This week, Amazon Science added automated reasoning to its list of research areas. We made this change because of the impact that automated reasoning is having here at Amazon. For example, Amazon Web Services’ customers now have direct access to automated-reasoning-based features such as IAM Access Analyzer, S3 Block Public Access, or VPC Reachability Analyzer. We also see Amazon development teams integrating automated-reasoning tools into their development processes, raising the bar on the security, durability, availability, and quality of our products.
The goal of this article is to provide a gentle introduction to automated reasoning for the industry professional who knows nothing about the area but is curious to learn more. All you will need to make sense of this article is to be able to read a few small C and Python code fragments. I will refer to a few specialist concepts along the way, but only with the goal of introducing them in an informal manner. I close with links to some of our favorite publicly available tools, videos, books, and articles for those looking to go more in-depth.
Let’s start with a simple example. Consider the following C function:
bool f(unsigned int x, unsigned int y) { return (x+y == y+x); }
Take a few moments to answer the question “Could f ever return false?” This is not a trick question: I’ve purposefully used a simple example to make a point.
To check the answer with exhaustive testing, we could try executing the following doubly nested test loop, which calls f on all possible pairs of values of the type unsigned int:
#include<stdio.h> #include<stdbool.h> #include<limits.h> bool f(unsigned int x, unsigned int y) { return (x+y == y+x); } void main() { for (unsigned int x=0;1;x++) { for (unsigned int y=0;1;y++) { if (!f(x,y)) printf("Error!\n"); if (y==UINT_MAX) break; } if (x==UINT_MAX) break; } }
Unfortunately, even on modern hardware, this doubly nested loop will run for a very long time. I compiled it and ran it on a 2.6 GHz Intel processor for over 48 hours before giving up.
Why does testing take so long? Because UINT_MAX is typically 4,294,967,295, there are 18,446,744,065,119,617,025 separate f calls to consider. On my 2.6 GHz machine, the compiled test loop called f approximately 430 million times a second. But to test all 18 quintillion cases at this performance, we would need over 1,360 years.
When we show the above code to industry professionals, they almost immediately work out that f can't return false as long as the underlying compiler/interpreter and hardware are correct. How do they do that? They reason about it. They remember from their school days that x + y can be rewritten as y + x and conclude that f always returns true.
An automated reasoning tool does this work for us: it attempts to answer questions about a program (or a logic formula) by using known techniques from mathematics. In this case, the tool would use algebra to deduce that x + y == y + x can be replaced with the simple expression true.
Automated-reasoning tools can be incredibly fast, even when the domains are infinite (e.g., unbounded mathematical integers rather than finite C ints). Unfortunately, the tools may answer “Don’t know” in some instances. We'll see a famous example of that below.
The science of automated reasoning is essentially focused on driving the frequency of these “Don’t know” answers down as far as possible: the less often the tools report "Don't know" (or time out while trying), the more useful they are.
Today’s tools are able to give answers for programs and queries where yesterday’s tools could not. Tomorrow’s tools will be even more powerful. We are seeing rapid progress in this field, which is why at Amazon, we are increasingly getting so much value from it. In fact, we see automated reasoning forming its own Amazon-style virtuous cycle, where more input problems to our tools drive improvements to the tools, which encourages more use of the tools.
A slightly more complex example. Now that we know the rough outlines of what automated reasoning is, the next small example gives a slightly more realistic taste of the sort of complexity that the tools are managing for us.
void g(int x, int y) { if (y > 0) while (x > y) x = x - y; }
Or, alternatively, consider a similar Python program over unbounded integers:
def g(x, y): assert isinstance(x, int) and isinstance(y, int) if y > 0: while x > y: x = x - y
Try to answer this question: “Does g always eventually return control back to its caller?”
When we show this program to industry professionals, they usually figure out the right answer quickly. A few, especially those who are aware of results in theoretical computer science, sometimes mistakenly think that we can't answer this question, with the rationale “This is an example of the halting problem, which has been proved insoluble”. In fact, we can reason about the halting behavior for specific programs, including this one. We’ll talk more about that later.
Here’s the reasoning that most industry professionals use when looking at this problem:
- In the case where y is not positive, execution jumps to the end of the function g. That’s the easy case.
- If, in every iteration of the loop, the value of the variable x decreases, then eventually, the loop condition x > y will fail, and the end of g will be reached.
- The value of x always decreases only if y is always positive, because only then does the update to x (i.e., x = x - y) decrease x. But y’s positivity is established by the conditional expression, so x always decreases.
The experienced programmer will usually worry about underflow in the x = x - y command of the C program but will then notice that x > y before the update to x and thus cannot underflow.
If you carried out the three steps above yourself, you now have a very intuitive view of the type of thinking an automated-reasoning tool is performing on our behalf when reasoning about a computer program. There are many nitty-gritty details that the tools have to face (e.g., heaps, stacks, strings, pointer arithmetic, recursion, concurrency, callbacks, etc.), but there’s also decades of research papers on techniques for handling these and other topics, along with various practical tools that put these ideas to work.
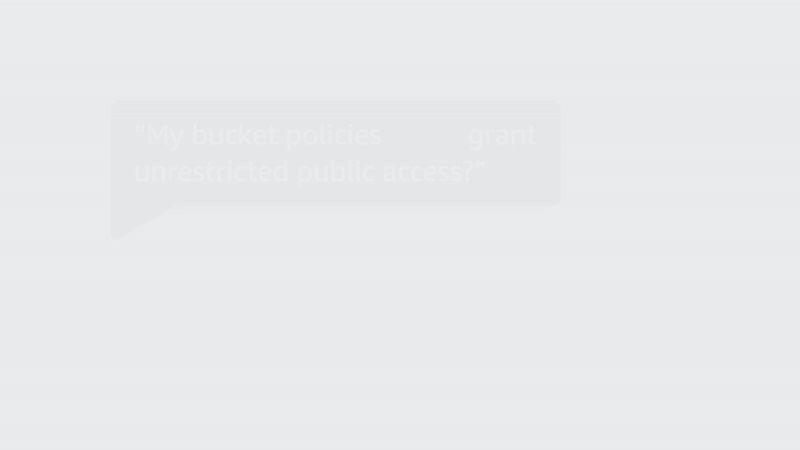
The main takeaway is that automated-reasoning tools are usually working through the three steps above on our behalf: Item 1 is reasoning about the program’s control structure. Item 2 is reasoning about what is eventually true within the program. Item 3 is reasoning about what is always true in the program.
Note that configuration artifacts such as AWS resource policies, VPC network descriptions, or even makefiles can be thought of as code. This viewpoint allows us to use the same techniques we use to reason about C or Python code to answer questions about the interpretation of configurations. It’s this insight that gives us tools like IAM Access Analyzer or VPC Reachability Analyzer.
An end to testing?
As we saw above when looking at f and g, automated reasoning can be dramatically faster than exhaustive testing. With tools available today, we can show properties of f or g in milliseconds, rather than waiting lifetimes with exhaustive testing.
Can we throw away our testing tools now and just move to automated reasoning? Not quite. Yes, we can dramatically reduce our dependency on testing, but we will not be completely eliminating it any time soon, if ever. Consider our first example:
bool f(unsigned int x, unsigned int y) { return (x + y == y + x); }
Recall the worry that a buggy compiler or microprocessor could in fact cause an executable program constructed from this source code to return false. We might also need to worry about the language runtime. For example, the C math library or the Python garbage collector might have bugs that cause a program to misbehave.
What’s interesting about testing, and something we often forget, is that it’s doing much more than just telling us about the C or Python source code. It’s also testing the compiler, the runtime, the interpreter, the microprocessor, etc. A test failure could be rooted in any of those tools in the stack.
Automated reasoning, in contrast, is usually applied to just one layer of that stack — the source code itself, or sometimes the compiler or the microprocessor. What we find so valuable about reasoning is it allows us to clearly define both what we do know and what we do not know about the layer under inspection.
Furthermore, the models of the surrounding environment (e.g., the compiler or the procedure calling our procedure) used by the automated-reasoning tool make our assumptions very precise. Separating the layers of the computational stack helps make better use of our time, energy, and money and the capabilities of the tools today and tomorrow.
Unfortunately, we will almost always need to make assumptions about something when using automated reasoning — for example, the principles of physics that govern our silicon chips. Thus, testing will never be fully replaced. We will want to perform end-to-end testing to try and validate our assumptions as best we can.
An impossible program
I previously mentioned that automated-reasoning tools sometimes return “Don’t know” rather than “yes” or “no”. They also sometimes run forever (or time out), thus never returning an answer. Let’s look at the famous "halting problem" program, in which we know tools cannot return “yes” or “no”.
Imagine that we have an automated-reasoning API, called terminates, that returns “yes” if a C function always terminates or “no” when the function could execute forever. As an example, we could build such an API using the tool described here (shameless self-promotion of author’s previous work). To get the idea of what a termination tool can do for us, consider two basic C functions, g (from above),
void g(int x, int y) { if (y > 0) while (x > y) x = x - y; }
and g2:
void g2(int x, int y) { while (x > y) x = x - y; }
For the reasons we have already discussed, the function g always returns control back to its caller, so terminates(g) should return true. Meanwhile, terminates(g2) should return false because, for example, g2(5, 0) will never terminate.
Now comes the difficult function. Consider h:
void h() { if terminates(h) while(1){} }
Notice that it's recursive. What’s the right answer for terminates(h)? The answer cannot be "yes". It also cannot be "no". Why?
Imagine that terminates(h) were to return "yes". If you read the code of h, you’ll see that in this case, the function does not terminate because of the conditional statement in the code of h that will execute the infinite loop while(1){}. Thus, in this case, the terminates(h) answer would be wrong, because h is defined recursively, calling terminates on itself.
Similarly, if terminates(h) were to return "no", then h would in fact terminate and return control to its caller, because the if case of the conditional statement is not met, and there is no else branch. Again, the answer would be wrong. This is why the “Don’t know” answer is actually unavoidable in this case.
The program h is a variation of examples given in Turing’s famous 1936 paper on decidability and Gödel’s incompleteness theorems from 1931. These papers tell us that problems like the halting problem cannot be “solved”, if by“solved” we mean that the solution procedure itself always terminates and answers either “yes” or “no” but never “Don’t know”. But that is not the definition of “solved” that many of us have in mind. For many of us, a tool that sometimes times out or occasionally returns “Don’t know” but, when it gives an answer, always gives the right answer is good enough.
This problem is analogous to airline travel: we know it’s not 100% safe, because crashes have happened in the past, and we are sure that they will happen in the future. But when you land safely, you know it worked that time. The goal of the airline industry is to reduce failure as much as possible, even though it’s in principle unavoidable.
To put that in the context of automated reasoning: for some programs, like h, we can never improve the tool enough to replace the "Don't know" answer. But there are many other cases where today's tools answer "Don't know", but future tools may be able to answer "yes" or "no". The modern scientific challenge for automated-reasoning subject-matter experts is to get the practical tools to return “yes” or “no” as often as possible. As an example of current work, check out CMU professor and Amazon scholar Marijn Heule and his quest to solve the Collatz termination problem.
Another thing to keep in mind is that automated-reasoning tools are regularly trying to solve “intractable” problems, e.g., problems in the NP complexity class. Here, the same thinking applies that we saw in the case of the halting problem: automated-reasoning tools have powerful heuristics that often work around the intractability problem for specific cases, but those heuristics can (and sometimes do) fail, resulting in “Don’t know” answers or impractically long execution time. The science is to improve the heuristics to minimize that problem.
Nomenclature
A host of names are used in the scientific literature to describe interrelated topics, of which automated reasoning is just one. Here’s a quick glossary:
- A logic is a formal and mechanical system for defining what is true and untrue. Examples: propositional logic or first-order logic.
- A theorem is a true statement in logic. Example: the four-color theorem.
- A proof is a valid argument in logic of a theorem. Example: Gonthier's proof of the four-color theorem.
- A mechanical theorem prover is a semi-automated-reasoning tool that checks a machine-readable expression of a proof often written down by a human. These tools often require human guidance. Example: HOL-light, from Amazon researcher John Harrison.
- Formal verification is the use of theorem proving when applied to models of computer systems to prove desired properties of the systems. Example: the CompCert verified C compiler.
- Formal methods is the broadest term, meaning simply the use of logic to reason formally about models of systems.
- Automated reasoning focuses on the automation of formal methods.
- A semi-automated-reasoning tool is one that requires hints from the user but still finds valid proofs in logic.
As you can see, we have a choice of monikers when working in this space. At Amazon, we’ve chosen to use automated reasoning, as we think it best captures our ambition for automation and scale. In practice, some of our internal teams use both automated and semi-automated reasoning tools, because the scientists we've hired can often get semi-automated reasoning tools to succeed where the heuristics in fully automated reasoning might fail. For our externally facing customer features, we currently use only fully automated approaches.
Next steps
In this essay, I’ve introduced the idea of automated reasoning, with the smallest of toy programs. I haven’t described how to handle realistic programs, with heap or concurrency. In fact, there are a wide variety of automated-reasoning tools and techniques, solving problems in all kinds of different domains, some of them quite narrow. To describe them all and the many branches and sub-disciplines of the field (e.g. “SMT solving”, “higher-order logic theorem proving”, “separation logic”) would take thousands of blogs posts and books.
Automated reasoning goes back to the early inventors of computers. And logic itself (which automated reasoning attempts to solve) is thousands of years old. In order to keep this post brief, I’ll stop here and suggest further reading. Note that it’s very easy to get lost in the weeds reading depth-first into this area, and you could emerge more confused than when you started. I encourage you to use a bounded depth-first search approach, looking sequentially at a wide variety of tools and techniques in only some detail and then moving on, rather than learning only one aspect deeply.
Suggested books:
- Handbook of Practical Logic and Automated Reasoning
- Temporal Verification of Reactive Systems
- Decision Procedures
- Model Checking
- Software Foundations
- Specifying Systems
- Introduction to Static Analysis
- Logic in Computer Science: Modelling and Reasoning about Systems
- Functional Algorithms, Verified!
- Handbook of Satisfiability
- The Calculus of Computation
International conferences/workshops:
- https://etaps.org/2020/tacas
- http://i-cav.org/2020/
- https://ijcar2020.org/
- https://popl21.sigplan.org/
- https://sat2020.idea-researchlab.org/
- http://smt-workshop.cs.uiowa.edu/
Tool competitions:
- http://termination-portal.org/wiki/Termination_Competition
- https://sv-comp.sosy-lab.org/2020/
- https://smt-comp.github.io/2020/
- http://www.satcompetition.org/
- http://www.tptp.org/CASC/27/
Some tools:
- AGREE: http://loonwerks.com/tools/agree.html
- Alloy: https://alloytools.org/
- Aprove: https://aprove.informatik.rwth-aachen.de/
- BioModelAnalzyer: https://biomodelanalyzer.com/
- Boogie: https://github.com/boogie-org/boogie.git
- CBMC: https://www.cprover.org/cbmc/
- Checked C: https://plum-umd.github.io/projects/checkedc.html
- Checker Framework: https://checkerframework.org/
- CoCoSim: https://github.com/NASA-SW-VnV/CoCoSim
- Coq: https://coq.inria.fr/
- CPA Checker: https://cpachecker.sosy-lab.org/
- CVC4: https://cvc4.github.io/
- Dafny: https://github.com/dafny-lang/dafny
- Dreal: https://github.com/dreal/dreal4
- HOL light: https://www.cl.cam.ac.uk/~jrh13/hol-light/
- Infer: https://fbinfer.com
- Iris: https://iris-project.org/
- Isabelle: https://isabelle.in.tum.de/
- Java PathFinder: https://github.com/javapathfinder
- JKind: https://github.com/loonwerks/jkind
- Keymaera X: https://keymaerax.org/
- Kind2: https://kind2-mc.github.io/kind2
- KLEE: https://klee.github.io
- Lean: https://leanprover.github.io/
- MiniSat: http://minisat.se/
- Nagini: https://github.com/marcoeilers/nagini
- P: https://github.com/p-org/P
- PRISM:https://www.prismmodelchecker.org
- PVS: https://pvs.csl.sri.com
- Rosette: http://emina.github.io/rosette/
- Rust programming language: https://www.rust-lang.org/ [When you are programming in Rust you are essentially proving the absence of memory corruption in a type system (assuming you're not using "unsafe" regions)]
- Sally: https://github.com/SRI-CSL/sally
- SAW: https://saw.galois.com/
- SeaHorn: http://seahorn.github.io/
- SMACK: https://smackers.github.io
- Soot: http://soot-oss.github.io/soot/
- SPIN: http://spinroot.com/spin/whatispin.html
- T2: https://mmjb.github.io/T2/
- TLA+: https://lamport.azurewebsites.net/tla/tla.html
- Vampire: https://vprover.github.io/
- VCC: https://github.com/microsoft/vcc
- Verifast: https://github.com/verifast/verifast
- Z3: https://github.com/Z3Prover/z3
Interviews of Amazon staff about their use of automated reasoning:
- Byron Cook PLDI'20 Ask Me Anything
- Byron Cook on The CUBE
- Neha Rungta on The CUBE
- Neha Rungta discusses constraint-based reasoning tools in AWS Config Rules
- Serdar Tasiran CAV'21 Ask Me Anything
- LogMeIn: How LogMeIn Automates Governance and Empowers Developers at Scale
AWS Lectures aimed at customers and industry:
- Automating Compliance Verification on AWS Using Provable Security, lecture with AWS VP of compliance Chad Woolf, and compliance auditor Coalfire's CEO Tom McAndrew
- An AWS Approach to Higher Standards of Assurance w/ Provable Security, Byron Cook
- Dive Deep into IAM Access Analyze, lecture by Andrew Gacek and others
- The Evolution of automated reasoning Technology at AWS, lecture with AWS VP of security Eric Brandwine
- Lecture by AWS CISO and VP of security Steve Schmidt about on the development and use of formal/constraint-based tools in AWS
- Re:Invent Keynote, lecture by CTO Werner Vogels
AWS talks aimed at the automated-reasoning science community:
- Debugging Network Reachability with Blocked Paths, CAV'21
- Embedded World 2021: Formally Verifying the FreeRTOS IPC Mechanism, Embedded World '21
- Formal reasoning about the security of Amazon Web Services, FLoC 2018 plenary lecture
- Formal reasoning about the security of Amazon Web Services, OOPSLA/SPLASH 2018 keynote lecture
- How I learned to stop worrying and start applying automated reasoning, FACC'21 (other relevant talks at FACC website)
- On automated reasoning for compliance certification, CAV workshop on Formal Approaches to Certifying Compliance (FACC)
- Pre-Deployment Security Assessment for Cloud Services through Semantic Reasoning, CAV'21
- Provable Security at AWS, USENIX Enigma 2019
- [Skip to 30mins in]: SideTrail: Verifying Time-Balancing of Cryptosystems
- Stratified abstraction of access control policies, CAV'20
- Verified Cryptographic Code for Everybody, CAV'21
- What is automated reasoning? How Is it Used at AWS?
AWS blog posts and informational videos:
- A simpler way to assess the network exposure of EC2 instances: AWS releases new network reachability assessments in Amazon Inspector
- AWS CTO blogs about automated reasoning Group: Proving security at scale with automated reasoning
- AWS CTO discusses S3 consistency
- AWS Podcast interview: Provable security podcast: Byron interviews Moshe Vardi
- AWS Podcast interview: Next Generation Security with automated reasoning, an Artificial Intelligence Technology
- Blog post about event-driven checks in AWS Config powered by automated constraint solving techniques over IAM policies [attend this session at NY summit] ... and more detail
- Blog post on the use of constraint-based reasoning about policies in the Amazon Web Services S3 console
- Blogs 1, 2, and 3 about work with Galois to prove the correctness of Amazon's crypto infrastructure component s2n
- Chad Wolff (VP of compliance at AWS) discusses his interest in using automated reasoning to simplify and raise the bar on compliance certification
- Daniel Schwartz-Narbonne shares how automated reasoning is helping achieve the provable security of AWS boot code
- How automated reasoning helps us innovate at S3 scale
- How automated reasoning improves Prime Vdeo experience
- How AWS SideTrail verifies key AWS cryptography code
- How AWS uses automated reasoning to help you achieve security at scale
- Jeff Barr about tools for IoT configuration verification
- On VPC Reachablity Analyzer
- New (Zelkova-based) AWS Config rules s3-blacklisted-actions-prohibited and bucket-policy-not-more-permissive are released
- Podcast: AI tech named automated reasoning provides next-gen cloud security
- Some detail about constraint-based IAM policy analysis used in Amazon Macie can be found here
- Tightening application security with Amazon CodeGuru
- Using Formal Methods to validate OTA Protocol
- ... more AWS blogs
Some course notes by Amazon Scholars who are also university professors:
- https://courses.cs.washington.edu/courses/cse507/21au/
- http://www.cs.cmu.edu/~mheule/15816-f21/
- https://www.cs.cmu.edu/~mheule/15217-f21/
A fun deep track:
Some algorithms found in the automated theorem provers we use today date as far back as 1959, when Hao Wang used automated reasoning to prove the theorems from Principia Mathematica.