When we first joined AWS AI/ML as Amazon Scholars over three years ago, we had already been doing scientific research in the area now known as responsible AI for a while. We had authored a number of papers proposing mathematical definitions of fairness and machine learning (ML) training algorithms enforcing them, as well as methods for ensuring strong notions of privacy in trained models. We were well versed in adjacent subjects like explainability and robustness and were generally denizens of the emerging responsible-AI research community. We even wrote a general-audience book on these topics to try to explain their importance to a broader audience.
So we were excited to come to AWS in 2020 to apply our expertise and methodologies to the ongoing responsible-AI efforts here — or at least, that was our mindset on arrival. But our journey has taken us somewhere quite different, somewhere more consequential and interesting than we expected. It’s not that the definitions and algorithms we knew from the research world aren’t relevant — they are — but rather that they are only one component of a complex AI workstream comprising data, models, services, enterprise customers, and end-users. It’s also a workstream in which AWS is uniquely situated due to its pioneering role in cloud computing generally and cloud AI services specifically.
Our time here has revealed to us some practical challenges of which we were previously unaware. These include diverse data modalities, “last mile” effects with customers and end-users, and the recent emergence of AI activism. Like many good interactions between industry and academia, what we’ve learned at AWS has altered our research agenda in healthy ways. In case it’s useful to anyone else trying to parse the burgeoning responsible-AI landscape (especially in the generative-AI era), we thought we’d detail some of our experiences here.
Modality matters
One of our first important practical lessons might be paraphrased as “modality matters”. By this we mean that the particular medium in which an AI service operates (such as visual images or spoken or written language) matters greatly in how we analyze and understand it from both performance and responsible-AI perspectives.
Consider specifically the desire for trained models be “fair”, or free of significant demographic bias. Much of the scientific literature on ML fairness assumes that the features used to compare performance across groups (which might include gender, race, age, and other attributes) are readily available, or can be accurately estimated, in both training and test datasets.
If this is indeed the case (as it might be for some spreadsheet-like “tabular” datasets recording things like medical or financial records, in which a person’s age and gender might be explicit columns), we can more easily test a trained model for bias. For instance, in a medical diagnosis application we might evaluate the model to make sure the error rates are approximately the same across genders. If these rates aren’t close enough, we can augment our data or retrain the model in various ways until the evaluation is passed to satisfaction.
But many cloud AI/ML services operate on data that simply does not contain explicit demographic information. Rather, these services live in entirely different modalities such as speech, natural language, and vision. Applications such as our speech recognition and transcription services take as input time series of frequencies that capture spoken utterances. Consequently, there are not direct annotations in the data of things like gender, race, or age.
But what can be more readily detected from speech data, and are also more directly related to performance, are regional dialects and accents — of which there are dozens in North American English alone. English-language speech can also feature non-native accents, influenced more by the first languages of the speakers than by the regions in which they currently live. This presents an even more diverse landscape, given the large number of first languages and the international mobility of speakers. And while spoken accents may be weakly correlated or associated with one or more ancestry groups, they are usually uninformative on things like age and gender (speakers with a Philadelphia accent may be young or old; male, female or nonbinary; etc.). Finally, the speech of even a particular person may exhibit many other sources of variation, such as situational stress and fatigue.
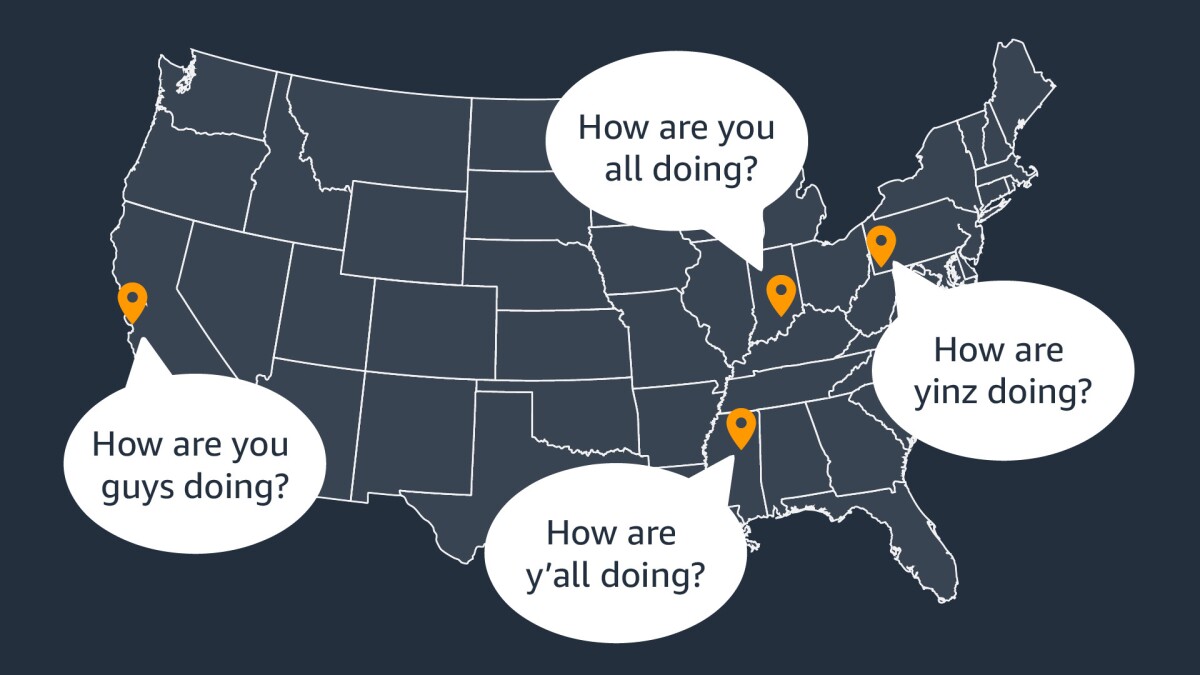
What is the responsible-AI practitioner to do when confronted with so many different accents and other moving parts, in a task as complex as speech transcription? At AWS, our answer is to meet the task and data on their own terms, which in this case involves some heavy lifting: meticulously gathering samples from large populations of representative speakers with different accents and carefully transcribing each word. The “representative” is important here: while it might be more expedient to (for instance) gather this data from professional actors trained in diction, such data would not be typical of spoken language in the wild.
We also gather speech data that exhibits variability along other important dimensions, including the acoustic conditions during recording (varying amounts and types of background noise, recordings made via different mobile-phone handsets, whose microphones may vary in quality, etc.). The sheer number of combinations makes obtaining sufficient coverage challenging. (In some domains such as computer vision, coverage issues that are similar — variability across visual properties such as skin tone, lighting conditions, indoor vs. outdoor settings, and so on — have led to increased interest in synthetic data to augment human-generated data, including for fairness testing here at AWS.)
Once curated, such datasets can be used for training a transcription model that is not only good overall but also roughly equally performant across accents. And “performant” here means something more complex than in a simple prediction task; speech recognition typically uses a measure like the word error rate. On top of all the curation and annotations above, we also annotate some data by self-reported speaker demographics to make sure we’re fair not just by accent but by race and gender as well, as detailed in the service’s accompanying service card.
Our overarching point here is twofold. First, while as a society we tend to focus on dimensions such as race and gender when speaking about and assessing fairness, sometimes the data simply doesn’t permit such assessments, and it may not be a good idea to impute such dimensions to the data (for instance, by trying to infer race from speech signals). And second, in such cases the data may lead us toward alternative notions of fairness that might be more task-relevant, as with word error rates across dialects and accents.
The last mile of responsible AI
The specific properties of individuals that can or cannot (or should not) be gleaned from a particular dataset or modality are not the only things that may be out of the direct control of AI developers — especially in the era of cloud computing. As we have seen above, it’s challenging work to get coverage of everything you can anticipate. It’s even harder to anticipate everything.
The supply chain phrase “the last mile” refers to the fact that “upstream” providers of goods and products may have limited control over the “downstream” suppliers that directly connect to end-users or consumers. The emergence of cloud providers like AWS has created an AI service supply chain with its own last-mile challenges.
AWS AI/ML provides enterprise customers with API access to services like speech transcription because many want to integrate such services into their own workflows but don’t have the resources, expertise, or interest to build them from scratch. These enterprise customers sit between the general-purpose services of a cloud provider like AWS and the final end-users of the technology. For example, a health care system might want to provide cloud speech transcription services optimized for medical vocabulary to allow doctors to take verbal notes during their patient rounds.
As diligent as we are at AWS at battle-testing our services and underlying models for state-of-the-art performance, fairness, and other responsible-AI dimensions, it is obviously impossible to anticipate all possible downstream use cases and conditions. Continuing our health care example, perhaps there is a floor of a particular hospital that has new and specialized imaging equipment that emits background noise at a specific regularity and acoustic frequency. In the likely event that these exact conditions were not represented in either the training or test data, it’s possible that overall word error rates will not only be higher but may be so differentially across accents and dialects.
Such last-mile effects can be as diverse as the enterprise customers themselves. With time and awareness of such conditions, we can use targeted training data and customer-side testing to improve downstream performance. But due to the proliferation of new use cases, it is an ever-evolving process, not one that is ever “finished”.
AI activism: from bugs to bias
It’s not only cloud customers whose last miles may present conditions that differ from those during training and testing. We live in a (healthy) era of what might be called AI activism, in which not only enterprises but individual citizens — including scientists, journalists, and members of nonprofit organizations — can obtain API or open-source access to ML services and models and perform their own evaluations on their own curated datasets. Such tests are often done to highlight weaknesses of the technology, including shortfalls in overall performance and fairness but also potential security and privacy vulnerabilities. As such, they are typically performed without the AI developer’s knowledge and may be first publicized in both research and mainstream media outlets. Indeed, we have been on the receiving end of such critical publicity in the past.
To date, the dynamic between AI developers and activists has been somewhat adversarial: activists design and conduct a private experimental evaluation of a deployed AI model and report their findings in open forums, and developers are left to evaluate the claims and make any needed improvements to their technology. It is a dynamic that is somewhat reminiscent of the historical tensions between more traditional software and security developers and the ethical and unethical hacker communities, in which external parties probe software, operating systems, and other platforms for vulnerabilities and either expose them for the public good or exploit them privately for profit.
Over time the software community has developed mechanisms to alter these dynamics to be more productive than adversarial, in particular in the form of bug bounty programs. These are formal events or competitions in which software developers invite the hacker community to deliberately find vulnerabilities in their technology and offer financial or other rewards for reporting and describing them to the developers.
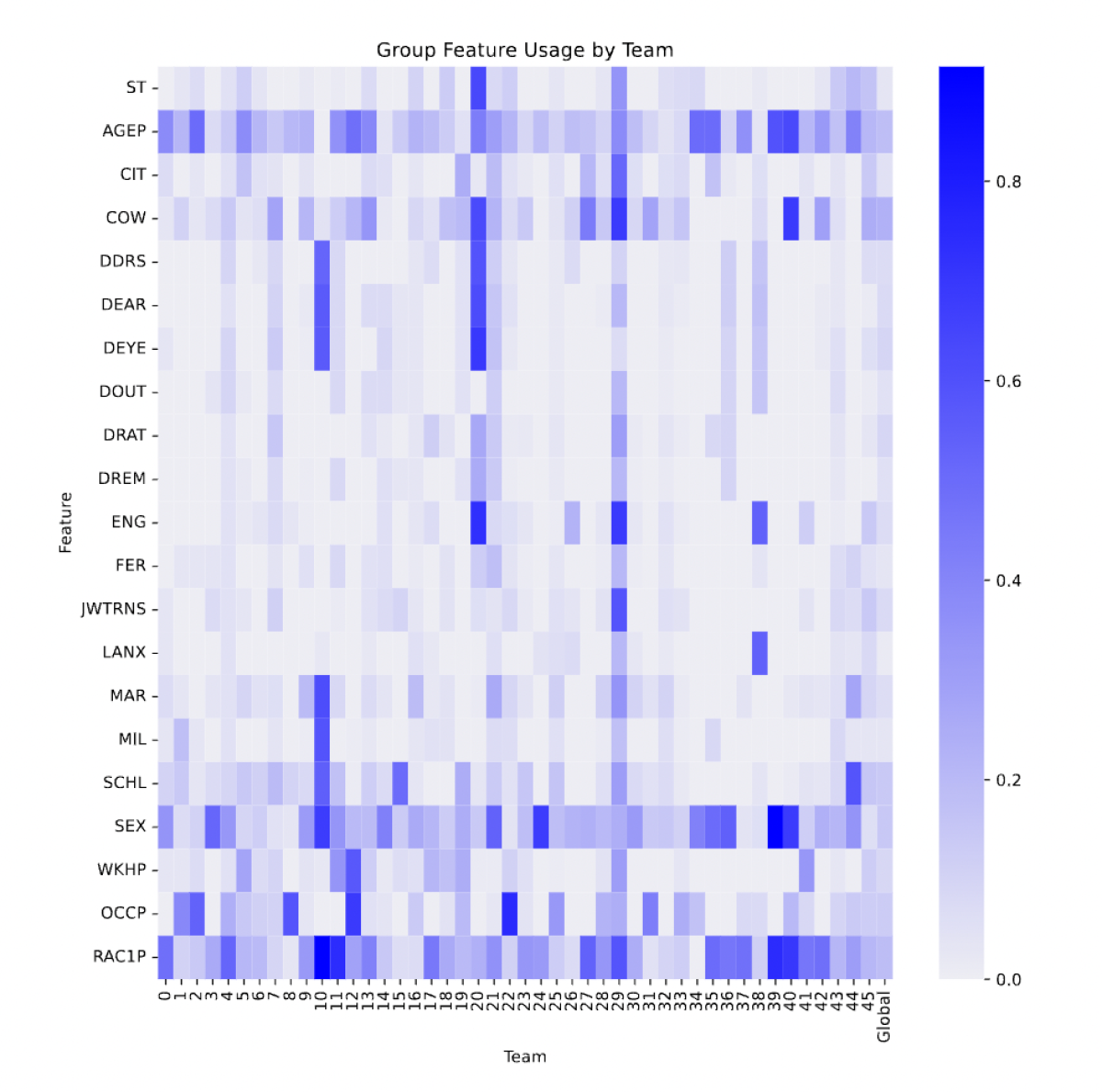
In the last couple of years, the ideas and motivations behind bug bounties have been adopted and adapted by the AI development community, in the form of “bias bounties”. Rather than finding bugs in traditional software, participants are invited to help identify demographic or other biases in trained ML models and systems. Early versions of this idea were informal hackathons of short duration focused on finding subsets of a dataset on which a model underperformed. But more recent proposals incubated at AWS and elsewhere include variants that are more formal and algorithmic in nature. The explosion of models, interest in, and concerns about generative AI have also led to more codified and institutionalized responsible-AI methodologies such as the HELM framework for evaluating large language models.
We view these recent developments — AI developers opening up their technology and its evaluation to a wider community of stakeholders than just enterprise customers, and those stakeholders playing an active role in identifying necessary improvements in both technical and nontechnical ways — as healthy and organic, a natural outcome of the complex and evolving AI industry. Indeed, such collaborations are in keeping with our recent White House commitments to external testing and model red-teaming.
Responsible AI is neither a problem to be “solved” once and for all, nor a problem that can be isolated to a single location in the pipeline stretching from developers to their customers to end-users and society at large. Developers are certainly the first line where best practices must be established and implemented and responsible-AI principles defended. But the keys to the long-term success of the AI industry lie in community, communication, and cooperation among all those affected by it.