Sometimes, good luck wears bad luck's clothing — and that was certainly the case in 2015 for the young electrical and computer engineer Supreeth Shashikumar, when his hunt for a PhD project came up empty. At the Georgia Institute of Technology, no professors were looking for students with his specialty — speech recognition and voice processing.
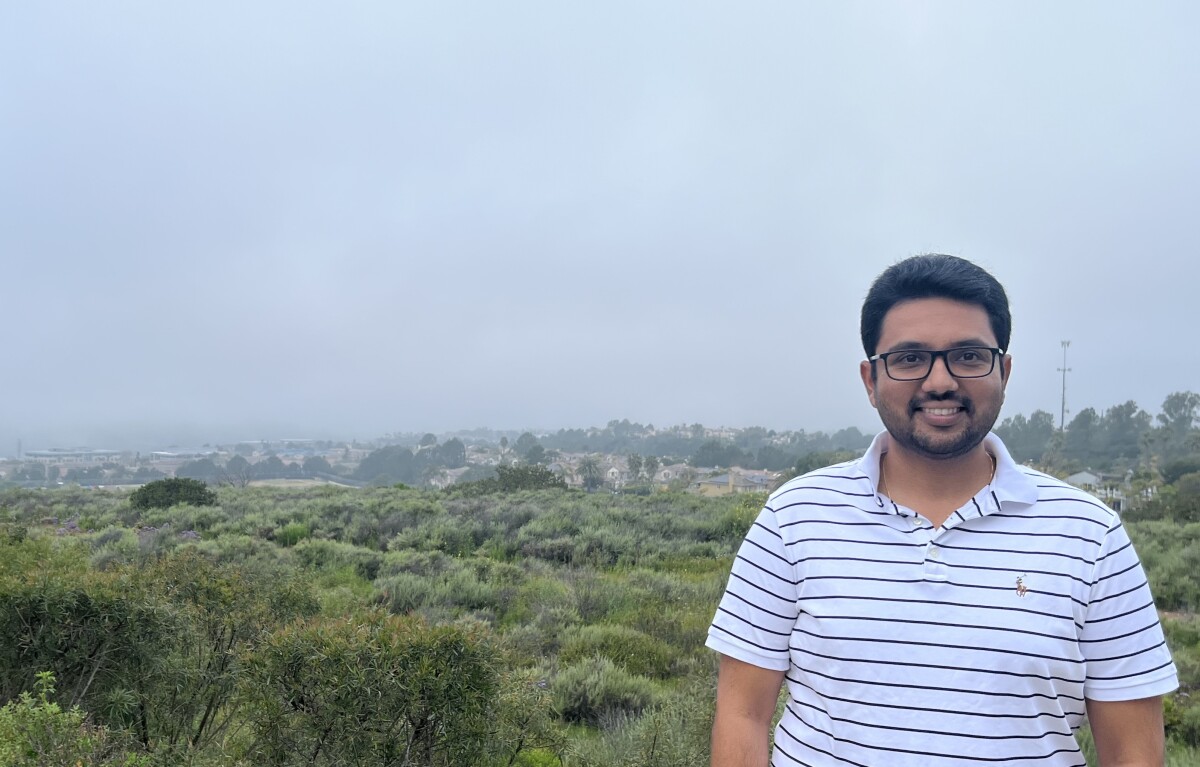
So Shashikumar decided to cast his net into a different field and came across a visionary mentor in Shamim Nemati, then an assistant professor in the Department of Biomedical Informatics at the Emory University in Atlanta, where he was also leading the Nemati Lab.
Today, Shashikumar and Nemati are continuing their collaboration at the University of California, San Diego (UCSD), and the medical technology they developed together, supported by an Amazon Research Award, is safeguarding and saving the lives of hospital patients. But let's rewind for a moment.
Back in 2015, when Shashikumar was still stymied in his search, he shifted his focus slightly from speech recognition to the somewhat similar field of biomedical signal processing. The fields overlap in that both are reliant on time series data, such as voice recordings or electrocardiograms.
Shashikumar saw that the Nemati Lab was pioneering the use of time series data held in hospital patients' electronic health records (EHRs) to develop early-warning systems that can aid clinicians by flagging patients who may be on the verge of sudden deterioration. Shashikumar found that to be a compelling idea, so he reached out to Nemati.
“It was a gamble, but it paid off,” Shashikumar says.
Taking on Shashikumar was an easy decision, says Nemati. “Georgia Tech produces some of the best engineers in the country. Add to that somebody who also enjoys bungee jumping and at the same time is extremely detail-oriented, and you’ll get a 10x engineer with a desire to push himself to the limits.” There they started on a multiyear journey toward the development of clinically actionable predictive models in healthcare. Shashikumar would later move with the Nemati Lab to its current home at UCSD.
The lab’s main focus is the onset of sepsis in hospital emergency departments (EDs), wards, and intensive-care units (ICUs). Sepsis is a sudden and life-threatening condition caused by an infection entering the bloodstream, triggering a catastrophic immune response that can lead to organ failure, septic shock, and death. It is a medical emergency that requires early and aggressive treatment with antibiotics. According to the US-based Sepsis Alliance, for every hour that treatment is delayed, the chance of sepsis moving through severe sepsis to septic shock and death rises by 4%-9%.
ED clinicians are constantly monitoring for signs of sepsis, such as fever and elevated heart rate or respiratory rate. When they suspect sepsis, they order lab tests to look for markers of organ damage. Thus detection, particularly early detection, is crucial.
Shashikumar was drawn to the fact that the Nemati Lab was focused on developing deployable technology. Many researchers take historical time-series patient data from single hospitals and create models to make predictions based on that data, but there is often a chasm between theory and practical deployment, due to the many challenges of working in the healthcare space.
“For us, whenever we pick a project, we are interested in how we can deploy a model into the real world, to do some good by making it clinically actionable,” says Shashikumar. “And, crucially, also make it generalizable.”
This generalizability of medical models is critical if machine learning is to realize its enormous potential benefit to patients.
“Generalizability is about ensuring that your claims about the performance of your model hold in other healthcare system settings,” says Nemati.
For example, say a machine learning model was trained to successfully predict the onset of sepsis in patients in hospital A, using data from that hospital. Could that model then be usefully applied to hospital B with different patient demographics, standards of care, and testing and monitoring procedures? And could it generalize again to hospitals C and D, too? It is an incredibly difficult challenge and one of the reasons for the chasm between research and implementation.
WUPERR
In 2022, in Nature Scientific Reports, Shashikumar and the Nemati team demonstrated that it was indeed possible, with a model called WUPERR (weight uncertainty propagation and episodic representation replay). The model was trained on the EHR data of more than 104,000 patients across four separate healthcare systems. The patient data included over 40 inputs, including ongoing vital signs such as blood pressure and pulse rate, lab test results such as lactate levels in the blood and white blood cell count, patient age, and comorbidities, such as cancer or liver failure.
The model overcame several big scientific and practical hurdles inherent to generalization across multiple hospitals: "catastrophic forgetting" and the necessity of keeping patient data confidential.
Catastrophic forgetting is a common problem with transfer learning. When a predictive model is successfully trained on one hospital (hospital A) and then transferred to the next (hospital B), the process will often involve fine-tuning the model on data from hospital B, as no two hospitals are the same. There's a risk, however, that the introduction of new hospital B data will lead the model to "forget" what it learned from hospital A's data.
In theory, one could keep the original model for hospital A and use the fine-tuned model for hospital B, and so on for hospitals C and D. However, not only is this approach impractical, but it also presents a daunting level of regulatory hurdles, according to Shashikumar. Having to deal with a growing number of different models, each of which must meet FDA evaluation and regulation, is simply not scalable.
WUPERR, however, tested a different solution using historical hospital data — a technique called "elastic weight consolidation". This approach echoes a concept found in cognitive neuroscience, according to Shashikumar.
"There are a bunch of neurons in your brain that are trained in the tasks you’ve learned,” he explains. “When you learn a new, similar task, you build on your previous experience — but you don't interfere with those neurons. Instead, you teach additional neurons the nuances of the new task."
With this approach — but with neurons replaced by adjustable model parameters — the team was able to maintain high accuracy in their sepsis predictions across the board with every new hospital added to the pool. By the end, the very accurate sepsis predictions for four hospitals were successfully produced by one model — an important advance.
You may now wonder: How could this model share fiercely protected patient data between separate hospitals? This is the second hurdle that WUPERR overcame, using a technique called “episodic representation replay.” In simple terms, this means that when the model was trained on hospital A's patient data, that data is passed through a neural network that strips away all patient identifiers and creates a representation of the data that is safe to share. The representations of the data are then shown to the model while training at the next hospital.
"I believe this was the first application of sharing neural-network representations from an older hospital with a new hospital in the context of sepsis prediction," says Shashikumar.
The result of all this is a single, manageable model that can generalize across a whole set of hospitals, with all the institutions involved benefiting from each other's patient data while never actually having access to it.
“There is beauty in generalizable knowledge and generalizable models, like a unified theory of everything,” says Nemati.
Things get real
Today the latest iteration of WUPERR is in live action in the ED of a UC San Diego Health hospital, providing clinicians with early warnings about patients predicted to develop sepsis in the next four hours. This version of WUPERR has also been augmented with, among other things, a statistical model that monitors its input data for quality, helping to reduce false alarms.
That’s important because false alarms are a big problem in sepsis detection. The hospital’s previous, less sophisticated system had a high rate of false alarms. Working with clinicians at the hospital, Shashikumar and his colleagues were able to tune WUPERR to predict 60% of all sepsis events. In the closely monitored environment of the ED, clinicians are expected to catch some portion of the sepsis cases with obvious signs and symptoms, and WUPERR provides a second pair of eyes to provide earlier warning and potentially catch additional cases of sepsis. What is critical to the clinicians is that false alarms, and the burdens they entail, remain low. While about half of WUPERR’s predictions were false alarms, that rate is relatively low, given the seriousness of sepsis.
Missed detections are also of great concern and are often attributable to patient complexity, inadequate monitoring, and low availability of data. Here, the team is applying active sensing to make timely recommendations for collecting sepsis-specific biomarkers in high-risk patients. The latest generation of the system combines false-alarm reduction with active sensing to achieve state-of-the-art performance.
The system has been in place for four months, with data collection ongoing. The clinicians in the ED have reported that, on average, the alarm is going off an hour or two earlier than when the doctors would have started to suspect an infection.
“They’re happy with that performance, particularly the lower false-alarm rate. It’s a very good validation of our work,” says Shashikumar. “But we still have a long way to go. In time, we want to extend this to other hospitals, intensive-care units, and hospital wards across the US and the world.”
The scaling up of this life-saving service is made easier by the fact that WUPERR is entirely cloud-based and hosted on Amazon Web Services.
“Using AWS services has been great for us," says Shashikumar. "Our sepsis software is running in real time in the hospital lab, and that’s mission-critical — it has to be up and running 100% of the time, without fail.” The team makes use of a wide range of AWS services, including autoscaling, load balancing, fault tolerance, and CloudWatch alarms.
Deploying the model in different locations is also greatly simplified. AWS provides HIPAA-compliant infrastructure, which is legally required to protect private health data transmitted to the cloud.
In fact, when the Nemati Lab moved to UC San Diego, they had to decide whether to buy their own in-house servers or move to the cloud. They moved the entirety of their computing services to AWS. “It has been super convenient,” says Shashikumar.
Last year, Nemati's team, including Shashikumar, co-founded Healcisio, a startup, as part of an effort to commercialize their model and ultimately receive FDA clearance, which will be essential for deploying the system to multiple hospitals in the US and abroad.
Meanwhile, they have great ambitions to improve the model. For now, it is limited to the time series data in EHRs. But the team’s current focus is on multimodal data, including wearable sensors, clinical notes, imaging, and more. They want their model to see everything a clinician has access to when they treat patients — all the contextual information — and additionally address “data deserts” via continuous monitoring of patients and active sensing.
Increasing the sensitivity of the model and reducing its false-alarm rate even further is the ultimate goal.
“At the end of the day, our focus is on building a model that can save as many lives as possible,” Shashikumar said. “I didn't get into healthcare out of passion, but it has become my passion.”