This week, at Amazon’s Delivering the Future symposium in Dortmund, Germany, Amazon announced that its Vulcan robots, which stow items into and pick items from fabric storage pods in Amazon fulfillment centers (FCs), have completed a pilot trial and are ready to move into beta testing.
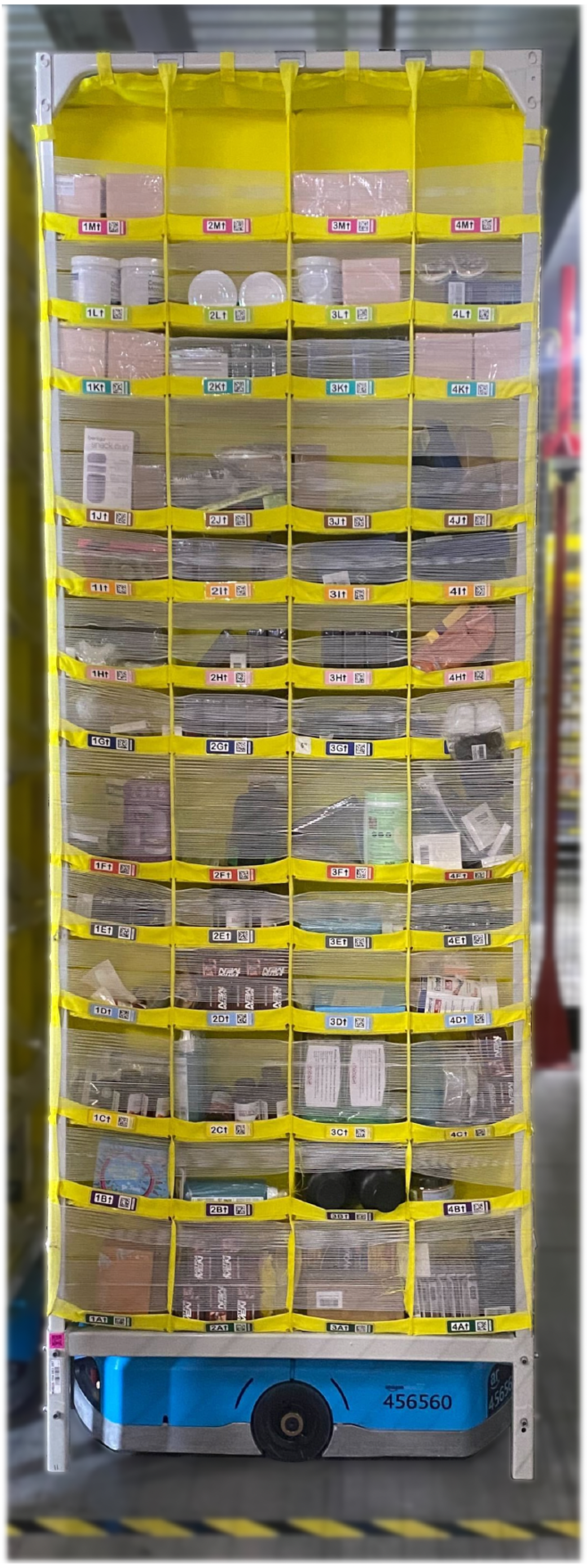
Amazon FCs already use robotic arms to retrieve packages and products from conveyor belts and open-topped bins. But a fabric pod is more like a set of cubbyholes, accessible only from the front, and the items in the individual cubbies are randomly assorted and stacked and held in place by elastic bands. It’s nearly impossible to retrieve an item from a cubby or insert one into it without coming into physical contact with other items and the pod walls.
The Vulcan robots thus have end-of-arm tools — grippers or suction tools — equipped with sensors that measure force and torque along all six axes. Unlike the robot arms currently used in Amazon FCs, the Vulcan robots are designed to make contact with random objects in their work environments; the tool sensors enable them to gauge how much force they are exerting on those objects — and to back off before the force becomes excessive.
“A lot of traditional industrial automation — think of welding robots or even the other Amazon manipulation projects — are moving through free space, so the robot arms are either touching the top of a pile, or they're not touching anything at all,” says Aaron Parness, a director of applied science with Amazon Robotics, who leads the Vulcan project. “Traditional industrial automation, going back to the ’90s, is built around preventing contact, and the robots operate using only vision and knowledge of where their joints are in space.
“What's really new and unique and exciting is we are using a sense of touch in addition to vision. One of the examples I give is when you as a person pick up a coin off a table, you don't command your fingers to go exactly to the specific point where you grab the coin. You actually touch the table first, and then you slide your fingers along the table until you contact the coin, and when you feel the coin, that's your trigger to rotate the coin up into your grasp. You're using contact both in the way you plan the motion and in the way you control the motion, and our robots are doing the same thing.”
The Vulcan pilot involved six Vulcan Stow robots in an FC in Spokane, Washington; the beta trial will involve another 30 robots in the same facility, to be followed by an even larger deployment at a facility in Germany, with Vulcan Stow and Vulcan Pick working together.
Inside the fulfillment center
When new items arrive at an FC, they are stowed in fabric pods at a stowing station; when a customer places an order, the corresponding items are picked from pods at a picking station. Autonomous robots carry the pods between the FC’s storage area and the stations. Picked items are sorted into totes and sent downstream for packaging.
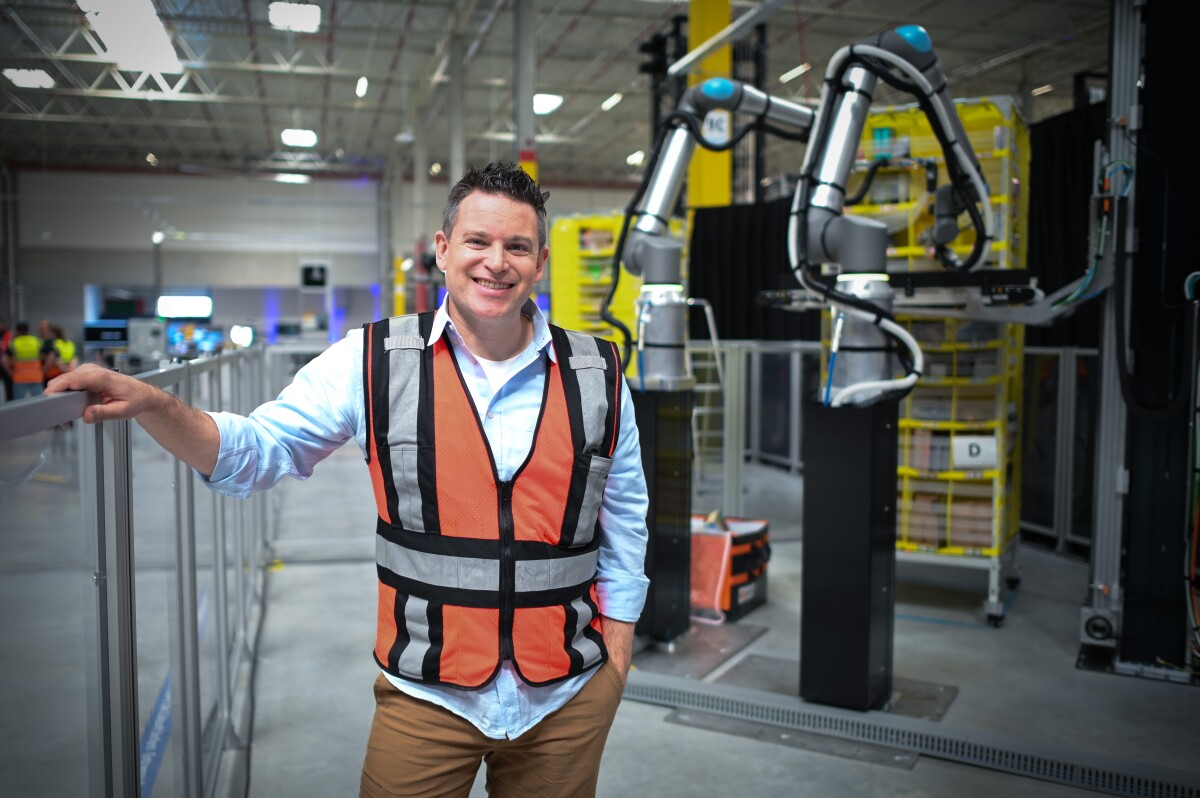
The allocation of items to pods and pod shelves is fairly random. This may seem counterintuitive, but in fact it maximizes the efficiency of the picking and stowing operations. An FC might have 250 stowing stations and 100 picking stations. Random assortment minimizes the likelihood that any two picking or stowing stations will require the same pod at the same time.
To reach the top shelves of a pod, a human worker needs to climb a stepladder. The plan is for the Vulcan robots to handle the majority of stow and pick operations on the highest and lowest shelves, while humans will focus on the middle shelves and on more challenging operations involving densely packed bins or items, such as fluid containers, that require careful handling.
End-of-arm tools
The Vulcan robots' main hardware innovation is the end-of-arm tools (EOATs) they use to perform their specialized tasks.
The pick robot’s EOAT is a suction device. It also has a depth camera to provide real-time feedback on the way in which the contents of the bin have shifted in response to the pick operation.
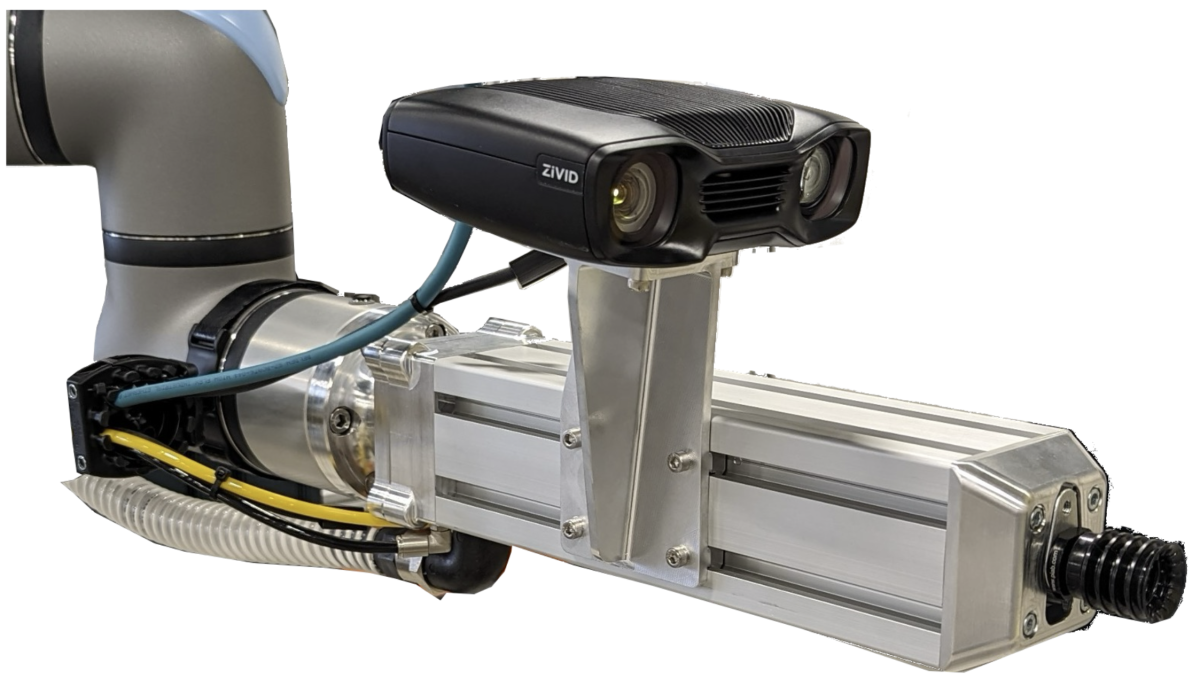
The stow EOAT is a gripper with two parallel plates that sandwich the item to be stowed. Each plate has a conveyer belt built in, and after the gripper moves into position, it remains stationary as the conveyer belts slide the item into position. The stow EOAT also has an extensible aluminum attachment that’s rather like a kitchen spatula, which it uses to move items in the bin aside to make space for the item being stowed.
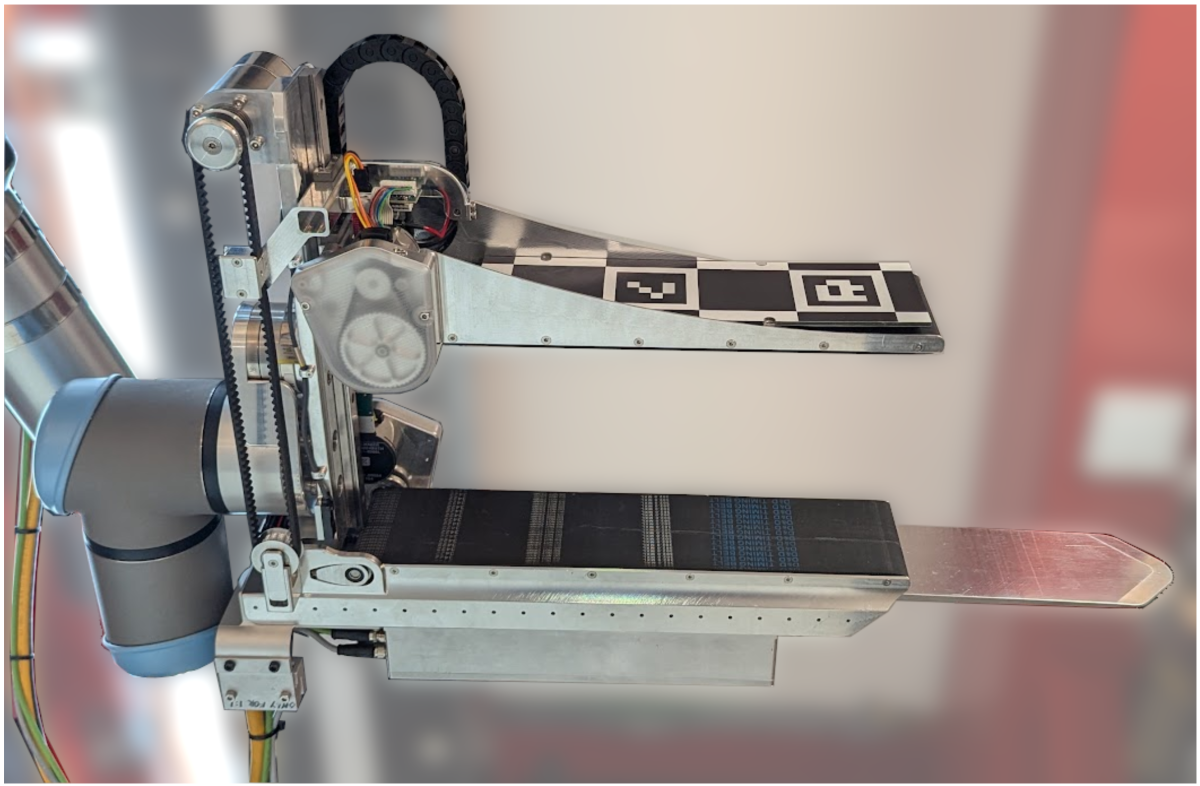
Both the pick and stow robots have a second arm whose EOAT is a hook, which is used to pull down or push up the elastic bands covering the front of the storage bin.
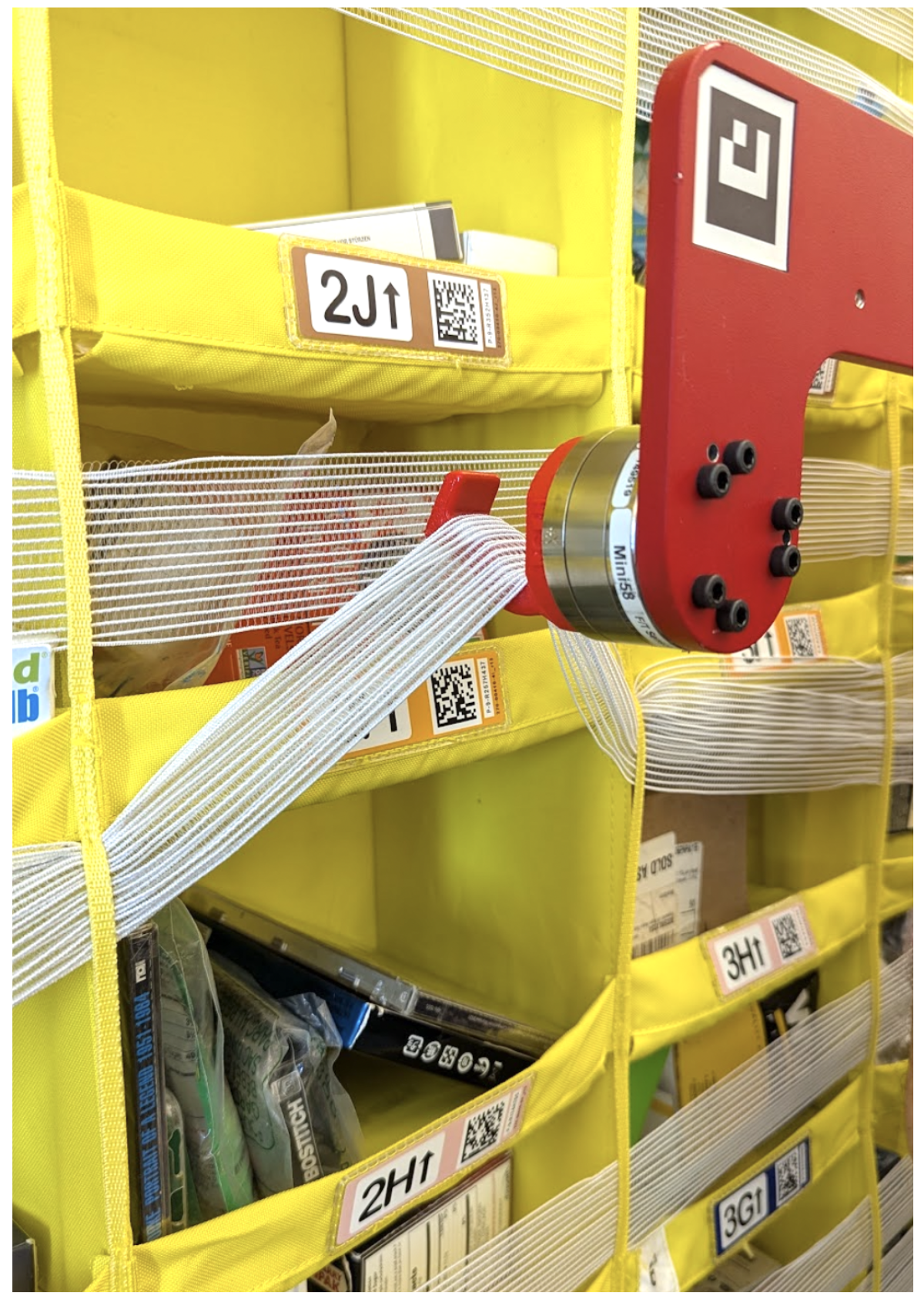
The stow algorithm
As a prelude to the stow operation, the stow robot’s EOAT receives an item from a conveyor belt. The width of the gripper opening is based on a computer vision system's inference of the item's dimensions.
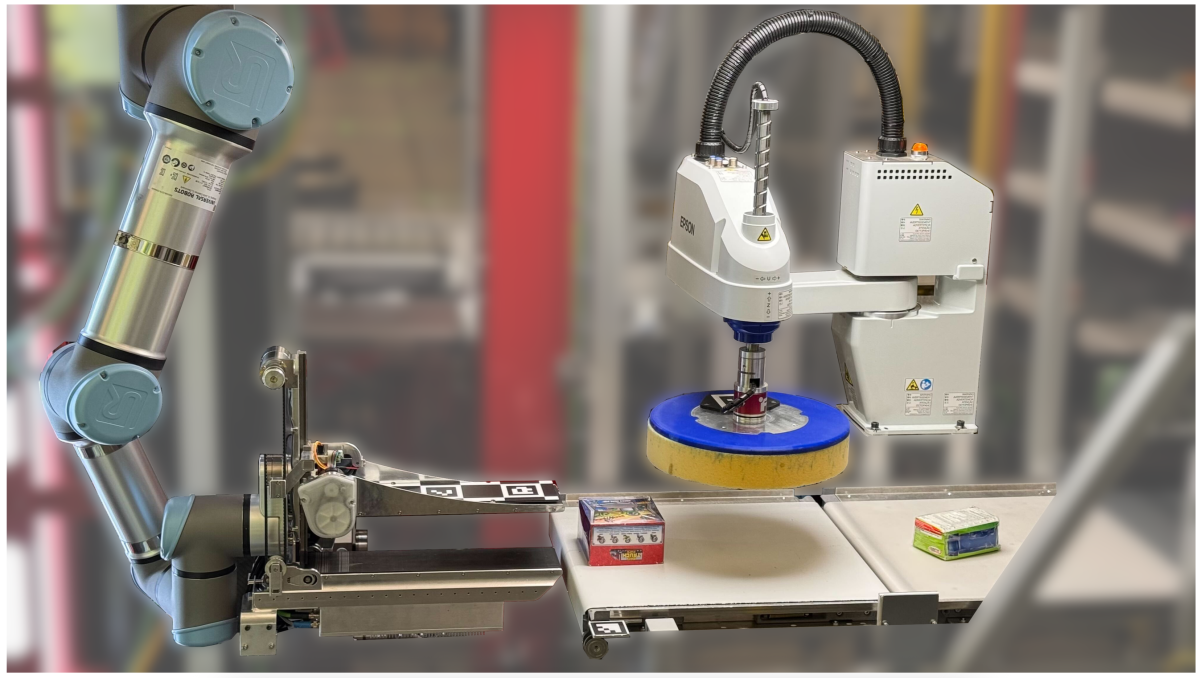
The stow system has three pairs of stereo cameras mounted on a tower, and their redundant stereo imaging allows it to build up a precise 3-D model of the pod and its contents.
At the beginning of a stow operation, the robot must identify a pod bin with enough space for the item to be stowed. A pod’s elastic bands can make imaging the items in each bin difficult, so the stow robot’s imaging algorithm was trained on synthetic bin images in which elastic bands were added by a generative-AI model.
The imaging algorithm uses three different deep-learning models to segment the bin image in three different ways: one model segments the elastic bands; one model segments the bins; and the third segments the objects inside the bands. These segments are then projected onto a three-dimensional point cloud captured by the stereo cameras to produce a composite 3-D segmentation of the bin.
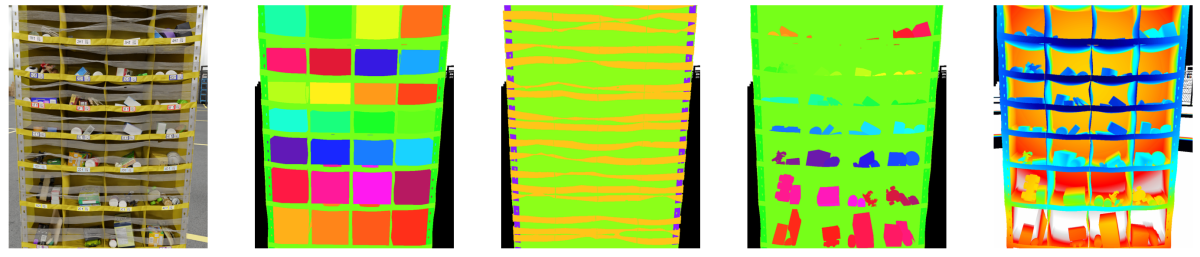
The stow algorithm then computes bounding boxes indicating the free space in each bin. If the sum of the free-space measurements for a particular bin is adequate for the item to be stowed, the algorithm selects the bin for insertion. If the bounding boxes are non-contiguous, the stow robot will push items to the side to free up space.
The algorithm uses convolution to identify space in a 2-D image in which an item can be inserted: that is, it steps through the image applying the same kernel — which represents the space necessary for an insertion — to successive blocks of pixels until it finds a match. It then projects the convolved 2-D image onto the 3-D model, and a machine learning model generates a set of affordances indicating where the item can be inserted and, if necessary, where the EOAT’s extensible blade can be inserted to move objects in the bin to the side.
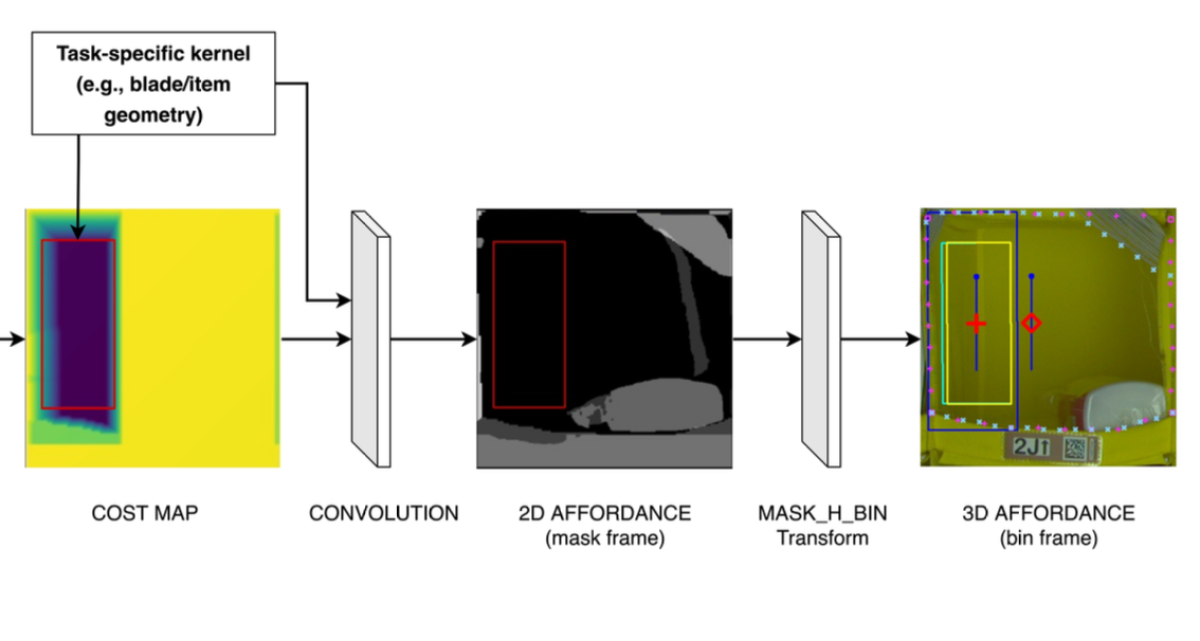
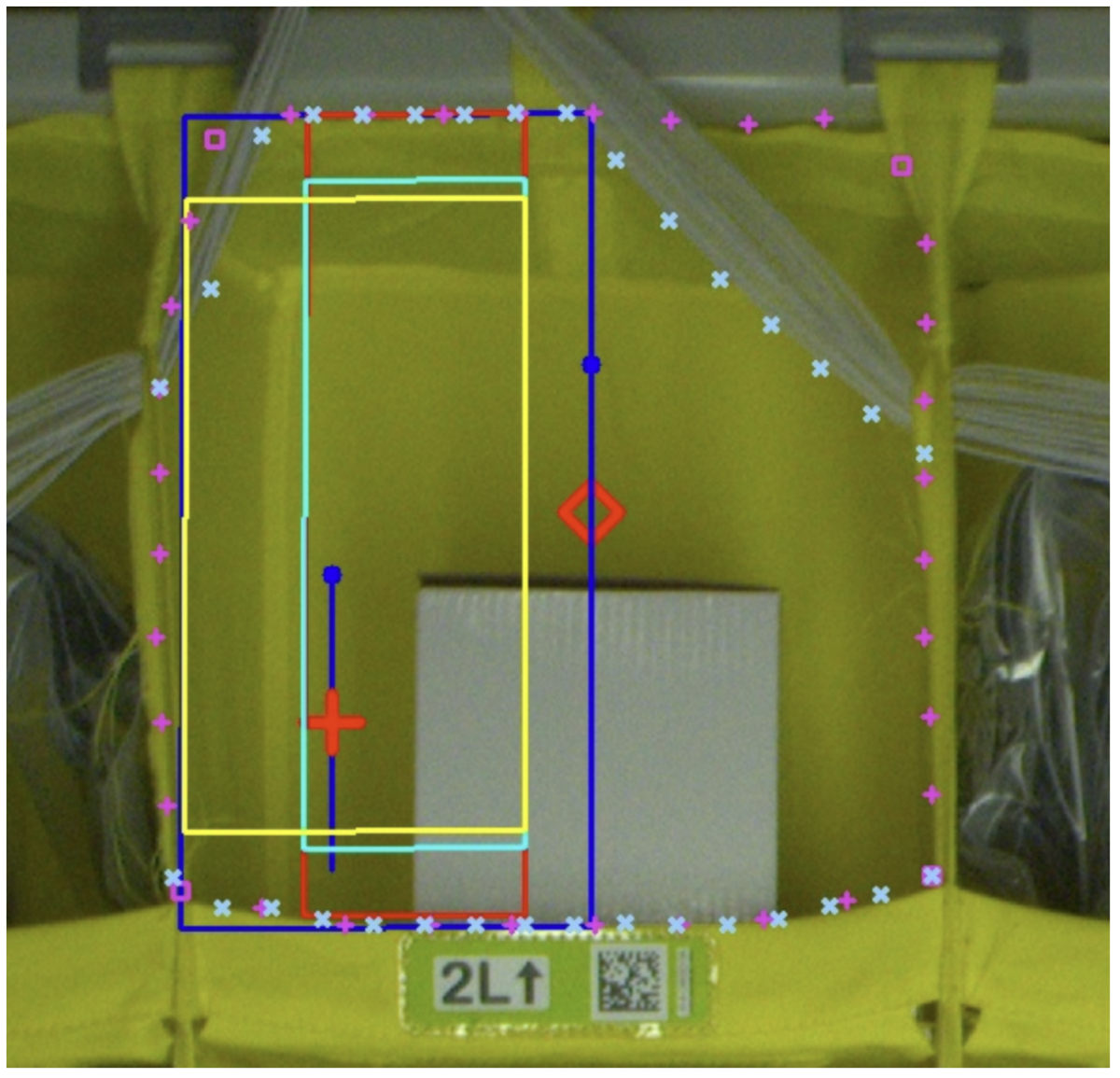
Based on the affordances, the stow algorithm then strings together a set of control primitives — such as approach, extend blade, sweep, and eject_item — to execute the stow. If necessary, the robot can insert the blade horizontally and rotate an object 90 degrees to clear space for an insertion.
“It's not just about creating a world model,” Parness explains. “It's not just about doing 3-D perception and saying, ‘Here's where everything is.’ Because we're interacting with the scene, we have to predict how that pile of objects will shift if we sweep them over to the side. And we have to think about like the physics of ‘If I collide with this T-shirt, is it going to be squishy, or is it going to be rigid?’ Or if I try and push on this bowling ball, am I going to have to use a lot of force? Versus a set of ping pong balls, where I'm not going to have to use a lot of force. That reasoning layer is also kind of unique.”
The pick algorithm
The first step in executing a pick operation is determining bin contents’ eligibility for robotic extraction: if a target object is obstructed by too many other objects in the bin, it’s passed to human pickers. The eligibility check is based on images captured by the FC’s existing imaging systems and augmented with metadata about the bins’ contents, which helps the imaging algorithm segment the bin contents.
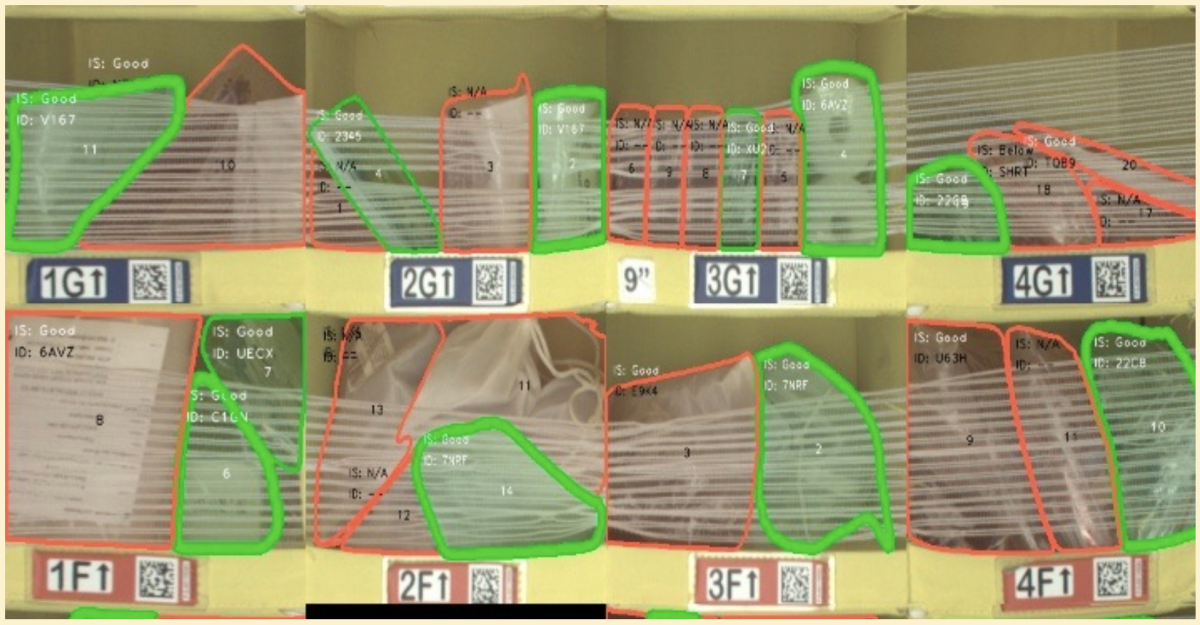
The pick operation itself uses the EOAT’s built-in camera, which uses structured light — an infrared pattern projected across the objects in the camera’s field of view — to gauge depth. Like the stow operation, the pick operation begins by segmenting the image, but the segmentation is performed by a single MaskDINO neural model. Parness’s team, however, added an extra layer to the MaskDINO model, which classifies the segmented objects into four categories: (1) not an item (e.g., elastic bands or metal bars), (2) an item in good status (not obstructed), (3) an item below others, or (4) an item blocked by others.
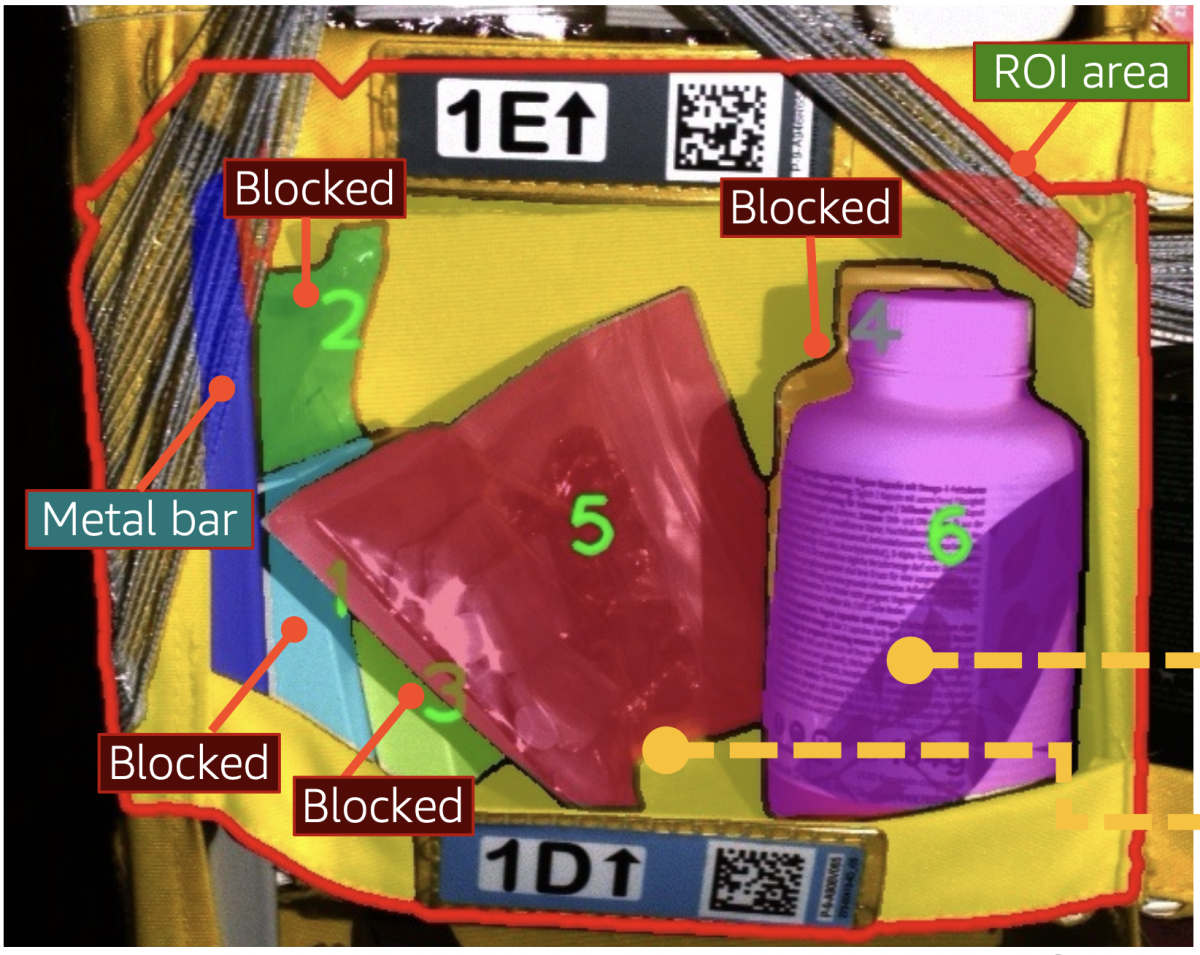
Like the stow algorithm, the pick algorithm projects the segmented image onto a point cloud indicating the depths of objects in the scene. The algorithm also uses a signed distance function to characterize the three-dimensional scene: free space at the front of a bin is represented with positive distance values, and occupied space behind a segmented surface is represented with negative distance values.
Next — without scanning barcodes — the algorithm must identify the object to be picked. Since the products in Amazon’s catalogue are constantly changing, and the lighting conditions under which objects are imaged can vary widely, the object identification compares target images on the fly to sample product images captured during other FC operations.
The product-matching model is trained through contrastive learning: it’s fed pairs of images, either same product photographed from different angles and under different lighting conditions, or two different products; it learns to minimize the distance between representations of the same object in the representational space and to maximize the distance between representations of different objects. It thus becomes a general-purpose product matcher.
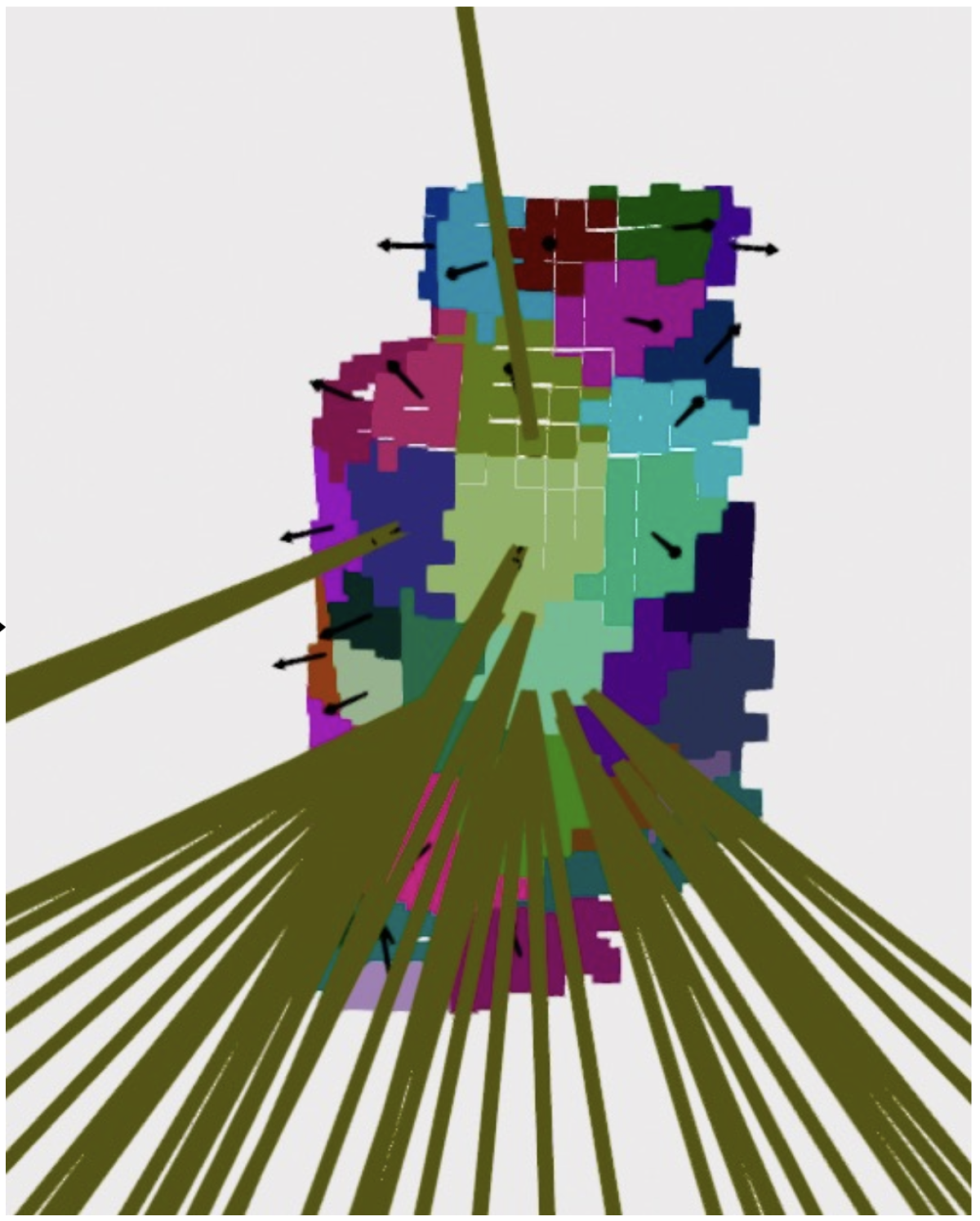
Using the 3-D composite, the algorithm identifies relatively flat surfaces of the target item that promise good adhesion points for the suction tool. Candidate surfaces are then ranked according to the signed distances of the regions around them, which indicate the likelihood of collisions during extraction.
Finally, the suction tool is deployed to affix itself to the highest-ranked candidate surface. During the extraction procedure, the suction pressure is monitored to ensure a secure hold, and the camera captures 10 low-res images per second to ensure that the extraction procedure hasn’t changed the geometry of the bin. If the initial pick point fails, the robot tries one of the other highly ranked candidates. In the event of too many failures, it passes the object on for human extraction.
“I really think of this as a new paradigm for robotic manipulation,” Parness says. “Getting out of the ‘I can only move through free space’ or ‘Touch the thing that's on the top of the pile’ to the new paradigm where I can handle all different kinds of items, and I can dig around and find the toy that's at the bottom of the toy chest, or I can handle groceries and pack groceries that are fragile in a bag. I think there's maybe 20 years of applications for this force-in-the-loop, high-contact style of manipulation.”
For more information about the Vulcan Pick and Stow robots, see the associated research papers: Pick | Stow.