Packet loss is a big problem for real-time voice communication over the Internet. Everyone has been in the situation where the network is becoming unreliable and enough packets are getting lost that it's hard — or impossible — to make out what the other person is saying.
One way to fight packet loss is through redundancy, in which each new packet includes information about prior packets. But existing redundancy schemes either have limited scope — carrying information only about the immediately preceding packet, for instance — or scale inefficiently.
The Deep REDundancy (DRED) technology from the Amazon Chime SDK team significantly improves quality and intelligibility under packet loss by efficiently transmitting large amounts of redundant information. Our approach leverages the ability of neural vocoders to reconstruct informationally rich speech signals from informationally sparse frequency spectrum snapshots, and we use a neural encoder to compress those snapshots still further. With this approach, we are able to load a single packet with information about as many as 50 prior packets (one second of speech) with minimal increase in bandwidth.
We describe our approach in a paper that we will present at this year’s ICASSP.
Redundant audio
All modern codecs (coder/decoders) have so-called packet-loss-concealment (PLC) algorithms that attempt to guess the content of lost packets. Those algorithms work fine for infrequent, short losses, as they can extrapolate phonemes to fill in gaps of a few tens of milliseconds. However, they cannot (and certainly should not try to) predict the next phoneme or word from the conversation. To deal with significantly degraded networks, we need more than just PLC.
One option is the 25-year-old spec for REDundant audio data (often referred to as just RED). Despite its age, RED is still in use today and is one of the few ways of transmitting redundant data for WebRTC, a popular open-source framework for real-time communication over the Web. RED has the advantage of being flexible and simple to use, but it is not very efficient. Transmitting two copies of the audio requires ... twice the bitrate.
The Opus audio codec — which is the default codec for WebRTC — introduced a more efficient scheme for redundancy called low-bit-rate redundancy (LBRR). With LBRR, each new audio packet can include a copy of the previous packet, encoded at a lower bit rate. That has the advantage of lowering the bit rate overhead. Also, because the scheme is deeply integrated into Opus, it can be simpler to use than RED.
That being said, the Opus LBRR is limited to just one frame of redundancy, so it cannot do much in the case of a long burst of lost packets. RED does not have that limitation, but transmitting a large number of copies would be impractical due to the overhead. There is always the risk that the extra redundancy will end up causing congestion and more losses.
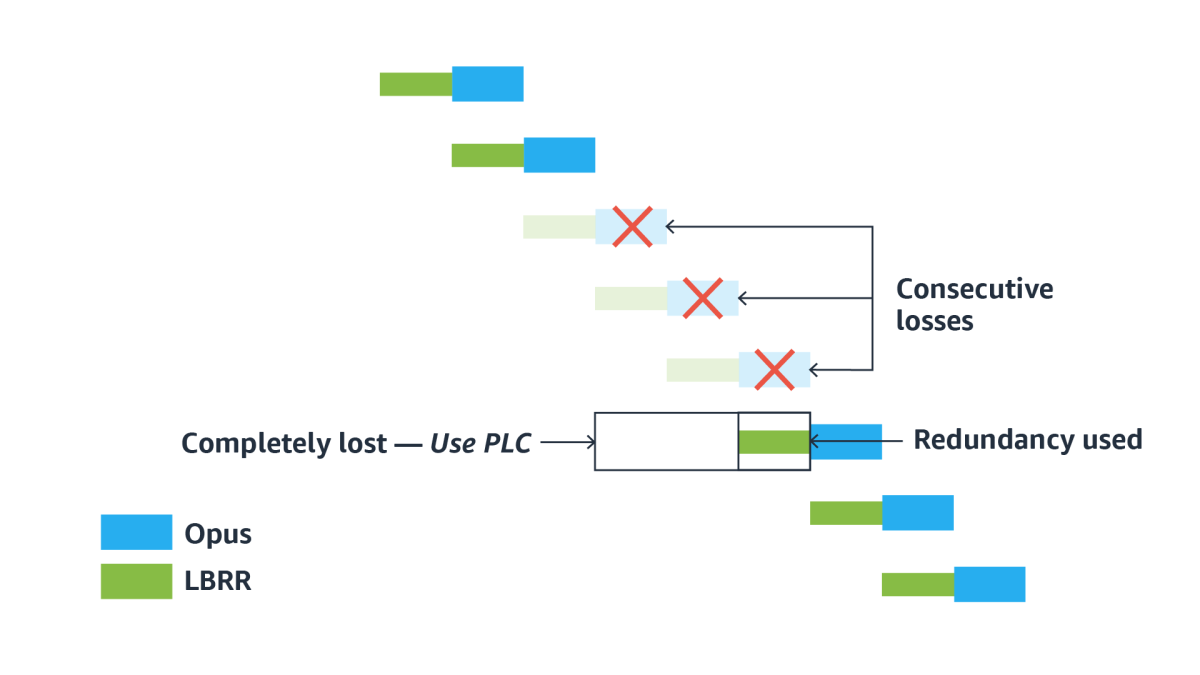
Deep REDundancy (DRED)
In the past few years, we have seen neural speech codecs that can produce good quality speech at only a fraction of the bit rate required by traditional speech codecs — typically less than three kilobits per second (3 kb/s). That was unthinkable just a few years ago. But for most real-time-communication applications, neural codecs aren't that useful, because just the packet headers required by the IP/UDP/RTP protocols take up 16 kb/s.
However, for the purpose of transmitting a large amount of redundancy, a neural speech codec can be very useful, and we propose a Deep REDundancy codec that has been specifically designed for that purpose. It has a different set of constraints than a regular speech codec:
- The redundancy in different packets needs to be independent (that's why we call it redundancy in the first place). However, within each packet, we can use as much prediction and other redundancy elimination as we like since IP packets are all-or-nothing (no corrupted packets).
- We want to encode meaningful acoustic features rather than abstract (latent) ones to avoid having to standardize more than needed and to leave room for future technology improvements.
- There is a large degree of overlap between consecutive redundancy packets. The encoder should leverage this overlap and should not need to encode each redundancy packet from scratch. The encoding complexity should remain constant even as we increase the amount of redundancy.
- Since short bursts are more common than long ones, the redundancy decoder should be able to decode the most recent audio quickly but may take longer to decode older signals.
- The Opus decoder has to be able to switch between decoding DRED, PLC, LBRR, and regular packets at any time.
Neural vocoders
Let's take a brief detour and discuss neural vocoders. A vocoder is an algorithm that takes in acoustic features that describe the spectrum of a speech signal over a short span of time and generates the corresponding (continuous) speech signal. Vocoders can be used in text-to-speech, where acoustic features are generated from text, and for speech compression, where the encoder transmits acoustic features, and a vocoder generates speech from the features.
Vocoders have been around since the ’70s, but none had ever achieved acceptable speech quality — until neural vocoders like WaveNet came about and changed everything. WaveNet itself was all but impossible to implement in real time (even on a GPU), but it led to lower-complexity neural vocoders, like the LPCNet vocoder we're using here.
Like many (but not all) neural vocoders, LPCNet is autoregressive, in that it produces the audio samples that best fit the previous samples — whether the previous samples are real speech or speech synthesized by LPCNet itself. As we will see below, that property can be very useful.
DRED architecture
The vocoder’s inputs — the acoustic features — don't describe the full speech waveform, but they do describe how the speech sounds to the human ear. That makes them lightweight and predictable and thus ideal for transmitting large amounts of redundancy.
The idea behind DRED is to compress the features as much as possible while ensuring that the recovered speech is still intelligible. When multiple packets go missing, we wait for the first packet to arrive and decode the features it contains. We then send those features to a vocoder — in our case, LPCNet — which re-synthesizes the missing speech for us from the point where the loss occurred. Once the "hole" is filled, we resume with Opus decoding as usual.
Combining the constraints listed earlier leads to the encoder architecture depicted below, which enables efficient encoding of highly redundant acoustic features — so that extended holes can be filled at the decoder.
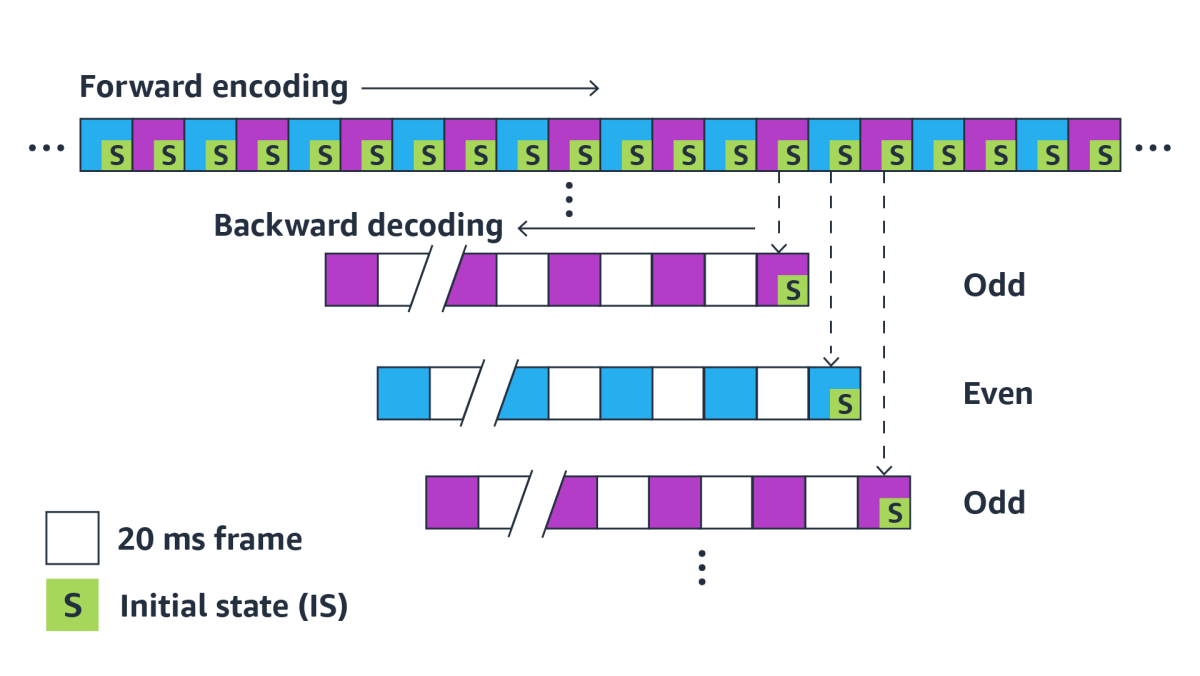
The DRED encoder works as follows. Every 20 milliseconds (ms), it produces a new vector that contains information about the last 40 ms of speech. Given this overlap, we need only half of the vectors to reconstruct the complete speech. To avoid our redundancy’s being itself redundant, in a given 20 ms packet, we include only every other redundancy coding vector, so the redundancy encoded in a given packet covers nonoverlapping segments of the past speech. In terms of the figure above, the signal can be recovered from just the odd/purple blocks or just the even/blue blocks.
The degree of redundancy is determined by the number of past chunks included in each packet; each chunk included in the redundancy coding corresponds to 40 ms of speech that can be recovered. Furthermore, rather than representing each chunk independently, the encoder takes advantage of the correlation between successive chunks and extracts a sort of interchunk difference to encode.
For decoding, to be able to synthesize the whole sequence, all we need is a starting point. But rather than decoding forward in time, as would be intuitive, we choose an initial state that corresponds to the most recent chunk; from there, we decode going backward in time. That means we can get quickly to the most recent audio, which is more likely to be useful. It also means that we can transmit as much — or as little — redundancy as we want just by choosing how many chunks to include in a packet.
Rate-distortion-optimized variational autoencoder
Now let's get into the details of how we minimize the bit rate to code our redundancy. Here we turn to a widely used method in the video coding world, rate distortion optimization (RDO), which means trying to simultaneously reduce the bit rate and the distortion we cause to the speech. In a regular autoencoder, we train an encoder to find a simple — typically, low-dimensional — vector representation of an input that can then be decoded back to something close to the original.
In our rate-distortion-optimized variational autoencoder (RDO-VAE), instead of imposing a limit on the dimensionality of the representation, we directly limit the number of bits required to code that representation. We can estimate the actual rate (in bits) required to code the latent representation, assuming entropy coding of a quantized Laplace distribution. As a result, not only do we automatically optimize the feature representation, but the training process automatically discards any useless dimensions by setting them to zero. We don't need to manually choose the number of dimensions.
Moreover, by varying the rate-distortion trade-off, we can train a rate-controllable quantizer. That allows us to use better quality for the most recent speech (which is more likely to be used) and a lower quality for older speech that would be used only for a long burst of loss. In the end, we use an average bit rate of around 500 bits/second (0.5 kb/s) and still have enough information to reconstruct intelligible speech.
Once we include DRED, this is what the packet loss scenario described above would look like:
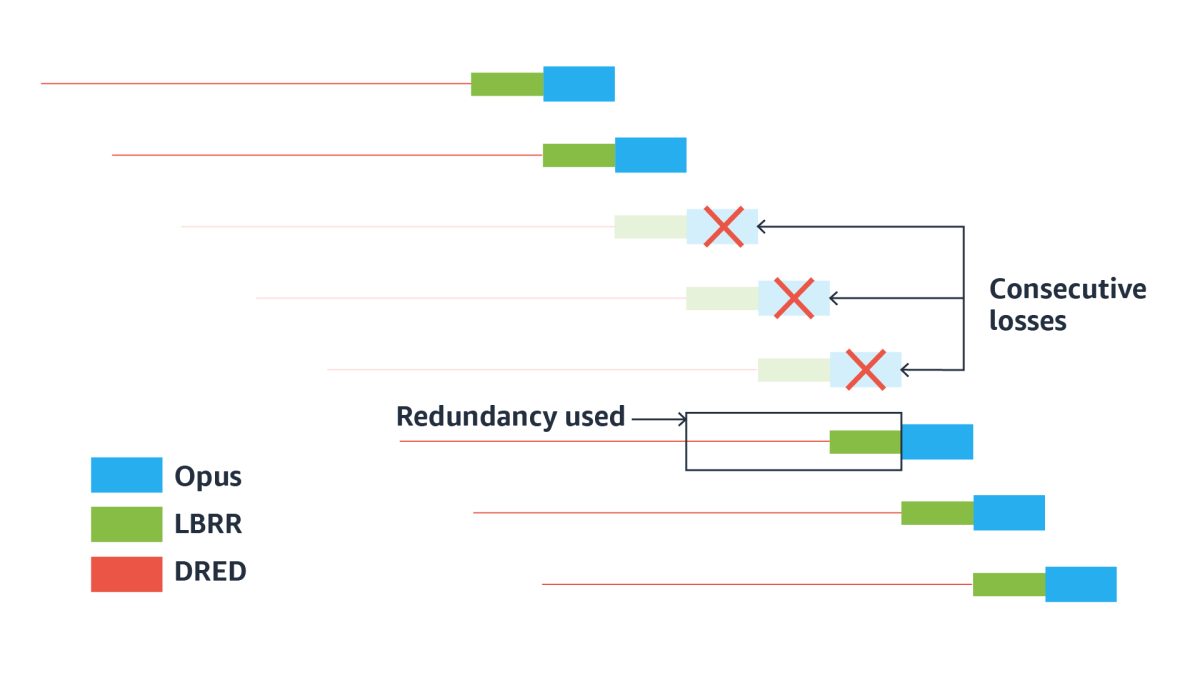
Although it is illustrated for just 70 milliseconds of redundancy, we scale this up to one full second of redundancy contained in each 20-millisecond packet. That's 50 copies of the information being sent, on the assumption that at least one will make it to its destination and enable reconstruction of the original speech.
Revisiting packet loss concealment
So what happens when we lose a packet and don't have any DRED data for it? We still need to play out something — and ideally not zeros. In that case, we can just guess. Over a short period of time, we can still predict acoustic features reasonably well and then ask LPCNet to fill in the missing audio based on those features. That is essentially what PLC does, and doing it with a neural vocoder like LPCNet works better than using traditional PLC algorithms like the one that's currently integrated into Opus. In fact, our neural PLC algorithm recently placed second in the Interspeech 2022 Audio Deep Packet Loss Concealment Challenge.
Results
How much does DRED improve speech quality and intelligibility under lossy network conditions? Let's start with a clip compressed with Opus wideband at 24 kb/s, plus 16 kb/s of LBRR redundancy (40 kb/s total). This is what we get without loss:
To show what happens in lossy conditions, let's use a particularly difficult — but real — loss sequence taken from the PLC Challenge. If we use the standard Opus redundancy (LBRR) and PLC, the resulting audio is missing large chunks that just cannot be filled:
If we add our DRED coding with one full second of redundancy included in each packet, at a cost of about 32 kb/s, the missing speech can be entirely recovered:
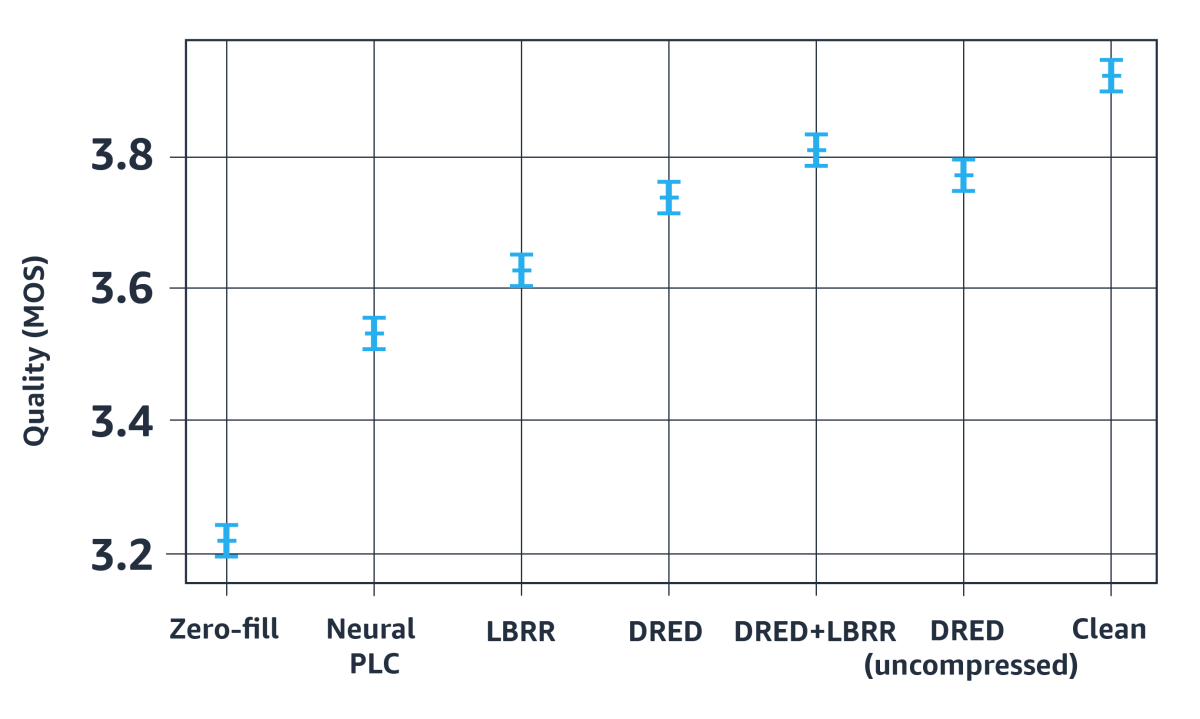
The example above is based on just one speech sequence, but we evaluated DRED on the full dataset for the original PLC Challenge, using mean opinion score (MOS) to aggregate the judgments of human reviewers. The results show that DRED alone (no LBRR) can reduce the impact of packet loss by about half even compared to our previous neural PLC. Also interesting is the fact that LBRR still provides a benefit even when DRED is used. With both LBRR and DRED, the impact of packet loss becomes very small, with just a 0.1 MOS degradation compared to the original, uncompressed speech.
This work is only one example of how Amazon is contributing to improving Opus. Our open-source neural PLC and DRED implementations are available on this development branch, and we welcome feedback and outside collaboration. We are also engaging with the IETF with the goal of updating the Opus standard in a fully compatible way. Our two Internet drafts (draft 1 | draft 2) offer more details on what we are proposing.