In 2020, Amazon’s retail fulfillment network in the U.S. expanded at a rapid clip. What followed was a dramatic — and swift — operational pivot.
This is the story of how Amazon’s national network of U.S. fulfillment centers (FCs), intermediate sorting centers, “last mile” delivery hubs, and transportation fleet were successfully restructured into eight largely self-sufficient regional networks, while retaining national coverage. The transformation was dubbed “regionalization.”
The COVID pandemic was a key factor in two ways. Due to lockdowns or otherwise, people were staying home and ordering more online than ever before.
“Our focus moved from trying to figure out how to make customer deliveries as fast as possible to trying to meet exceptional customer demand by pushing as much volume as we could through our network,” says Adam Baker, Amazon’s vice president of global transportation.
It was in late 2020 that a long-term planning science team led by research director Amitabh Sinha sent up a warning flare: the fast-growing network risked becoming overcomplicated and unwieldy.
“We projected our scenario out to three or four years and took this to Amazon’s leadership with an idea of how to do things differently,” he says. That idea contained the seed that would grow into regionalization.
Joining the dots
The crux of the issue was that Amazon was trying to connect too many physical dots. Its fulfillment infrastructure made sense when it had fewer FCs, because it meant customers across the U.S. could tap into Amazon’s full product range. And with fewer FCs, the trucks carrying the products across the country were fuller, so it was cost-effective.
As the number of FCs and other fulfillment buildings in the U.S. rose sharply, that approach started to look like it might not be the right long-term path. “We would fulfill customer orders from the FCs near them until we couldn’t anymore, and then — okay, it's coming from wherever we still have capacity,” says Russell Allgor, Amazon’s chief scientist for worldwide operations. That “wherever” was the problem.
It meant each of Amazon’s FCs was serving not only its locality, but also customer locations all over the U.S. To illustrate the problem, imagine you had to deliver 10,000 products nationwide, quickly, to 100 distant locations, from 10 FCs across the country. You could have each FC dispatch 100 trucks, each carrying 10 items, to each of the locations. That’s 1,000 long-haul trucks and a lot of rubber on the road — clearly an unsustainable idea on all fronts.
Now imagine that you could partition the 100 customer locations into 10 regions of 10 locations apiece, with each region served by a dedicated FC. In this scenario, each region’s FC can dispatch 10 trucks, each carrying 100 packages a piece. That would require just 100 trucks nationwide, driving much shorter distances. That’s faster for customers and more sustainable: a win-win situation. That’s regionalization in a nutshell, and by mid-2021, Amazon threw its full weight behind the idea.
Picking the number
For about a year, Sinha and his team used state-of-the-art network-optimization tools to model and simulate the many potential ways customer orders might flow through a regionalized system, and what effect different configurations would have on delivery speeds and transport costs. There was an enormous number of potential scenarios to explore.
“We were dealing with millions of variables and constraints, and a lot of uncertainty,” says Cristiana Lara, a senior research scientist who worked on estimating the financial impacts of the initiative. “That’s not surprising, because we were completely shifting the paradigm of how we fulfill customer orders.”
A critical early question was how many regions to form. The smaller the regions, the faster the customer deliveries, because Amazon’s inventory would be closer to customers. “In addition to speedy deliveries, the crucial thing was that each region must carry the breadth of selection that customers expect” says Sinha.
The ambitious aim? To have a high proportion of the tens of millions of products offered in the store available to customers within each region, with the rest shipped from further afield only when needed.
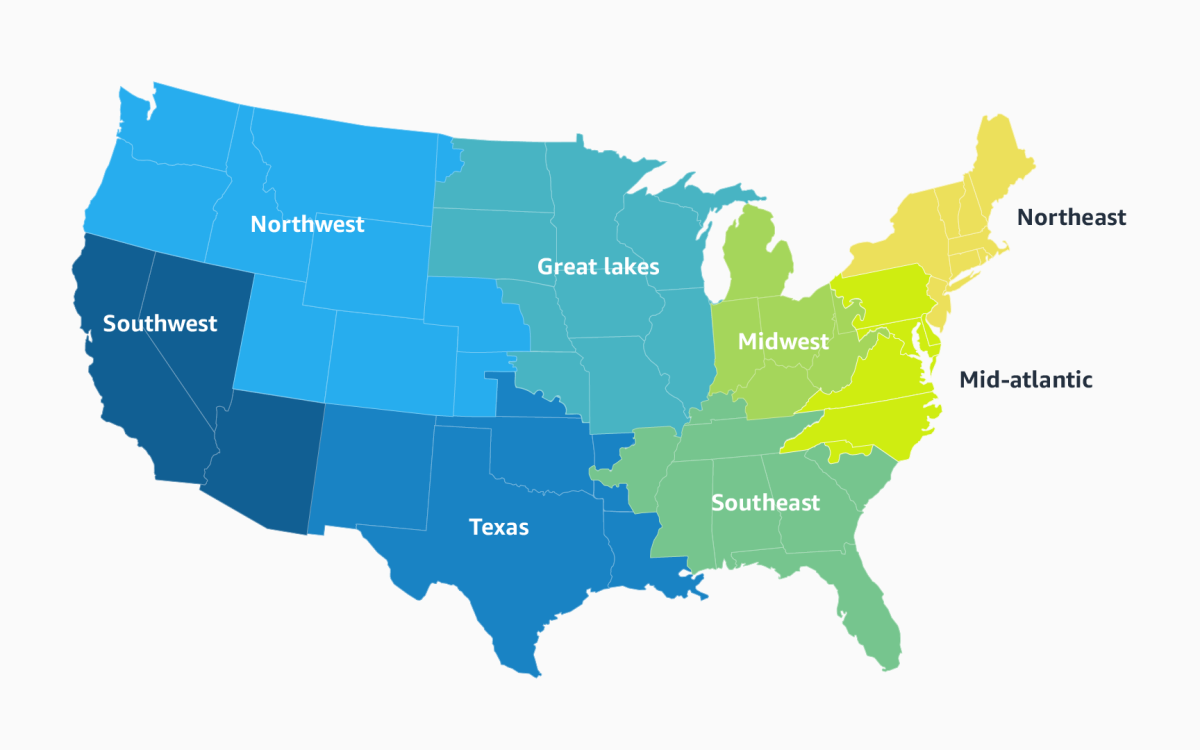
With this goal in mind, the collaborators alighted on the number: eight regions. That was as high as they could go without sacrificing speed or requiring excessive inter-regional movement of inventory to meet customer orders, which would defeat the purpose of the exercise.
“We ran extensive, fine-grained analysis for pretty much the entirety of 2022, examining in turn the different aspects of how it would all work,” says Sinha, whose team worked closely with Amazon’s Global Transportation Service (GTS) – which designs, plans, and executes the Amazon Transportation network.
Before long, a timeline was put in place. Come January 18, 2023, the newly minted Northeast and Mid-Atlantic Amazon regions would pioneer this new fulfillment pattern, with the other six regions slated to follow thereafter.
Flipping the switch
A critical piece of regionalization was using the insights supplied to map out more efficient, shorter routes for orders. As soon as a customer clicks the "buy now" button, Amazon's Adaptive TRansportation OPtimization Service (ATROPS) assigns the optimal route for the purchased item. The transportation team devoted the latter part of 2022 to overhauling and testing a completely new set of ATROPS routes designed specifically for this regionalization plan.
On January 18, with the 2022 holiday rush safely in the rearview mirror, it was time to make the leap. The transportation team had contingency plans in place, and colleagues in different global time zones were standing by to offer around-the-clock support if something went wrong.
“We flipped the switch overnight, and immediately started to see the results we were hoping for. It changed faster than any of us expected. It was delightful,” says Nick McCabe, senior manager of GTS network design.
“We had some minor concerns to work through,” Baker adds, “but our delivery speed instantly picked up and our customers saw the benefit in their orders right away.”
Overall, the transition went so well, Amazon brought forward the complete activation of the other regions by a full month.
Rapid results
Regionalization is working. Before the switch, the percentage of customer orders being fulfilled entirely from FCs within what would become each region was 62%. That figure has already surged to 76% — a stunning efficiency gain — and is expected to continue to climb.
Delivery speeds have also picked up, says Sinha, because more goods are travelling shorter distances. And this effect will only strengthen as regionalization continues to take root.
Another quick success of regionalization was how much fuller a subset of Amazon’s trucks has become. Most customer orders leave an FC and are transported to a sorting center, which receives and consolidates customer orders, filling up the trucks that take them to delivery stations for the “last mile” of their journey. Sometimes, for logistical reasons, FCs send trucks directly to delivery stations. Post-regionalization, because there are fewer FCs shipping more packages to each destination, there is greater opportunity to operate these FC-to-delivery station direct trucks, resulting in more efficient delivery routes.
“Suddenly, 70 to 80 per cent of the order volume is not coming from FCs scattered around the country, but from, say, 10 FCs inside the region, so trucks on these short-distance direct lanes are now showing a great fill rate,” says senior applied scientist Semih Atakan, who models how products flow between Amazon’s FCs and delivery stations.
Regionalization has also transformed how the wider national network is managed.
“Before, it was difficult to control the whole network because of our sheer number of trucking lanes,” says Baker. “It was like pushing on a giant spiderweb.” Post-regionalization, he says, that number of lanes reduced markedly, making it much easier to make choices about when and how much to ship between regions.
Scanning the horizon
And this is just the beginning, says research scientist Xiaoyan Si, who is modeling how the fulfillment network might evolve over the next three years.
“Eight regions is our starting point. As we move forward, we will have the opportunity create smaller geographic regions with as much demand per region as we have today,” says Si. “Using the data we have now, we can place future fulfillment buildings more strategically, and we are working with other researchers on the team to design new regions more scientifically.”
Smaller regions will enable Amazon to deliver even faster to customers, while making each region even more efficient in terms of distance travelled, inventory management and truck fill.
Amazon’s Day One culture places great value on horizon scanning, innovation, and risk-taking to deliver customer benefits. The regionalization initiative that sprang from this mindset is a testament not only to the vision and enormous team effort required to pull it off, but also to the flexibility of Amazon’s infrastructure.
Because despite being Amazon’s biggest operational transformation in a decade, it was completely reversible had it misfired. After all, says Si, in what might be the understatement of the year: “When you boil it right down, regionalization is just a software setting.”