Customer-obsessed science
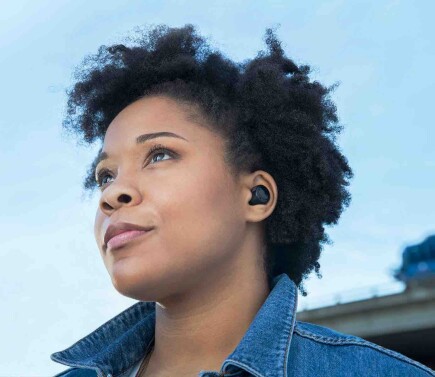
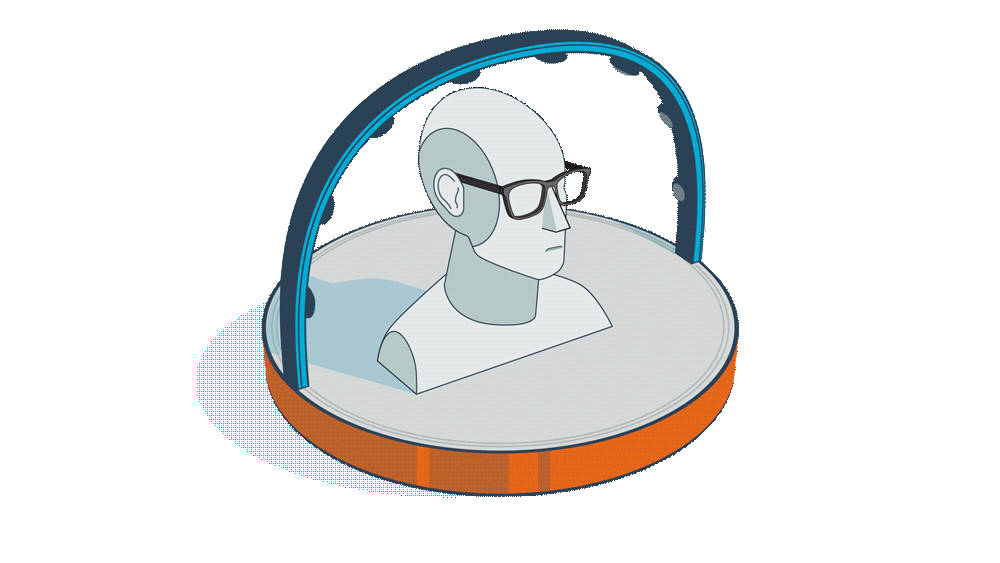
Research areas
-
April 11, 2025Novel three-pronged approach combines claim-level evaluations, chain-of-thought reasoning, and classification of hallucination error types.
-
-
Featured news
-
AISTATS 20252025We study the well-motivated problem of online distribution shift in which the data arrive in batches and the distribution of each batch can change arbitrarily over time. Since the shifts can be large or small, abrupt or gradual, the length of the relevant historical data to learn from may vary over time, which poses a major challenge in designing algorithms that can automatically adapt to the best “attention
-
2025We introduce AnoLLM, a novel framework that leverages large language models (LLMs) for unsupervised tabular anomaly detection. By converting tabular data into a standardized text format, we further adapt a pre-trained LLM with this serialized data, and assign anomaly scores based on the negative log likelihood generated by the LLM. Unlike traditional methods that can require extensive feature engineering
-
CLeaR 20252025We propose a new approach to falsify causal discovery algorithms without ground truth, which is based on testing the causal model on a variable pair excluded during learning the causal model. Specifically, given data on X,Y,Z = X,Y,Z1,...,Zk, we apply the causal discovery algorithm separately to the ’leave-one-out’ data sets X,Z and Y,Z. We demonstrate that the two resulting causal models, in the form of
-
AISTATS 20252025Modeling and analysis for event series generated by users of heterogeneous behavioral patterns are closely involved in our daily lives, including credit card fraud detection, online platform user recommendation, and social network analysis. The most commonly adopted approach to this task is to assign users to behavior-based categories and analyze each of them separately. However, this requires extensive
-
M2AD: Multi-sensor multi-system anomaly detection through global scoring and calibrated thresholdingAISTATS 20252025With the widespread availability of sensor data across industrial and operational systems, we frequently encounter heterogeneous time series from multiple systems. Anomaly detection is crucial for such systems to facilitate predictive maintenance. However, most existing anomaly detection methods are designed for either univariate or single-system multivariate data, making them insufficient for these complex
Academia
View allWhether you're a faculty member or student, there are number of ways you can engage with Amazon.
View all