Cedar is a new authorization-policy language used by the Amazon Verified Permissions and AWS Verified Access managed services, and we recently released it publicly. Using Cedar, developers can write policies that specify fine-grained permissions for their applications. The applications then authorize access requests by calling Cedar’s authorization engine. Because Cedar policies are separate from application code, they can be independently authored, updated, analyzed, and audited.
We want to assure developers that Cedar’s authorization decisions will be correct. To provide that assurance, we follow a two-part process we call verification-guided development when we’re working on Cedar. First, we use automated reasoning to prove important correctness properties about formal models of Cedar’s components. Second, we use differential random testing to show that the models match the production code. In this blog post we present an overview of verification-guided development for Cedar.
A primer on Cedar
Cedar is a language for writing and enforcing authorization policies for custom applications. Cedar policies are expressed in syntax resembling natural language. They define who (the principal) can do what (the action) on what target (the resource) under which conditions (when)?
To see how Cedar works, consider a simple application, TinyTodo, designed for managing task lists. TinyTodo uses Cedar to control who can do what. Here is one of TinyTodo’s policies:
// policy 1 permit(principal, action, resource) when { resource has owner && resource.owner == principal };
This policy states that any principal (a TinyTodo User) can perform any action on any resource (a TinyTodo List) as long as the resource’s creator, defined by its owner attribute, matches the requesting principal. Here’s another TinyTodo Cedar policy:
// policy 2 permit ( principal, action == Action::"GetList", resource ) when { principal in resource.editors || principal in resource.readers };
This policy states that any principal can read the contents of a task list (Action::"GetList") if that principal is in either the list’s readers group or its editors group. Here is a third policy:
// policy 3 forbid ( principal in Team::"interns", action == Action::"CreateList", resource == Application::"TinyTodo" );
This policy states that any principal who is an intern (in Team::"interns") is forbidden from creating a new task list (Action::"CreateList") using TinyTodo (Application::"TinyTodo").
When the application needs to enforce access, as when a user of TinyTodo issues a command, it only needs to make a corresponding request to the Cedar authorization engine. The authorization engine evaluates the request in light of the Cedar policies and relevant application data. If it returns decision Allow, TinyTodo can proceed with the command. If it returns decision Deny, TinyTodo can report that the command is not permitted.
How do we build Cedar to be trustworthy?
Our work on Cedar uses a process we call verification-guided development to ensure that Cedar’s authorization engine makes the correct decisions. The process has two parts. First, we model Cedar’s authorization engine and validator in the Dafny verification-aware programming language. With Dafny, you can write code, and you can specify properties about what the code is meant to do under all circumstances. Using Dafny’s built-in automated-reasoning capabilities we have proved that the code satisfies a variety of safety and security properties.
Second, we use differential random testing (DRT) to confirm that Cedar’s production implementation, written in Rust, matches the Dafny model’s behavior. We generate millions of diverse inputs and feed them to both the Dafny model and the production code. If both versions always produce the same output, we have a high degree of confidence that the implementation matches the model.
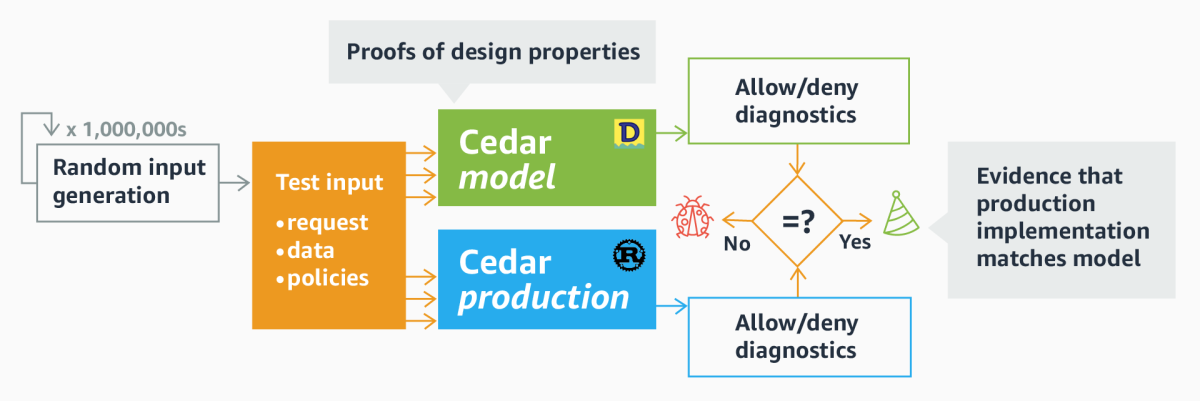
Proving properties about Cedar authorization
Cedar’s authorization algorithm was designed to be secure by default, as exemplified by the following two properties:
- explicit permit — permission is granted only by individual permit policies and is not gained by error or default;
- forbid overrides permit — any applicable forbid policy always denies access, even if there is a permit policy that allows it.
With these properties, sets of policies are easier to understand. Policy authors know that permit policies are the only way access is granted, and forbid policies decline access regardless of whether it is explicitly permitted.
Given an authorization request, the Cedar authorization engine takes each Cedar policy and evaluates it after substituting the application request parameters into the principal, action and resource variables. For example, for the request principal= User::”Alice”, action=Action::”GetList”, and resource=List::”AliceList”, substituting for the variables in policy 1 would produce the expression List::”AliceList” has owner && List::”AliceList”.owner == User::”Alice”. If this expression evaluates to true, we say the request satisfies the policy. The authorization engine collects the satisfied forbid and permit policies into distinct sets and then makes its decision.
We model the authorization engine as a Dafny function and use Dafny’s automated-reasoning capabilities to state and prove the explicit-permit and forbid-overrides-permit properties. To see how this helps uncover mistakes, let’s consider a buggy version of the authorization engine:
function method isAuthorized(): Response { // BUGGY VERSION var f := forbids(); var p := permits(); if f != {} then Response(Deny, f) else Response(Allow, p) }
The logic states that if any forbid policy is applicable (set f is not the empty set {}), the result should be Deny, thus overriding any applicable permit policies (in set p). Otherwise, the result is Allow. While this logic correctly reflects the desired forbid-overrides-permit property, it does not correctly capture explicit permit. Just because there are no applicable forbid policies doesn’t mean there are any applicable permit policies. We can see this by specifying and attempting to prove explicit permit in Dafny:
// A request is explicitly permitted when a permit policy is satisfied predicate IsExplicitlyPermitted(request: Request, store: Store) { exists p :: p in store.policies.policies.Keys && store.policies.policies[p].effect == Permit && Authorizer(request, store).satisfied(p) }
lemma AllowedIfExplicitlyPermitted(request: Request, store: Store) ensures // A request is allowed if it is explicitly permitted (Authorizer(request, store).isAuthorized().decision == Allow) ==> IsExplicitlyPermitted(request, store) { ... }
A Dafny predicate is a function that takes arguments and returns a logical condition, and a Dafny lemma is a property to be proved. The IsExplicitlyPermitted predicate defines the condition that there is an applicable permit policy for the given request. The AllowedIfExplicitlyPermitted lemma states that a decision of Allow necessarily means the request was explicitly permitted. This lemma does not hold for the isAuthorized definition above; Dafny complains that A postcondition might not hold on this return path and points to the ensures clause.
Here is the corrected code:
function method isAuthorized(): Response { var f := forbids(); var p := permits(); if f == {} && p != {} then Response(Allow, p) else Response(Deny, f) }
Now a response is Allow only if there are no applicable forbid policies, and there is at least one applicable permit policy. With this change, Dafny automatically proves AllowedIfExplicitlyPermitted. It also proves forbid overrides permit (not shown).
We have used the Cedar Dafny models to prove a variety of properties. Our most significant proof is that the Cedar validator, which confirms that Cedar policies are consistent with the application’s data model, is sound: if the validator accepts a policy, evaluating the policy should never result in certain classes of error. When carrying out this proof in Dafny, we found a number of subtle bugs in the validator’s design that we were able to correct.
We note that Dafny models are useful not just for automated reasoning but for manual reasoning, too. The Dafny code is much easier to read than the Rust implementation. As one measure of this, at the time of this writing the Dafny model for the authorizer has about one-sixth as many lines of code as the production code. Both Cedar users and tool implementers can refer to the Dafny models to quickly understand precise details about how Cedar works.
Differential random testing
Once we have proved properties about the Cedar Dafny model, we want to provide evidence that they hold for the production code, too, which we can do by using DRT to show that the model and the production code behave the same. Using the cargo fuzz random-testing framework, we generate millions of inputs — access requests, accompanying data, and policies — and send them to both the Dafny model engine and the Rust production engine. If the two versions agree on the decision, then all is well. If they disagree, then we have found a bug.
The main challenge with using DRT effectively is to ensure the necessary code coverage by generating useful and diverse inputs. Randomly generated policies are unlikely to mention the same groups and attributes chosen in randomly generated requests and data. As a result, pure random generation will miss a lot of core evaluation logic and overindex on error-handling code. To resolve this, we wrote several input generators, including ones that take care to generate policies, data, and requests that are consistent with one another, while also producing policies that use Cedar’s key language constructs. As of this writing, we run DRT for six hours nightly and execute on the order of 100 million total tests.
The use of DRT during Cedar’s development has discovered corner cases where there were discrepancies between the model and the production code, making it an important tool in our toolkit. For example, there was a bug in a Rust package we were using for IP address operations; the Dafny model exposed an issue in how the package was parsing IP addresses. Since the bug is in an external package, we fixed the problem within our code while we wait for the upstream fix. We also found subtle bugs in the Cedar policy parser, in how the authorizer handles missing application data, and how namespace prefixes on application data (e.g., TinyTodo::List::”AliceList”) are interpreted.
Learn more
In this post we have discussed the verification-guided development process we have followed for the Cedar authorization policy language. In this process, we model Cedar language components in the Dafny programming language and use Dafny’s automated-reasoning capabilities to prove properties about them. We check that the Cedar production code matches the Dafny model through differential random testing. This process has revealed several interesting bugs during development and has given us greater confidence that Cedar’s authorization engine makes correct decisions.
To learn more, you can check out the Cedar Dafny models and differential-testing code on GitHub. You can also learn more about Dafny on the Dafny website and the Cedar service on the Cedar website.