Editor’s note: Michele Donini is a senior applied scientist with Amazon Web Services (AWS). He and his co-author, Luca Oneto, associate professor of computer engineering at University of Genoa, have written about how different approaches can make data-driven predictions fairer for underrepresented groups. Oneto also won a 2019 Machine Learning Research award for his work on algorithmic fairness. In this article, Donini and Oneto explore the research they and other collaborators have published related to designing machine learning (ML) models from a human-centered perspective, and building responsible AI.
What is fairness?
Fairness can be defined in many different ways, and many different formal notions exist, such as demographic parity, equal opportunity, and equal odds.
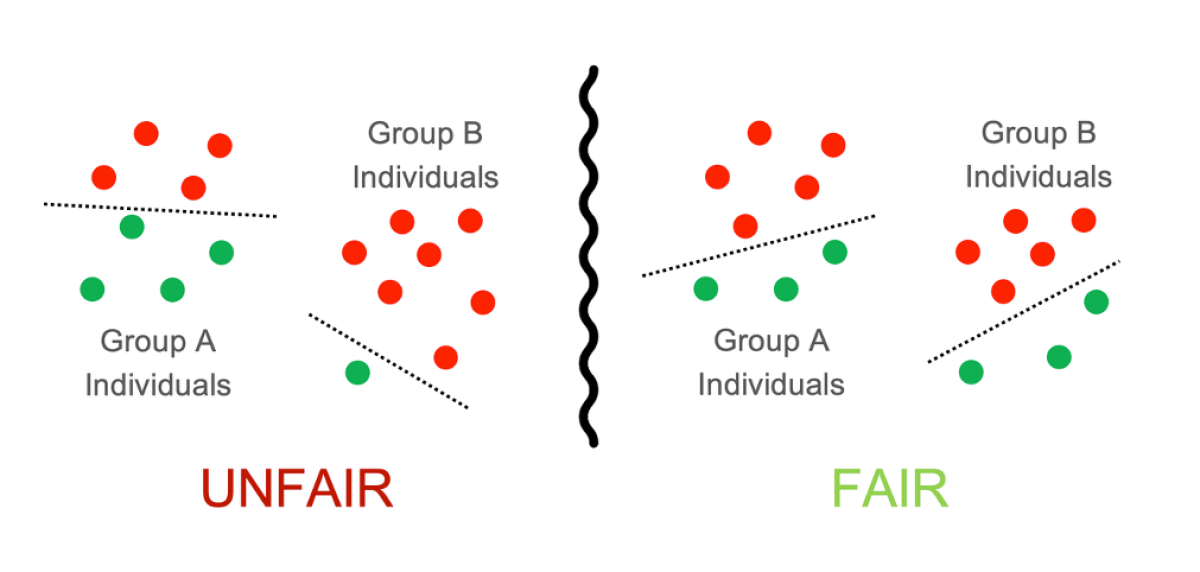
Nevertheless, the basic and common idea behind notions of fairness is that the learned ML model should behave equivalently, or at least similarly, no matter whether it is applied to one subgroup of the population (e.g., males) or to another one (e.g., females).
For example, demographic parity, which arguably is the most common notion of fairness, implies that the probability of a certain output of an ML model (e.g., deciding to make a loan) should not depend on the value of specific demographic attributes (e.g., gender, race, or age).
Moving toward fairer models
Broadly speaking, we can group current literature on algorithmic fairness into three main approaches:
- The first approach consists of pre-processing the data to remove historical biases and then feeding this data to classical ML models.
- The second approach consists of post-processing an already learned ML model. This approach is useful when very complex ML models need to be made fairer without touching their inner structure or when re-training them is unfeasible (due to computational cost, or time requirements).
- The third approach, called in-processing, consists of enforcing fairness notions by imposing specific statistical constraints during the learning phase of the model. This is the most natural approach, but so far, it has required ad hoc solutions tailored to specific tasks and data sets.
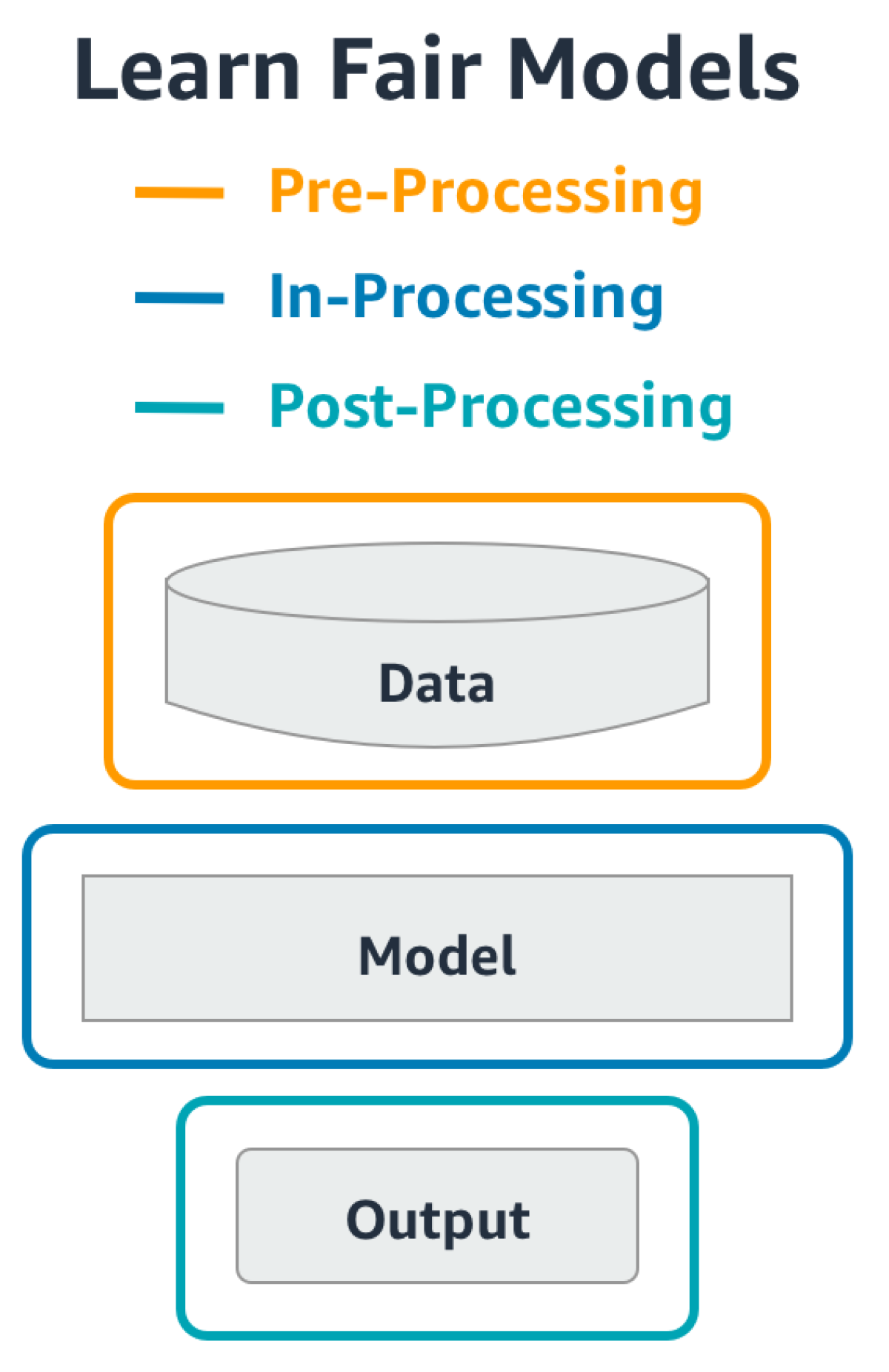
We decided to explore and analyze possible techniques to make ML algorithms capable of learning fairer models.
We started from the base concepts of statistical learning theory — a mathematical framework for describing machine learning — and, in particular, from empirical risk minimization theory. The core concept of empirical risk minimization is that a model’s performance on test data may not accurately predict its performance on real-world data, as the real-world data may have a different probability distribution.
Empirical-risk-minimization theory provides a way to estimate the “true risk” of a model from its “empirical risk”, which can be computed from the available data. We extended this concept to the true and empirical fairness risk of ML models.
Below is a summary of three papers we’ve published related to these topics.
“Empirical risk minimization under fairness constraints”
This paper presents a new in-processing method, meaning that we incorporate a fairness constraint into the learning problem. We derive theoretical guarantees on both the accuracy and fairness of the resulting models, and we show how to apply our method to a large family of machine learning algorithms, including linear models and support vector machines for classification (a widely used supervised-learning method).
We observe that, in practice, we can meet our fairness constraint simply by requiring that a scalar product between two vectors remains small (an orthogonality constraint between the vector of the weights describing our model and the vector describing the discrimination between the different subgroups). We further observe that, for linear models, this requirement translates into a simple pre-processing method. Experiments indicate that our approach is empirically effective and performs favorably against state-of-the-art approaches.
“Fair regression with Wasserstein barycenters”
In this paper, we consider the case in which the ML model learns a regression function (as opposed to a classification task). We propose a post-processing method for transforming a real-valued regression function — the ML model — into one that satisfies the demographic-parity constraint (i.e., the probability of getting a positive outcome should be virtually the same for different subgroups). In particular, the new regression function is as good an approximation of the original as is possible while still satisfying the constraint, making it an optimal fair predictor.
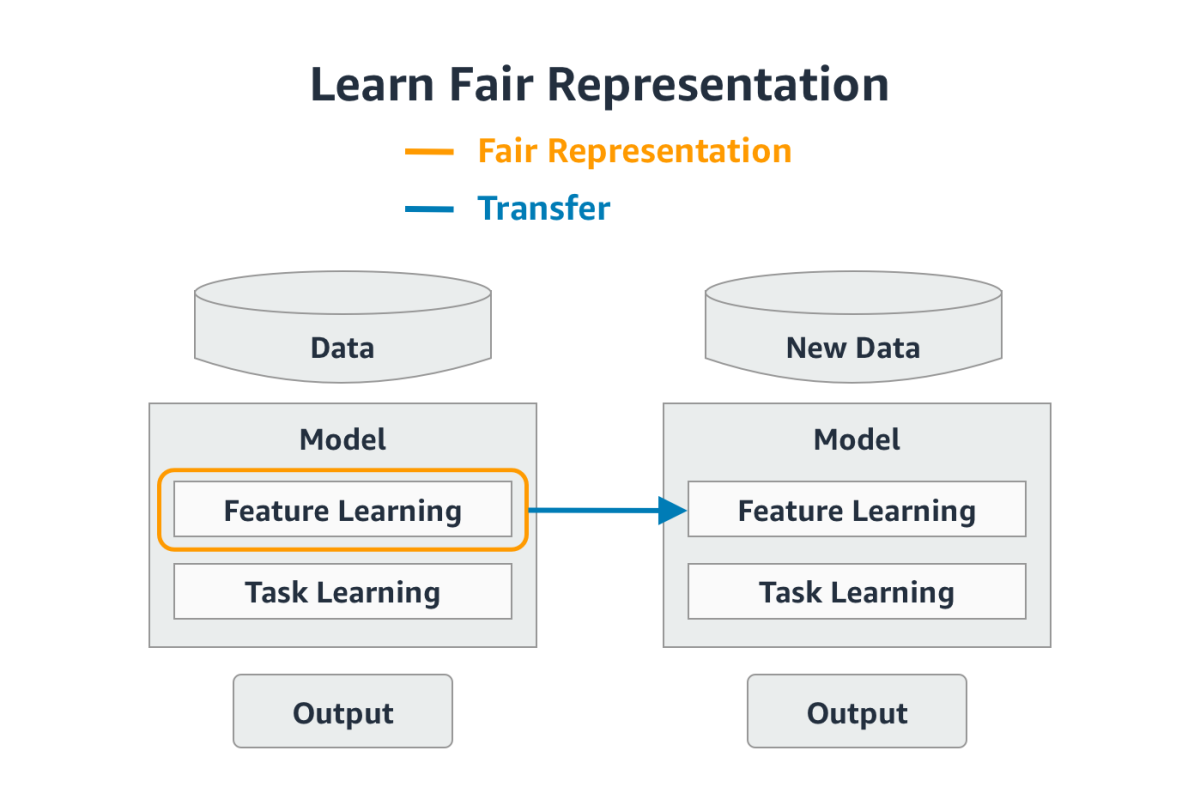
We assume that the sensitive attribute — the demographic attribute that should not bias outcome — is available to the ML model at inference time and not only during training. We establish a connection between learning a fair model for regression and optimal transport theory, which describes how to measure distances among probability distributions. On that basis, we derive a closed-form expression for the optimal fair predictor.
Specifically, under the unfair regression function, different populations have different probability distributions; the function skews the probabilities for the population with the sensitive attribute. The difference between subgroups’ distributions can be calculated using the Wasserstein distance. We show that the mean of the distribution of the optimal fair predictor is the mean of the different subgroups’ distributions, as calculated using Wasserstein distance. This mean is known as the Wasserstein barycenter.
This result offers an intuitive interpretation of optimal fair prediction and suggests a simple post-processing algorithm to achieve fairness. We establish fairness-risk guarantees for this procedure. Numerical experiments indicate that our method is very effective in learning fair models, with a relative increase in error rate that is smaller than the relative gain in fairness.
"Exploiting MMD and Sinkhorn divergences for fair and transferable representation learning”
Where the first paper described a general learning method, and the second a regression method, this paper concerns deep learning. We show how to improve demographic parity in the multitask-learning setting, in which a deep-learning model learns a single representation of the input data that is useful for multiple tasks. We derive theoretical guarantees on the learned model, establishing that the representation will still reduce bias even when transferred to novel tasks.
We propose a learning algorithm that imposes constraints based on two different ways of measuring distances between probability distributions, maximum mean discrepancy and Sinkhorn divergence. Keeping this distance small ensures that we represent similar inputs in a similar way when they differ only on the sensitive attribute. We present experiments on three real-world datasets, showing that the proposed method outperforms state-of-the-art approaches by a significant margin.
Algorithmic fairness is a topic of great importance, with impact on many applications. In our work, we have attempted to take a small step forward, but the issue requires much further research; even the definition of what “being fair” means for an ML model is still an open research question.
It’s also becoming clearer that we need to keep humans in the loop during the lifecycle of ML models, to evaluate whether the models are acting as we would like them to. In this sense, it is important to note that many other research subjects – such as the explainability, interpretability, and privacy of ML models – are deeply connected to algorithmic fairness. They can work in synergy, with the common goal of increasing the trustworthiness of ML models.