Customer-obsessed science
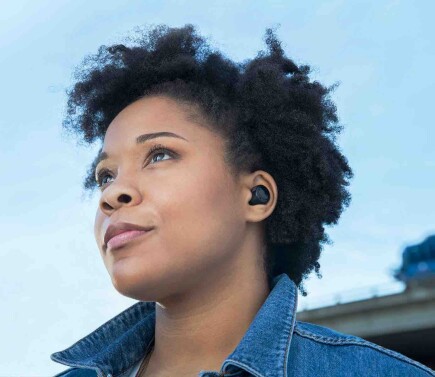
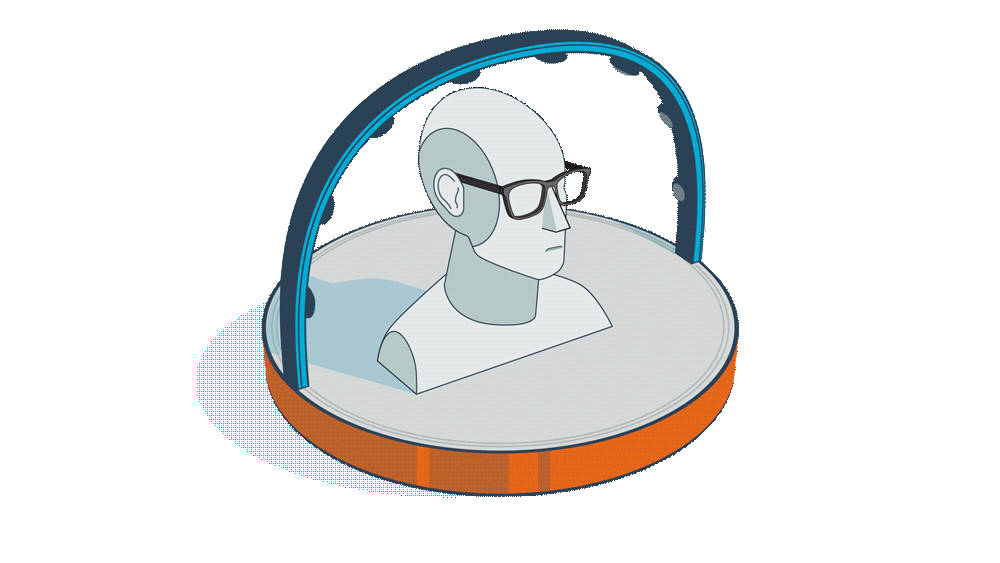
Research areas
-
April 11, 2025Novel three-pronged approach combines claim-level evaluations, chain-of-thought reasoning, and classification of hallucination error types.
-
-
Featured news
-
AAAI 2025 Workshop on Advancing LLM-Based Multi-Agent Collaboration2025Large Language Models (LLMs) have revolutionized AI-generated content evaluation, with the LLM-as-a-Judge paradigm becoming increasingly popular. However, current single-LLM evaluation approaches face significant challenges, including inconsistent judgments and inherent biases from pre-training data. To address these limitations, we propose CollabEval, a novel multi-agent evaluation framework that implements
-
2025Traditional segmentation models, while effective in isolated tasks, often fail to generalize to more complex and open-ended segmentation problems, such as free-form, open-vocabulary, and in-the-wild scenarios. To bridge this gap, we propose to scale up image segmentation across diverse datasets and tasks such that the knowledge across different tasks and datasets can be integrated while improving the generalization
-
PLDI 20252025We present the first technique to synthesize programs that compose side-effecting functions, pure functions, and control flow, from partial traces containing records of only the side-effecting functions. This technique can be applied to synthesize API composing scripts from logs of calls made to those APIs, or a script from traces of system calls made by a workload, for example. All of the provided traces
-
2025Recent advancements in 3D Large Multi-modal Models (3D-LMMs) have driven significant progress in 3D question answering. However, recent multi-frame VisionLanguage Models (VLMs) demonstrate superior performance compared to 3D-LMMs on 3D question answering tasks, largely due to the greater scale and diversity of available 2D image data in contrast to the more limited 3D data. Multi-frame VLMs, although achieving
-
ICMLT 20252025Applications of reinforcement learning (RL) in real-world scenarios are often limited by its generalizability across multiple different environments. Contextual RL offers a principled solution to this issue by capturing environmental heterogeneity through observable contextual variables. However, directly applying Contextual RL may not achieve optimal results when contexts exhibit high randomness and variance
Academia
View allWhether you're a faculty member or student, there are number of ways you can engage with Amazon.
View all