In the past few weeks, Amazon announced versions of Alexa in three new languages: Hindi, U.S. Spanish, and Brazilian Portuguese.
Like all new-language launches, these addressed the problem of how to bootstrap the machine learning models that interpret customer requests, without the ability to learn from customer interactions. At a high level, the solution is to use synthetic data. These three locales were the first to benefit from two new in-house tools, developed by the Alexa AI team, that produce higher-quality synthetic data more efficiently.
Each new locale has its own speech recognition model, which converts an acoustic speech signal into text. But interpreting that text — determining what the customer wants Alexa to do — is the job of Alexa’s natural-language-understanding (NLU) systems.
When a new-language version of Alexa is under development, training data for its NLU systems is scarce. Alexa feature teams will propose some canonical examples of customer requests in the new language, which we refer to as “golden utterances”; training data from existing locales can be translated by machine translation systems; crowd workers may be recruited to generate sample texts; and some data may come from Cleo, an Alexa skill that allows multilingual customers to help train new-language models by responding to voice prompts with open-form utterances.
Even when data from all these sources is available, however, it’s sometimes not enough to train a reliable NLU model. The new bootstrapping tools, from Alexa AI’s Applied Modeling and Data Science group, treat the available sample utterances as templates and generate new data by combining and varying those templates.
One of the tools, which uses a technique called grammar induction, analyzes a handful of golden utterances to learn general syntactic and semantic patterns. From those patterns, it produces a series of rewrite expressions that can generate thousands of new, similar sentences. The other tool, guided resampling, generates new sentences by recombining words and phrases from examples in the available data. Guided resampling concentrates on optimizing the volume and distribution of sentence types, to maximize the accuracy of the resulting NLU models.
Rules of Grammar
Grammars have been a tool in Alexa’s NLU toolkit since well before the first Echo device shipped. A grammar is a set of rewrite rules for varying basic template sentences through word insertions, deletions, and substitutions.
Below is a very simple grammar, which models requests to play either pop or rock music, with or without the modifiers “more” and “some”. Below the rules of the grammar is a diagram of a computational system (a finite-state transducer, or FST) that implements them.
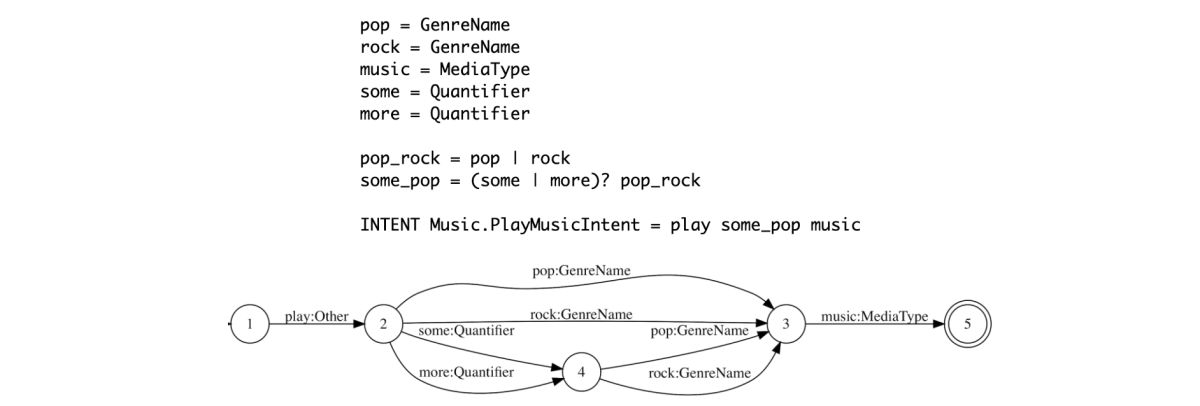
Given a list of, say, 50 golden utterances, a computational linguist could probably generate a representative grammar in a day, and it could be operationalized by the end of the following day. With the Applied Modeling and Data Science (AMDS) group’s grammar induction tool, that whole process takes seconds.
AMDS research scientists Ge Yu and Chris Hench and language engineer Zac Smith experimented with a neural network that learned to produce grammars from golden utterances. But they found that an alternative approach, called Bayesian model merging, offered similar performance with advantages in reproducibility and iteration speed.
The resulting system identifies linguistic patterns in lists of golden utterances and uses them to generate candidate rules for varying sentence templates. For instance, if two words (say, “pop” and “rock”) consistently occur in similar syntactic positions, but the phrasing around them varies, then one candidate rule will be that (in some defined contexts) “pop” and “rock” are interchangeable.
After exhaustively listing candidate rules, the system uses Bayesian probability to calculate which rule accounts for the most variance in the sample data, without overgeneralizing or introducing inconsistencies. That rule becomes an eligible variable in further iterations of the process, which recursively repeats until the grammar is optimized.
Crucially, the tool’s method for creating substitution rules allows it to take advantage of existing catalogues of frequently occurring terms or phrases. If, for instance, the golden utterances were sports related, and the grammar induction tool determined that the words “Celtics” and “Lakers” were interchangeable, it would also conclude that they were interchangeable with “Warriors”, “Spurs”, “Knicks”, and all the other names of NBA teams in a standard catalogue used by a variety of Alexa services.
From a list of 50 or 60 golden utterances, the grammar induction tool might extract 100-odd rules that can generate several thousand sentences of training data, all in a matter of seconds.
Safe Swaps
The guided-resampling tool also uses catalogues and existing examples to augment training data. Suppose that the available data contains the sentences “play Camila Cabello” and “can you play a song by Justin Bieber?”, which have been annotated to indicate that “Camila Cabello” and “Justin Bieber” are of the type ArtistName. In NLU parlance, ArtistName is a slot type, and “Camila Cabello” and “Justin Bieber” are slot values.
The guided-resampling tool generates additional training examples by swapping out slot values — producing, for instance, “play Justin Bieber” and “can you play a song by Camila Cabello?” Adding the vast Amazon Music databases of artist names and song titles to the mix produces many additional thousands of training sentences.
Blindly swapping slot values can lead to unintended consequences, so which slot values can be safely swapped? For example, in the sentences “play jazz music” and “read detective books”, both “jazz” and “detective” would be labeled with the slot type GenreName. But customers are unlikely to ask Alexa to play “detective music”, and unnatural training data would degrade the performance of the resulting NLU model.
AMDS’s Olga Golovneva, a research scientist, and Christopher DiPersio, a language engineer, used the Jaccard index — which measures the overlap between two sets — to evaluate pairwise similarity between slot contents in different types of requests. On that basis, they defined a threshold for valid slot mixing.
Quantifying Complexity
As there are many different ways to request music, another vital question is how many variations of each template to generate in order to produce realistic training data. One answer is simply to follow the data distributions from languages that Alexa already supports.
Comparing distributions of sentence types across languages requires representing customer requests in a more abstract form. We can encode a sentence like “play Camila Cabello” according to the word pattern other + ArtistName, where other represents the verb “play”, and ArtistName represents “Camila Cabello”. For “play ‘Havana’ by Camila Cabello”, the pattern would be other + SongName + other + ArtistName. To abstract away from syntactic differences between languages, we can condense this pattern further to other + ArtistName + SongName, which represents only the semantic concepts included in the request.
Given this level of abstraction, Golovneva and DiPersio investigated several alternative techniques for determining the semantic distributions of synthetic data.
Using Shannon entropy, which is a measure of uncertainty, Golovneva and DiPersio calculated the complexity of semantic sentence patterns, focusing on slots and their combinations. Entropy for semantic slots takes into consideration how many different values each slot might have, as well as how frequent each slot is in the data set overall. For example, the slot SongName occurs very frequently in music requests, and its potential values (different song titles) number in the millions; in contrast, GenreName also occurs frequently in music requests, but its set of possible values (music genres) is fairly small.
Customer requests to Alexa often include multiple slots (such as “play ‘Vogue’|SongName by Madonna|ArtistName” or “set a daily|RecurrenceType reminder to {walk the dog}|ReminderContent for {seven a. m.}|Time”), which increases the pattern complexity further.
In their experiments, Golovneva and DiPersio used the entropy measures from slot distributions in the data and the complexity of slot combinations to determine the optimal distribution of semantic patterns in synthetic training data. This results in proportionally larger training sets for more complex patterns than for less complex ones. NLU models trained on such data sets achieved higher performance than those trained on datasets which merely “borrowed” slot distributions from existing languages.
Alexa is always getting smarter, and these and other innovations from AMDS researchers help ensure the best experience possible when Alexa launches in a new locale.
Acknowledgments: Ge Yu, Chris Hench, Zac Smith, Olga Golovneva, Christopher DiPersio, Karolina Owczarzak, Sreekar Bhaviripudi, Andrew Turner