Today we announced the public beta launch of Alexa Conversations dialogue management. Alexa developers can now leverage a state-of-the-art dialogue manager powered by deep learning to create complex, nonlinear experiences — conversations that go well beyond today's typical one-shot interactions, such as "Alexa, what's the weather forecast for today?" or "Alexa, set a ten-minute pasta timer".
Alexa’s natural-language-understanding models classify requests according to domain, or the particular service that should handle the intent that the customer wants executed. The models also identify the slot types of the entities named in the requests, or the roles those entities play in fulfilling the request. In the request “Play ‘Rise Up’ by Andra Day”, the domain is Music, the intent is PlayMusic, and the names “Rise Up” and “Andra Day” fill the slots SongName and ArtistName.
Also at today's Alexa Live event, Nedim Fresko, vice president of Alexa Devices and Developers, announced that Amazon scientists have begun applying deep neural networks to custom skills and are seeing increases in accuracy. Read more here.
Natural conversations don’t follow these kinds of predetermined dialogue paths and often include anaphoric references (such as referring to a previously mentioned song by saying “play it”), contextual carryover of entities, customer revisions of requests, and many other types of interactions.
Alexa Conversations enables customers to interact with Alexa in a natural and conversational manner. At the same time, it relieves developers of the effort they would typically need to expend in authoring complex dialogue management rules, which are hard to maintain and often result in brittle customer experiences. Our dialogue augmentation algorithms and deep-learning models address the challenge of designing flexible and robust conversational experiences.
Dialogue management for Alexa Conversations is powered by two major science innovations: a dialogue simulator for data augmentation that generalizes a small number of sample dialogues provided by a developer into tens of thousands of annotated dialogues, and a conversations-first modeling architecture that leverages the generated dialogues to train deep-learning-based models to support dialogues beyond just the happy paths provided by the sample dialogues.
The Alexa Conversations dialogue simulator
Building high-performing deep-learning models requires large and diverse data sets, which are costly to acquire. With Alexa Conversations, the dialogue simulator automatically generates diversity from a few developer-provided sample dialogues that cover skill functionality, and it also generates difficult or uncommon exchanges that could occur.
The inputs to the dialogue simulator include developer application programming interfaces (APIs), slots and associated catalogues for slot values (e.g. city, state), and response templates (Alexa’s responses in different situations, such as requesting a slot value from the customer). These inputs together with their input arguments and output values define the skill-specific schema of actions and slots that the dialogue manager will predict.
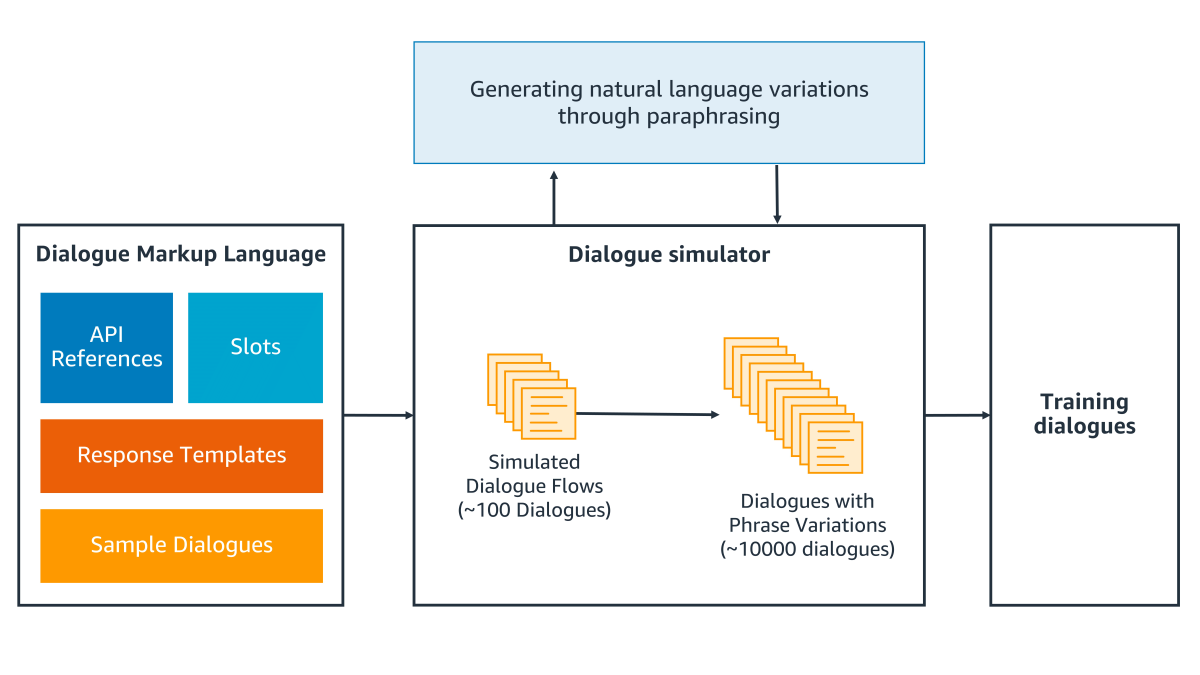
The dialogue simulator uses these inputs to generate additional sample dialogues in two steps.
In the first step, the simulator generates dialogue variations that represent different paths a conversation can take, such as different sequences of slot values and divergent paths that arise when a customer changes her mind.
More specifically, we conceive a conversation as a collaborative, goal-oriented interaction between two agents, a customer and Alexa. In this setting, the customer has a goal she wants to achieve, such as booking an airplane flight, and Alexa has access to resources, such as APIs for searching flight information or booking flights, that can help the customer reach her goal.
The simulated dialogues are generated through the interaction of two agent simulators, one for the customer, the other for Alexa. From the sample dialogues provided by the developer, the simulator first samples several plausible goals that customers interacting with the skill may want to achieve.
Conditioned on a sample goal, we generate synthetic interactions between the two simulator agents. The customer agent progressively reveals its goal to the Alexa agent, while the Alexa agent gathers the customer agent’s information, confirms information, and asks follow-up questions about missing information, guiding the interaction toward goal completion.
In the second step, the simulator injects language variations into the dialogue paths. The variations include alternate expressions of the same customer intention, such as “recommend me a movie” versus “I want to watch a movie”. Some of these alternatives are provided by the sample conversations and Alexa response templates, while others are generated through paraphrasing.
The variations also include alternate slot values (such as “Andra Day” or “Alicia Keys” for the slot ArtistName), which are sampled from slot catalogues provided by the developer. Through these two steps, the simulator generates tens of thousands of annotated dialogue examples that are used for training the conversational models.
The Alexa Conversations modeling architecture
A natural conversational experience could follow any one of a wide range of nonlinear dialogue patterns. Our conversations-first modeling architecture leverages dialogue-simulator and conversational-modeling components to support dialogue patterns that include carryover of entities, anaphora, confirmation of slots and APIs, and proactively offering related functionality, as well as robust support for a customer changing her mind midway through a conversation.
We follow an end-to-end dialogue-modeling approach, where the models take into account the current customer utterance and context from the entire conversation history to predict the optimal next actions for Alexa. Those actions might include calling a developer-provided API to retrieve information and relaying that information to the customer; asking for more information from the customer; or any number of other possibilities.
The modeling architecture is built using state-of-the-art deep-learning technology and consists of three models: a named-entity-recognition (NER) model, an action prediction (AP) model, and an argument-filling (AF) model. The models are built by combining supervised training techniques on the annotated synthetic dialogues generated by the dialogue simulator and unsupervised pretraining of large Transformer-based components on text corpora.
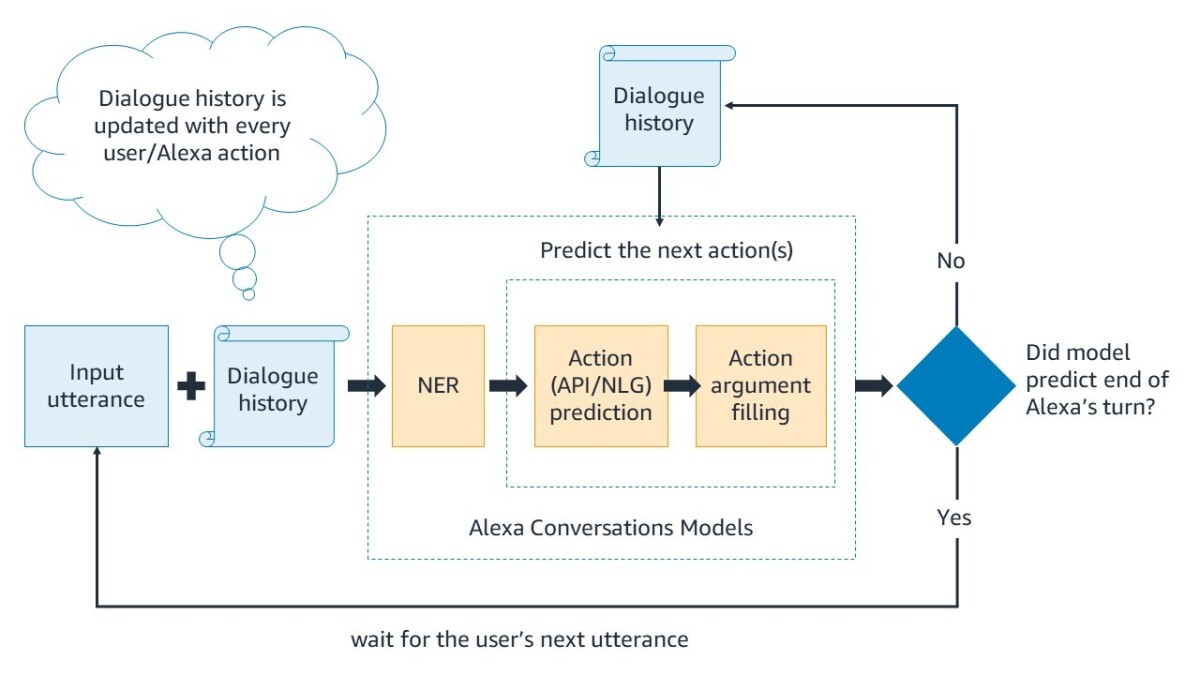
First, the NER model identifies slots in each of the customer utterances, selecting from slots the developer defined as part of the build-time assets (date, city, etc.). For example, for the request “search for flights to Seattle tomorrow”, the NER model will identify “Seattle” as a city slot and “tomorrow” as a date slot.
The NER model is a sequence-tagging model built using a bidirectional LSTM layer on top of a Transformer-based pretrained sentence encoder. In addition to the current sentence, NER also takes dialogue context as input, which is encoded through a hierarchical LSTM architecture that captures the conversational history, including past slots and Alexa actions.
Next, the AP model predicts the optimal next action for Alexa to take, such as calling an API or responding to the customer to either elicit more information or complete a request. The action space is defined by the APIs and Alexa response templates that the developer provides during the skill-authoring process.
The AP model is a classification model that, like the NER model, uses a hierarchical LSTM architecture to encode the current utterance and past dialogue context, which ultimately passes to a feed-forward network to generate the action prediction.
Finally, the AF model fills in the argument values for the API and response templates by looking at the entire dialogue for context. Using an attention-based pointing mechanism over the dialogue context, the AF model selects compatible slots from all slot values that the NER model recognized earlier.
For example, suppose slot values “Seattle” and “tomorrow” exist in the dialogue context for city and date slots respectively, and the AP model predicted the SearchFlight API as the optimal next action. The AF model will fill in the API arguments with the appropriate values, generating a complete API call: SearchFlight (city=“Seattle”, date="tomorrow").
The AP and AF models may also predict and generate more than one action after a customer utterance. For example, they may decide to first call an API to retrieve flight information and then call an Alexa response template to communicate this information to the customer. Therefore, the AP and AF models can make sequential predictions of actions, including the decision to stop predicting more actions and wait for the next customer request.
The finer points
Consistency check logic ensures that the resulting predictions are all valid actions, consistent with developer-provided information about their APIs. For example, the system would not generate an API call with an empty input argument, if that input argument is required by the developer.
The inputs include the entire dialogue history, as well as the latest customer request, and the resulting model predictions are contextual, relevant, and not repetitive. For example, if a customer has already provided the date of a trip while searching for a flight, Alexa will not ask for the date when booking the flight. Instead, the date provided earlier will contextually carry over and pass to the appropriate API.
We leveraged large pretrained Transformer components (BERT) that encode current and past requests in the conversation. To ensure state-of-the-art model build-time and runtime latency, we performed inference architecture optimizations such as accelerating embedding computation on GPUs, implementing efficient caching, and leveraging both data- and model-level parallelism.
We are excited about the advances that enable Alexa developers to build flexible and robust conversational experiences that allow customers to have natural interactions with their devices. Developers interested in learning more about the "how" of building these conversational experiences should read our accompanying developer blog.
For more information about the technical advances behind Alexa Conversations, at right are relevant publications related to our work in dialogue systems, dialogue state tracking, and data augmentation.
Acknowledgments: The entire Alexa Conversations team for making the innovations highlighted here possible.