: the same pool of inventory is frequently used to serve demand for orders with different shipping speeds. Consider a box of diapers: it can be used to fulfill an order for a two-day Prime delivery. It can also be used to ease the life of harried parents who have placed an order on Prime Now, and need diapers for their baby delivered in a two-hour window.
Amazon’s scientists also have to contend with a high degree of uncertainty. Customer demand for products cannot be perfectly predicted even with the most advanced machine learning models. In addition, lead times from vendors are subject to natural variation due to manufacturing capacity, transportation times, weather, etc., adding another layer of uncertainty.
This required building a custom solution, one that relies on sound scientific principles and rigor, and borrowing ideas from academic literature as building blocks, but with ground-breaking in-house invention.
Humair notes that the scale of Amazon’s operations, the complexity of the network, and the uncertainties associated with the company’s dynamic ordering system make it impossible to even write down a closed-form objective function for the optimization problem the team was trying to solve.
While multi-echelon inventory optimization is a well-researched field, the bulk of literature focused on single-product models, proposed solutions for much simpler networks, or used greatly simplified assumptions for replenishing inventory.
“There is a large body of academic literature on multi-echelon inventory management, and papers typically focus on one or two main aspects of the problem,” noted Alp Muharremoglu, a senior principal scientist in SCOT who spent 15 years as a faculty member at Columbia University and the University of Texas at Dallas. “Amazon’s scale and complexity meant no existing solution was a perfect fit. This required building a custom solution, one that relies on sound scientific principles and rigor, and borrowing ideas from academic literature as building blocks, but with ground-breaking in-house invention to push the boundaries of academic research. It is a thrill to see multi-echelon inventory theory truly in action in such a large scale and dynamic supply chain.”
As a result, the system developed by SCOT (a project whose roots stretch back to 2016) is a significant break from the past. The heart of the model is a multi-product, multi-fulfillment center, capacity-constrained model for optimizing inventory levels for multiple delivery speeds, under a dynamic fulfillment policy. The framework then uses a Lagrangian-type decomposition framework to control and optimize inventory levels across Amazon’s network in near real-time.
Broadly speaking, decomposition is a mathematical technique that breaks a large, complex problem up into smaller and simpler ones. Each of these problems is then solved in parallel or sequentially. The Lagrangian method of decomposition factors complicated constraints into the solution, while providing a ‘cost’ for violating these constraints. This cost makes the problem easier to solve by providing an upper bound to the maximization problem, which is critical when planning for inventory levels at Amazon’s scale.
“We computed opportunity costs for storage and flows at every fulfillment center,” Humair said. “Using Lagrangean decomposition, we then used these costs to calculate the related inventory positions at these locations. Crucially, we incorporated a stochastic dynamic fulfillment policy in a scalable optimization model, allowing Amazon to calculate inventory levels not at just one location, but at every layer in our fulfillment network.”
Mobilizing the organization
While creating the new multi-echelon system was an imposing scientific challenge, it also represented a significant organizational accomplishment, one that required collaboration across multiple teams.
“Moving multi-echelon from concept to implementation was one of the most difficult organizational challenges we’ve worked through; we had many potential implementations that looked radically different in terms of model capabilities, interfaces, engineering challenges, and long-term implications for how our teams would interact with each other,” said Jeff Maurer, a SCOT vice president who has been instrumental in rolling out the automation of Amazon’s supply chain and oversaw the roll out of the multi-echelon system.
“This was also a case where there wasn’t a great way to decide between them without building and exploring one or more approaches in production. Ultimately, that’s what we did — we picked the best options we could identify, built them out, learned from them, then repeated that process. We learned things by experimenting with real production implementations that we could never have learned from simplified models or simulations alone, given the complexity of the real-world dynamics of our supply chain. But it was hard on the teams — it wasn’t always obvious that the systems the teams were iterating on were the best path, given the high directional ambiguity.”
“Sometimes, the only way to make a massive change is to realize that you have no option but to make that change,” said Yan Xia, principal applied scientist at Amazon. Humair noted that Xia played “a pivotal role” over the four years it took the company to migrate to the new multi-echelon system.
Xia recalled that teams within SCOT were keenly aware of the limitations of the existing system. However, there was skepticism that the multi-echelon system was the right solution.
“The skepticism was understandable,” Xia said. “It’s one thing to have a big idea. But you also have to be able to present the benefits of your idea in a coherent way.”
Xia gave an example of how he helped convince members from the buying and placement teams about the benefits of the new model.
“One team decides optimal suppliers to source products from, while another team makes decisions on where these products should be placed,” Xia explained. “I was able to show them how the two functions would essentially be unified in the multi-echelon system. Sure, it would change how they worked on a day-to-day basis — but it would do so in a way that made their lives simpler.”
To help ensure that resources were made available for the development of the multi-echelon system, Xia also focused on driving alignment among leaders in SCOT. He developed a simulation based on real-world data. The results clearly demonstrated that the proposed solution for inventory forecasting, buying, and placement would result in a steep decline in shipping costs, which in turn would allow Amazon to keep prices lower for customers.
Teams involved in multi-echelon planning discussions were galvanized after seeing the results of the simulation.
“Everyone bought into the vision,” Xia said. “We began to collaborate in near real-time. If we ran into a problem, we didn’t wait around for a weekly sprint meeting. We just got together in a room, or stood next to a whiteboard and solved it.”
Xia said that this was also when things began to get more complex.
“An awareness of the complexity of the existing setup began to dawn on us,” says Xia. “We began to realize how every component in the system had multiple dependencies. For example, the buying platforms were tightly integrated with older legacy systems – we now had to factor these dependencies into our solutions.”
Solving a multi-item, multi-echelon with stochastic demand and lead-time and aggregated capacity constraints and differentiated customer service levels. That sort of thing is just unheard of in the academia and the industry.
The team iterated on the multi-echelon solution in a sequence of three in-production experiments (or labs) that spanned 2018 to 2020. The first lab incorporated components of the new system coupled with the old platform. It was a resounding success in terms of reducing costs, even while fulfilling orders associated with higher shipping speeds. The team moved on to testing the subsequent version of the multi-echelon system in the second lab.
“That wasn’t nearly as good,” Xia recalled. “Most things didn’t work as expected.”
However, the team was encouraged by leadership to keep going. This wasn’t SCOT’s first attempt at taking on big and ambitious projects. The organization had taken three years to deploy the first automated supply chain management system where they overcame various challenges.
“Sure, the failure of the second lab was demotivating,” Xia says. “But we knew from experience that this failure was only to be expected. It was part of the process.”
The team fixed the bugs, and moved on to testing new features in the third lab. These included critical system capabilities, such the ability to model order cut-off times for deliveries within a particular time window.
The system went live in 2020, and over the past year, the multi-echelon system has had a large and statistically significant impact in positioning products closer to customers.
“On a personal level, I am incredibly proud of our team. Having worked in the area of multi-echelon inventory optimization before I joined Amazon, I have a deep appreciation of how difficult it was,” Bhatia noted. “There is a strong sense of pride for the work the team is doing — such as solving a multi-item, multi-echelon with stochastic demand and lead-time and aggregated capacity constraints and differentiated customer service levels. That sort of thing is just unheard of in academia and industry. This is why I find it gratifying to work as a scientist and a leader at Amazon. It gives me a lot of pride, and none of this could have been achieved without the people and the culture we have.”