Robotic-ad-click detection is the task of determining whether an ad click on an e-commerce website was initiated by a human or a software agent. Its goal is to ensure that advertisers’ campaigns are not billed for robotic activity and that human clicks are not invalidated. It must act in real time, to cause minimal disruption to the advertiser experience, and it must be scalable, comprehensive, precise, and able to respond rapidly to changing traffic patterns.
At this year’s Conference on Innovative Applications of Artificial Intelligence (IAAI) — part of AAAI, the annual meeting of the Association for the Advancement of Artificial Intelligence — we presented SLIDR, or SLIce-Level Detection of Robots, a real-time deep-neural-network model trained with weak supervision to identify invalid clicks on online ads. SLIDR has been deployed on Amazon since 2021, safeguarding advertiser campaigns against robotic clicks.
In the paper, we formulate a convex optimization problem that enables SLIDR to achieve optimal performance on individual traffic slices, with a budget of overall false positives. We also describe our system design, which enables continuous offline retraining and large-scale real-time inference, and we share some of the important lessons we’ve learned from deploying SLIDR, including the use of guardrails to prevent updates of anomalous models and disaster recovery mechanisms to mitigate or correct decisions made by a faulty model.
Challenges
Detecting robotic activity in online advertising faces various challenges: (1) precise ground-truth labels with high coverage are hard to come by; (2) bot behavior patterns are continuously evolving; (3) bot behavior patterns vary significantly across different traffic slices (e.g., desktop vs, mobile); and (4) false positives reduce ad revenue.
Labels
Since accurate ground truth is unavailable at scale, we generate data labels by identifying two high-hurdle activities that are very unlikely to be performed by a bot: (1) ad clicks that lead to purchases and (2) ad clicks from customer accounts with high RFM scores. RFM scores represent the recency (R), frequency (F), and monetary (M) value of customers’ purchasing patterns on Amazon. Clicks of either sort are labeled as human; all remaining clicks are marked as non-human.
Metrics
Due to the lack of reliable ground truth labels, typical metrics such as accuracy cannot be used to evaluate the model performance. So we turn to a trio of more-specific metrics.
Invalidation rate (IVR) is defined as the fraction of total clicks marked as robotic by the algorithm. IVR is indicative of the recall of our model, since a model with a higher IVR is more likely to invalidate robotic clicks.
On its own, however, IVR can be misleading, since a poorly performing model will invalidate human and robot clicks. Hence we measure IVR in conjunction with the false-positive rate (FPR). We consider purchasing clicks as a proxy for the distribution of human clicks and define FPR as the fraction of purchasing clicks invalidated by the algorithm. Here, we make two assumptions: (1) all purchasing clicks are human, and (2) purchasing clicks are a representative sample of all human clicks.
We also define a more precise variant of recall by checking the model’s coverage over a heuristic that identifies clicks with a high likelihood to be robotic. The heuristic labels all clicks in user sessions with more than k ad clicks in an hour as robotic. We call this metric robotic coverage.
A neural model for detecting bots
We consider various input features for our model that will enable it to disambiguate robotic and human behavior:
- User-level frequency and velocity counters compute volumes and rates of clicks from users over various time periods. These enable identification of emergent robotic attacks that involve sudden bursts of clicks.
- User entity counters keep track of statistics such as number of distinct sessions or users from an IP. These features help to identify IP addresses that may be gateways with many users behind them.
- Time of click tracks hour of day and day of week, which are mapped to a unit circle. Although human activity follows diurnal and weekly activity patterns, robotic activity often does not.
- Logged-in status differentiates between customers and non-logged-in sessions as we expect a lot more robotic traffic in the latter.
The neural network is a binary classifier consisting of three fully connected layers with ReLU activations and L2 regularization in the intermediate layers.
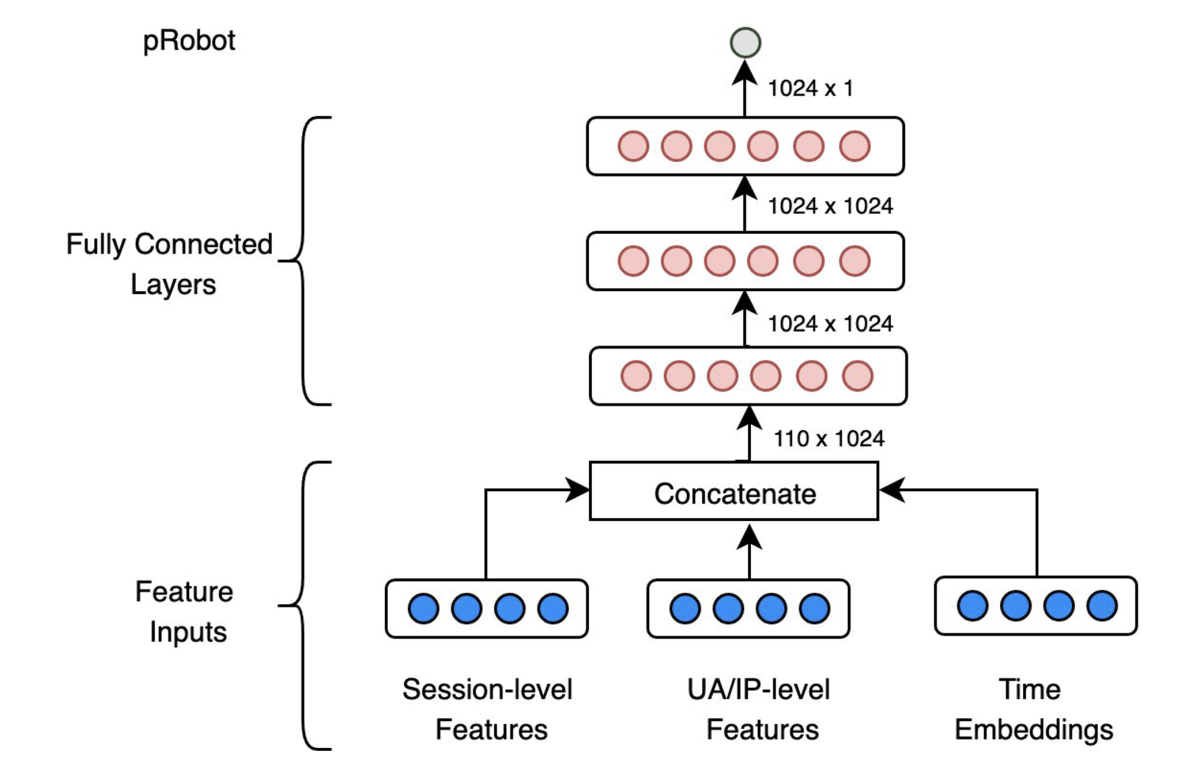
While training our model, we use sample weights that weigh clicks equivalently across hour of day, day of the week, logged-in status, and the label value. We have found sample weights to be crucial in improving the model’s performance and stability, especially with respect to sparse data slices such as night hours.
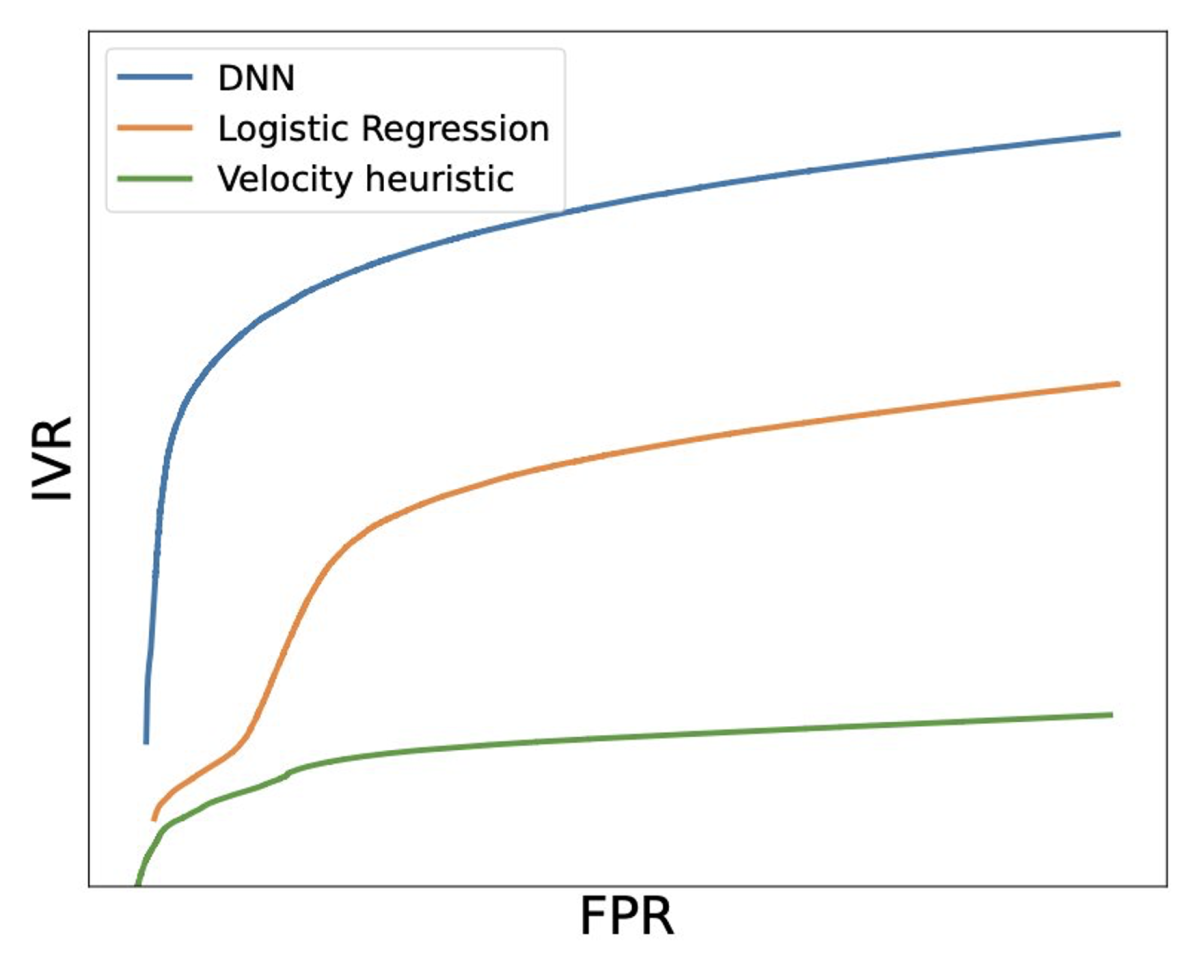
We compare our model against baselines such as logistic regression and a heuristic rule that computes velocity scores of clicks. Both the baselines lack the ability to model complex patterns and hence are unable to perform as well as the neural network.
Calibration
Calibration involves choosing a threshold for the model’s output probability above which all clicks are marked as invalid. The model should invalidate certain highly robotic clicks but at the same time not incur high revenue loss by invalidating human clicks. Toward this, one option is to pick the “knee” of the IVR-FPR curve, beyond which the false positive rate increases sharply when compared to the increase in IVR.
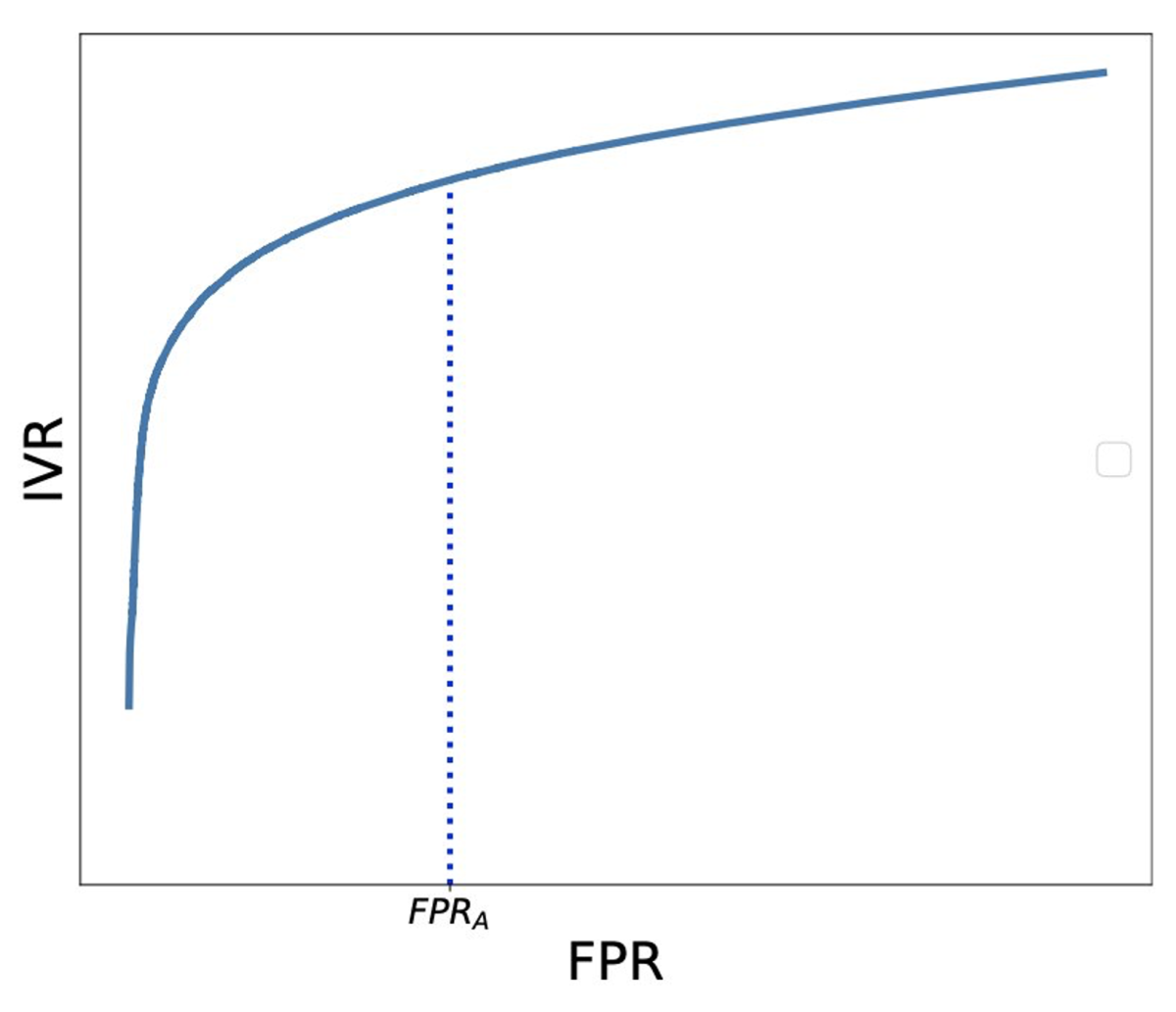
But calibrating the model across all traffic slices together leads to different behaviors for different slices. For example, a decision threshold obtained via overall calibration, when applied to the desktop slice, could be undercalibrated: a lower probability threshold could invalidate more bots. Similarly, when the global decision threshold is applied to the mobile slice, it could be overcalibrated: a higher probability threshold might be able to recover some revenue loss without compromising on the bot coverage.
To ensure fairness across all traffic slices, we formulate calibration as a convex optimization problem. We perform joint optimization across all slices by fixing an overall FPR budget (an upper limit to the FPR of all slices combined) and solve to maximize the combined IVR on all slices together. The optimization must meet two conditions: (1) each slice has a minimum robotic coverage, which establishes a lower found for its FPR, and (2) the combined FPR of all slices should not exceed the FPR budget.
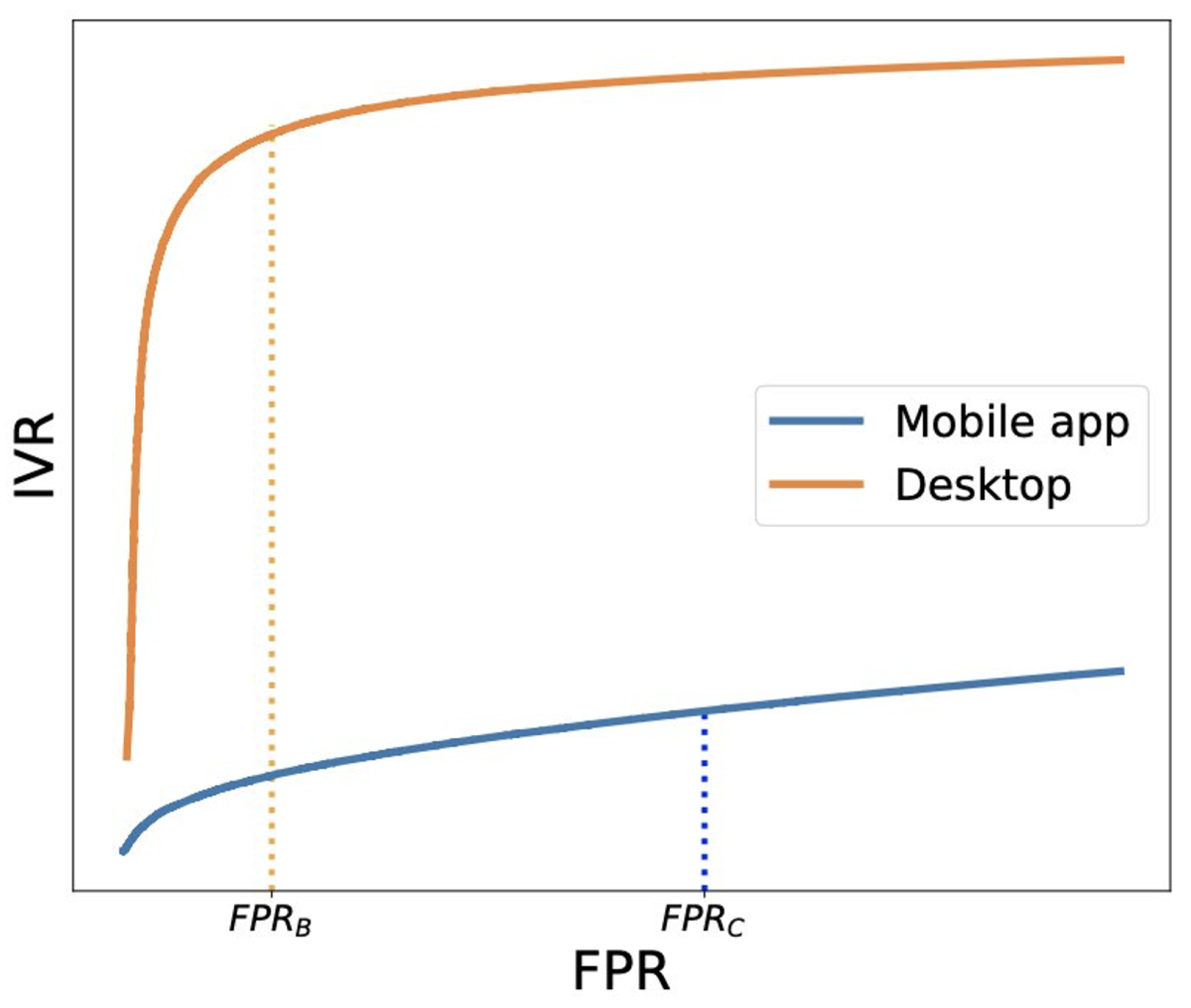
Since the IVR-FPR curve of each slice can be approximated as a quadratic function of the FPR, solving the joint optimization problem finds appropriate values for each slice. We have found slice-level calibration to be crucial in lowering overall FPR and increasing robotic coverage.
Deployment
To quickly adapt to changing bot patterns, we built an offline system that retrains and recalibrates the model on a daily basis. For incoming traffic requests, the real-time component computes the feature values using a combination of Redis and read-only DB caches and runs the neural-network inference on a horizontally scalable fleet of GPU instances. To meet the real-time constraint, the entire inference service, which runs on AWS, has a p99.9 latency below five milliseconds.
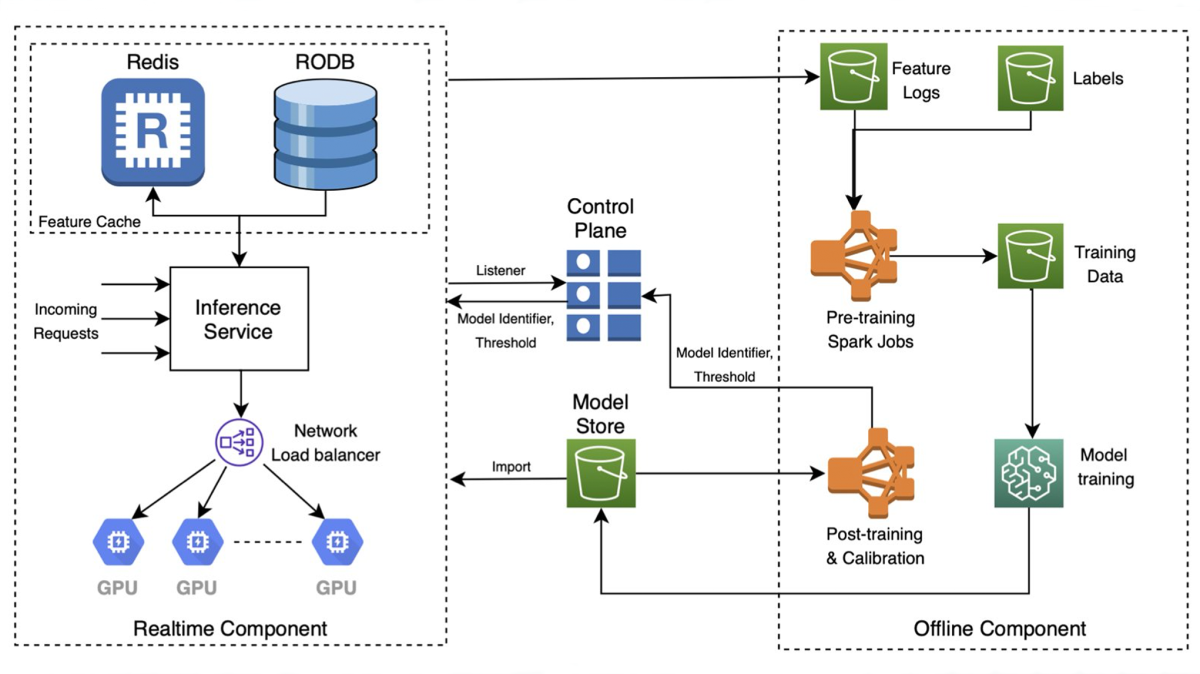
To address data and model anomalies during retraining and recalibration, we put certain guardrails on the input training data and the model performance. For example, when purchase labels are missing for a few hours, the model can learn to invalidate a large amount of traffic. Guardrails such as minimum human density in every hour of a week prevent such behavior.
We have also developed disaster recovery mechanisms such as quick rollbacks to a previously stable model when a sharp metric deviation is observed and a replay tool that can replay traffic through a previously stable model or recompute real-time features and publish delayed decisions, which help prevent high-impact events.
In the future, we plan to add more features to the model, such as learned representations for users, IPs, UserAgents, and search queries. We presented our initial work in that direction in our NeurIPS 2022 paper, “Self supervised pre-training for large scale tabular data”. We also plan to experiment with advanced neural architectures such as deep and cross-networks, which can effectively capture feature interactions in tabular data.
Acknowledgements: Muneeb Ahmed