On July 12 and 13, millions of Amazon Prime members visited the Amazon Store to score deals on everything from televisions to clothes. Customers think of Prime Day as a big annual sale. Supply chain managers call it a peak event — one that requires advanced planning to ensure people can buy the discounted products they want and receive them as quickly as possible.
This year was also the biggest Prime Day event for Amazon's selling partners, most of whom are small and medium-sized businesses, whose sales growth in Amazon’s store outpaced Amazon's retail business.
The scientists on Amazon's Supply Chain Optimization Technologies (SCOT) team have spent many years building and refining processes to handle peak events like Prime Day, Black Friday, Valentine's Day, and all of the other occasions where shoppers are looking for specific discounted items. The team's algorithms help determine which products to stock, and in what quantities; where and how to store them; and the best way to route them to customers.
But what happens when a "peak event'' is unplanned and lasts more than two years?
The COVID-19 pandemic touched off a series of demand spikes, supply chain disruptions, and labor shortages that have persisted with varying intensity since early 2020. The SCOT team was already utilizing state-of-the-art tools in machine learning, mathematical modelling, and optimization. Those tools played an essential role in the pandemic response. The crisis also sparked an examination of how those tools could be adapted to manage the new normal.
A different kind of peak
Even on a ho-hum day in pre-COVID times, managing inventory in Amazon's gargantuan network was a task that drew scientists intrigued by the complexity of challenges, the sheer volume of data, and the opportunity to have customer impact at global scale.
Scientists on the demand forecasting team, for example, have been doing this in one form or another for more than 15 years, constantly refining the algorithms that help ensure Amazon stocks enough of the products customers want.
As a planning challenge, an event like Prime Day might seem manageable. After all, Amazon sets the dates, picks the products, and sets the discounts. But even a planned event like Prime Day, now in its eighth year, brings its own challenges.
There's a special construction to the [Prime Day] forecast. It's a different week of the year than every other year.
"There's a special construction to the forecast. It's a different week of the year than every other year," explains Abhishek Gupta, who leads the demand forecasting science team within SCOT.
The forecasting team must account not only for the anomaly of Prime Day itself, but the subtleties within Prime Day.
"Just understanding the interaction between the deal attributes and the time of the year is one challenge," Gupta says. "The dates that Prime Day falls on, as well as the types of deals available, change from year to year. It's not like Halloween, where we know that everyone is going to look for costumes."
Before the pandemic, Gupta's team was already employing deep-learning models for time series forecasting, essentially teaching the network to discern patterns in data, like sales of string lights going up around the holidays.
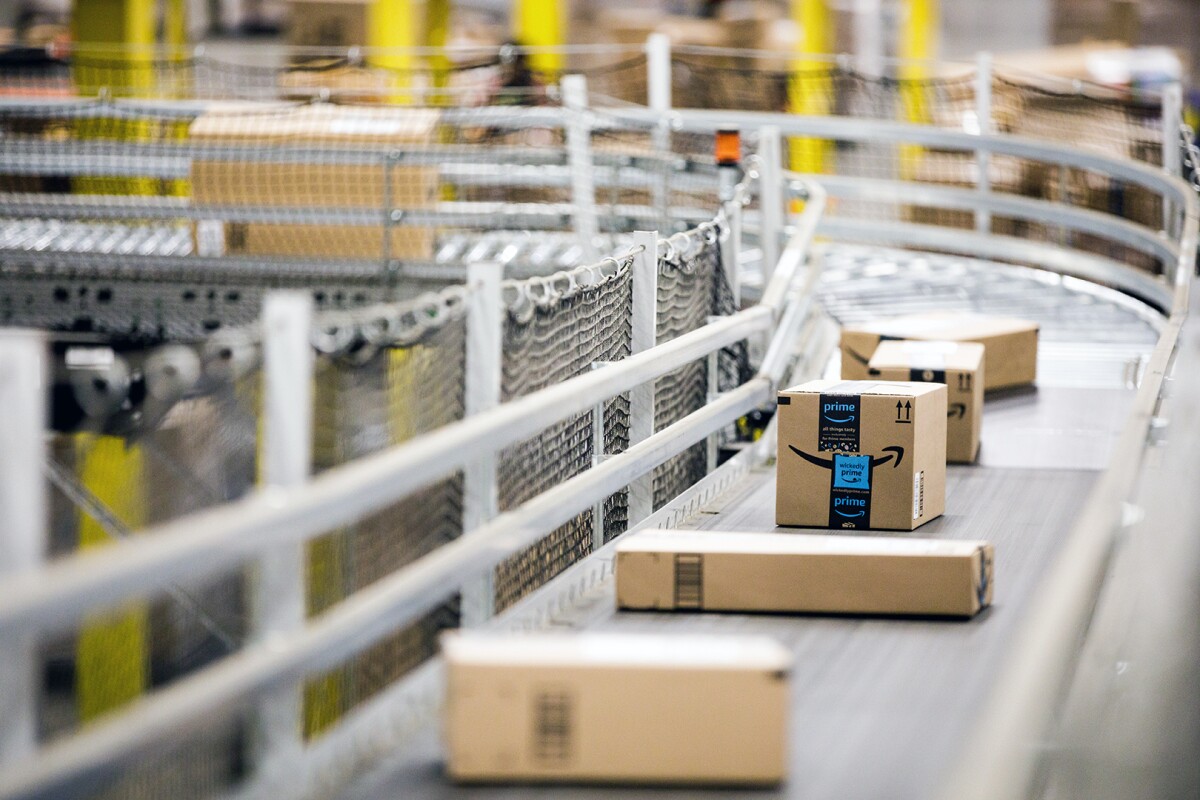
But these models were not as strong when it came to a predictable-yet-unpredictable event like Prime Day. Constantly looking to improve the technology further, the forecasting science team took inspiration from advances in natural language processing to improve its models.
Natural language processing models use a mechanism called attention to enhance understanding. Attention directs models to “attend to” specific words that suggest a logical next word, e.g. the word “dog” may be followed by “food.”
Time series data are also sequential in nature, and they can uncover deeper insights by associating similar periods in time.
Over the past two years, the SCOT team has developed and deployed an encoder-decoder attention scheme that enables the network to look at the time series history and identify the previous time points most relevant for the forecasting period.
"The impact of these types of models in natural language processing have been game-changing," Gupta says. "And similarly, we're seeing extremely good results in our time series forecasts. The accuracy has improved substantially, and the volatility of the forecast has gone down."
A massive simulation platform
All of the decisions that follow forecasting — capacity planning, buying, placement, storage, and fulfillment — are merged into a large-scale, distributed simulation system that helps the SCOT team manage inventory as demand fluctuates.
It's arguably the biggest simulation platform in the world. The underlying dynamics are so complicated and there are many decision makers...
"It's arguably the biggest simulation platform in the world," says Yan Xia, a principal applied scientist within SCOT’s Inventory Planning and Control team. "The underlying dynamics are so complicated, and there are many decision makers. It's impossible to capture through a set of mathematical equations."
Xia and colleagues are experts at managing various physical capacity constraints in Amazon’s multi-tier global fulfillment network.
Peak events like Prime Day or the holidays present potential constraint challenges, such as whether Amazon can store the right amount of inventory in the right location as customer demand spikes. Tradeoffs need to be made in terms of what inventory to carry to best protect the customer experience in those instances.
By 2019, Amazon scientists had developed a patented Adaptive Capacity Control (ACC) tool designed precisely for these occasions. In the lead-up to Black Friday, Xia's team would run the tool over a few weeks to help determine inventory for the holiday rush.
"All of that changed when COVID hit us. We were severely constrained all over the place, and we had to push this tool to its limit to solve some capacity related problems that the team hadn’t ever encountered before," Xia says. "We went from solving for capacity in maybe two countries eight weeks a year to maybe a dozen countries 52 weeks a year — and at a much more granular level than before."
So the team worked to both improve the ACC tool and develop new control systems to manage capacity more comprehensively and automatically as capacity challenges became more prevalent.
The predictive power of sampling
"The pandemic was like a peak that lasted two years," says Keith Zackrone, director of software development within Inventory Planning and Control. "The mechanisms that we've used to plan for peak events really are what gave us the ability to operate through the pandemic with good trade-offs for customers."
For the ACC tool, that meant reworking it for a "warm start," Xia says, using historical data to update the capacity control inputs on a rolling basis, rather than beginning with a blank slate every time, as before.
The team also began running simulations on samples of the inventory. That allowed them to predict how the larger inventory flow would respond to control signals based on less than 5% of the total, rather than trying to simulate all of the hundreds of millions of items Amazon stocks at any given time.
"We are very effective nowadays at sampling," Xia says. "That is a big deal for us, to be able to execute this across so many different types of capacity within a marketplace — and also across marketplaces."
The SCOT team also adapted its inventory control software to be much more aware of customer need, meaning it could prioritize items that customers needed quickly (e.g., baby food), versus others that could tolerate a slight delay (e.g., a camera lens). That sensitivity has enabled the team to optimize the system across a larger number of product categories.
"Now that we've emerged from the peak period of the pandemic, we have an opportunity to move to a second generation," Zackrone says. "We can fine-tune these models we built out during the pandemic and really look at how we can make this available to a wider product selection."
The pandemic and ongoing supply chain issues have highlighted that there's never a perfect crystal ball, but they have helped the SCOT team strengthen the algorithms it uses to meet both peak events like Prime Day and everyday customer demand.
"If we can predict better, then we can buy the right amount and put it across our network of fulfillment centers,” explains Gupta. "This ensures that the right product, gets to the right customer in the most efficient way possible.”