When a customer visits Amazon, there is an almost inherent expectation that the item they are searching for will be in stock. And that expectation is understandable — Amazon sells more than 400 million products in over 185 countries.
However, the sheer volume of products makes it cost-prohibitive to maintain surplus inventory levels for every product.
Historical patterns can be leveraged to make decisions on inventory levels for products with predictable consumption patterns — think household staples like laundry detergent or trash bags. However, most products exhibit a variability in demand due to factors that are beyond Amazon’s control.
Take the example of a book like Michelle Obama’s ‘Becoming’, or the recent proliferation of sweatsuits, which emerged as both comfortable and fashion-forward clothing option during 2020. It’s difficult to account for the steep spike in sales caused by a publicity tour featuring Oprah Winfrey, and nearly impossible to foresee the effect COVID-19 would have on, among other things, stay-at-home clothing trends.
Today, Amazon’s forecasting team has drawn on advances in fields like deep learning, image recognition and natural language processing to develop a forecasting model that makes accurate decisions across diverse product categories. Arriving at this unified forecasting model hasn’t been the result of one “eureka” moment. Rather, it has been a decade-plus long journey.
“When we started the forecasting team at Amazon, we had ten people and no scientists,” says Ping Xu, forecasting science director within Amazon’s Supply Chain Optimization Technologies (SCOT) organization. “Today, we have close to 200 people on our team. The focus on scientific and technological innovation has been key in allowing us to draw an accurate estimate of the immense variability in future demand, and make sure that customers are able to fulfill their shopping needs on Amazon.”
In the beginning: A patchwork of models
Kari Torkkola, senior principal research scientist, has played a key role in driving the evolution of Amazon’s forecasting systems in his 12 years at the company.
“When I joined Amazon, the company relied on traditional time series models for forecasting,” says Torkkola.
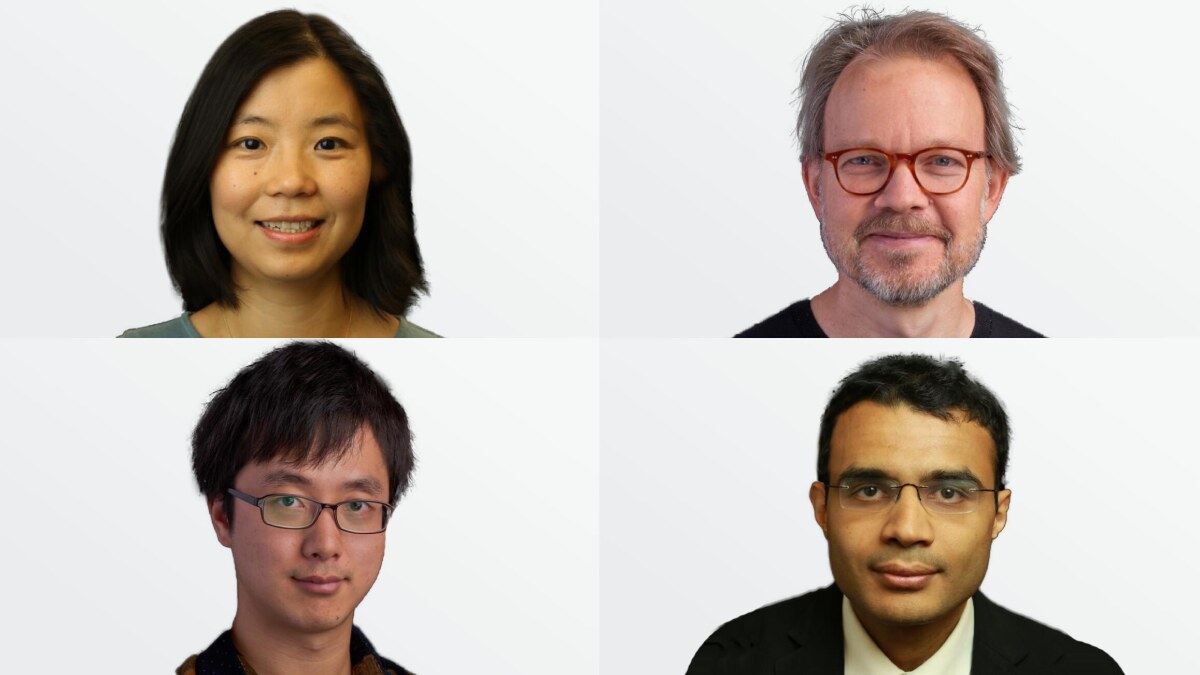
Time series forecasting is a statistical technique that uses historical values and associated patterns to predict future activity. In 2008, Amazon’s forecasting system used standard textbook time series forecasting methods to make predictions.
The system produced accurate forecasts in scenarios where the time series was predictable and stationary. However, it was unable to produce accurate forecasts for situations such as new products that had no prior history or products with highly seasonal sale patterns. Amazon’s forecasting teams had to develop new methods to account for each of these scenarios.
The system was incredibly hard to maintain. It gradually became clear that we needed to work towards developing a unified forecasting model.
So they set about developing an add-on component to model seasonal patterns in products such as winter jackets. Another specialized component solved for the effects of price elasticity, where products see spikes in demand due to price drops, while yet another component called Distribution Engine modeled past errors to produce estimates of forecast distributions on top of point forecasts.
“There were multiple components, all of which needed our attention,” says Torkkola. “The system was incredibly hard to maintain. It gradually became clear that we needed to work towards developing a unified forecasting model.”
Enter the random forest
If the number of components made maintaining the forecasting system laborious, routing special forecasting cases or even product groups to specialized models, which involved encoding expert knowledge — complicated matters even further.
Then Torkkola had a deceptively simple insight as he began working toward a unified forecasting model. “There are products across multiple categories that behave the same way,” he said.
For example, there is clear delineation between new products and products with an established history. The forecast for a new video game or laptop can be generated, in part, from how similar products behaved when they had launched in the past.
Torkkola extracted a set of features from information such as demand, sales, product category, and page views. He used these features to train a random forest model. Random forests are commonly used machine learning algorithms that comprise a number of decision trees. The outputs of the decision trees are then bundled together to provide a more stable and accurate prediction.
“By pooling everything together in one model, we gained statistical strength across multiple categories,” Torkkola says.
At the time, Amazon’s base forecasting system produced point forecasts to predict future demand — a single number that conveys information about the future demand. However, full forecast distributions or a set of quantiles of the distribution are necessary when it comes to make informed forecasting decisions on inventory levels. The Distribution Engine, which was another add-on to the base system, was producing poorly calibrated distributions.
Torkkola wrote an initial implementation of the random forest approach to output quantiles of forecast distributions. This was rewritten as a new incarnation called Sparse Quantile Random Forest (SQRF). That implementation allowed a single forecasting system to make forecasts for different product lines where each may have had different features present, thus each of those features seem very “sparse”. SQRF could also scale to millions of products, and represented a step change for Amazon to produce forecasts at scale.
However, the system suffered from a serious drawback. It still required the team to manually engineer features for the model — in other words, the system needed humans to define the input variables that would provide the best possible output.
That was all set to change in 2013, when the field of deep learning went into overdrive.
Deep learning produces the unified model
“In 2013, there was a lot of excitement in the machine learning community around deep learning,” Torkkola says. “There were significant advances in the field of image recognition. In addition, tensor frameworks such as THEANO developed by the University of Montreal were allowing developers to build deep learning models on the fly. Currently popular frameworks such as TensorFlow were not yet available.”
Neural networks held a tantalizing prospect for Amazon’s forecasting team. In theory, neural networks could do away with the need to manually engineer features. The network could ingest raw data and learn the most relevant implicit features needed to produce a forecast without human input.
With the help of interns hired over the summers of 2014 and 2015, Torkkola experimented with both feed forward and recurrent neural networks (RNNs). In feed forward networks, the connections between nodes do not form a cycle; the opposite is true with RNNs. The team began by developing a RNN to produce a point forecast. Over the next summer, another intern developed a model to produce a distribution forecast. However, these early iterations did not outperform SQRF, the existing production system.
Amazon’s forecasting team went back to the drawing board and had another insight, one that would prove crucial in the journey towards developing a unified forecasting model.
“We trained the network on minimizing quantile loss over multiple forecast horizons,” Torkkola says. Quantile loss is among the most important metrics used in forecasting systems. It is appropriate when under- and over-prediction errors have different costs, such as in inventory buying.
“When you train a system on the same metric that you are interested in evaluating, the system performs better,” Torkkola says. The new feed forward network delivered a significant improvement in forecasting relative to SQRF.
This was the breakthrough that the team had been working towards: the team could finally start retiring the plethora of old models and utilize a unified forecasting model that would produce accurate forecasts for multiple scenarios, forecasts, and categories. The result was a 15-fold improvement in forecast accuracy and great simplification of the entire system.
At last, no feature engineering!
While the feed forward network had delivered an impressive improvement in performance, the system still continued using the same hand engineered features SQRF had used. "There was no way to tell how far those features were from optimal," Ruofeng Wen, senior applied scientist who formerly worked as a forecasting scientist and joined the project in 2016, pointed out. “Some were redundant, and some were useless.”
The team set out to develop a model that would remove the need to manually engineer domain-specific features, thus being applicable to any general Forecasting problem. The breakthrough approach, known as MQ-RNN/CNN, was published in a 2018 paper titled "A Multi-Horizon Quantile Recurrent Forecaster". It built off the recent advances made in recurrent networks (RNN) and convolutional networks (CNNs).
CNNs are frequently used in image recognition due to their ability to scan an image, determine the saliency of various parts of that image, and make decisions about the relative importance of those facets. RNNs are usually used in a different domain, parsing semantics and sentiments from texts. Crucially, both RNNs/CNNs are able to extract the most relevant features without manual engineering. “Afterall, forecasting is based on past sequential patterns,” Wen said, “and RNNs/CNNs are pretty good at capturing them.”
Leveraging the new general approach allowed Amazon to forecast the demand of any fast-moving products by a single model structure. This out-performed a dozen of legacy systems designed for difference product lines, since the model was smart enough to learn business-specific demand patterns all by itself. However, for a system to make accurate predictions about the future, it has to have a detailed understanding of the errors it has made in the past. However, the architecture of Multi-Horizon Quantile Recurrent Forecaster had few mechanisms that would enable the model to ingest knowledge about past errors.
Amazon’s forecasting team worked through this limitation by turning to the latest advances in natural language processing (NLP).
Leaning on natural language processing
Dhruv Madeka, a principal applied scientist who had conducted innovative work in developing election forecasting systems at Bloomberg, was among the scientists who had joined Amazon’s forecasting team in 2017.
“Sentences are a sequence of words,” Madeka says. “The attention mechanisms in many NLP models look at a sequence of words, and determine which other parts of the sentence are important for a given context and task. By incorporating these context-aware mechanisms, we now had a way to make our forecasting system pay attention to its history, and gain an understanding of the errors it had made in the past.”
Amazon’s forecasting team honed in on the transformer architectures that were shaking up the world of NLP. Their new approach, which used decoder-encoder attention mechanisms for context-alignment, was outlined in the paper "MQTransformer: Multi-Horizon Forecasts with Context Dependent and Feedback-Aware Attention" published in December 2020. The decoder-encoder attention mechanisms meant that the system could study its own history and improve forecasting accuracy and decrease the volatility of the forecast.
With MQ Transformer, Amazon now has a unified forecasting model able to make even more accurate predictions across the company’s vast catalog of products.
Today, the team is developing deep reinforcement learning models that will enable Amazon to ensure that the accuracy improvements in forecasts translate directly into cost savings, resulting in lower costs for customers. To design a system that optimizes directly for savings — as opposed to inventory levels — the forecasting team is drawing on cutting-edge research from fields such as deep reinforcement learning.
“Amazon is an exceptional place for a scientist because of the focus on innovation grounded on making a real impact,” says Xu. “Thinking big is more than having a bold vision. It involves planting seeds, growing it continuously by failing fast, and doubling down on scaling once the evidence of success becomes apparent.”