At Amazon, we take the protection of customer data very seriously. We are also committed to eliminating the biases that can exist in off-the-shelf language models — such as GPT-3 and RoBERTa — that are the basis of most modern natural-language processing. Trained on public texts, these language models are known to reflect the biases implicit in those texts.
These two topics — privacy protection and fairness — are at the core of trustworthy machine learning, an important area of research at Alexa AI. In 2021, we made contributions in the following areas:
- Privacy-preserving machine learning: Differential privacy provides a rigorous way to quantify the privacy of machine learning models. We investigated vulnerabilities presented in the differential-privacy literature and propose computationally efficient mechanisms for protecting against them.
- Federated learning: Federated learning (FL) is a distributed-training technique that keeps customer data on-device. Devices send only model parameter updates to the cloud, not raw data. We studied several FL challenges arising in an industrial setting.
- Fairness in machine learning: Machine learning (ML) models should perform equally well regardless of who’s using them. But even knowing how to quantify fairness is a challenge. We introduced measures of fairness and methods to mitigate bias in ML models.
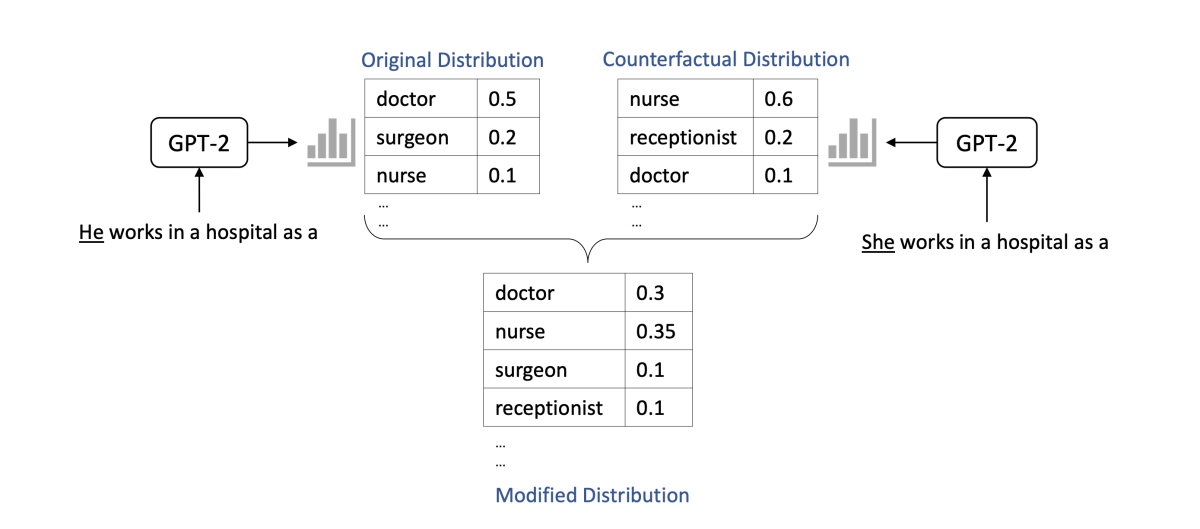
Below, we summarize our research in these areas, which will be presented at ACL and ICASSP later this year. We also invite readers to participate in workshops and sessions we are organizing at NAACL 2022 and Interspeech 2022.
1. Privacy-preserving ML
The intuition behind differential privacy (DP) is that access to the outputs of a model should not provide any hint about what inputs were used to train the model. DP quantifies that intuition as a difference (in probabilities) between the outputs of a model trained on a given dataset and the outputs of the same model trained on the same dataset after a single input is removed.
One way to meet a DP privacy guarantee is to add some noise to the model parameters during training in order to obfuscate their relationship to training data. But this can compromise accuracy. The so-called privacy/utility tradeoff appears in every DP application.
Another side effect of adding a DP mechanism is increased training time. Given that training natural-language-understanding (NLU) models with large volumes of data can be prohibitively slow and that industry standards require fast training and deployment — e.g., when new features are being released — we developed a training method that meets DP requirements but remains efficient. We describe the method in a paper we’re presenting at this year’s ICASSP, “An efficient DP-SGD mechanism for large scale NLP models”.
In this work, we study the most popular DP mechanism for deep neural networks, DP-SGD, and build a computationally efficient alternative, eDP-SGD, in which we use a batch-processing scheme that leverages the GPU architecture and automates part of the hyperparameter-tuning process. While both DP-SGD and eDP-SGD provide the same privacy guarantees, we show that the training time for our mechanism is very similar to its non-DP counterpart’s. The original DP-SGD extends training time as much as 130-fold.
Since we did our study, researchers have developed methods with stronger theoretical DP guarantees than the ones we impose in our paper, but our approach is consistent with those methods. Overall, this work makes DP more generally accessible and helps us integrate NLU models with DP guarantees into our production systems, where new models are frequently released, and a significant increase in training time is prohibitive.
While DP provides theoretical privacy guarantees, we are also interested in practical guarantees, i.e., measuring the amount of information that could potentially leak from a given model. In addition to the performance and training time of eDP-SGD, we also studied the correlation between theoretical and practical privacy guarantees. We measured practical privacy leakage using the most common method in the field, the success rate of membership inference attacks on a given model. Our experiments provide a general picture of how to optimize the privacy/utility trade-off using DP techniques for NLU models.
We also expanded the set of mechanisms for protecting NLU models against other types of attacks. In “Canary extraction in natural language understanding models”, which we will present at ACL 2022, we study the vulnerability of text classification models to a certain kind of white-box attack called a model inversion attack (ModIvA), where a fictional attack has access to the entire set of model parameters and intends to retrieve examples used during training. Existing model inversion techniques are applied to models with either continuous inputs or continuous outputs. In our work, we adopt a similar approach to text classification tasks where both inputs and outputs are discrete.
As new model architectures are developed that might display new types of vulnerabilities, we will continue innovating efficient ways of protecting our customers’ privacy.
Upcoming activities
2. Federated Learning
The idea behind federated learning (FL) is that, during the training of an ML model, part of the computation is delegated to customers’ devices, leveraging the processing power of those devices while avoiding the centralization of privacy-sensitive datasets. Each device modifies a common, shared model according to locally stored data, then sends an updated model to a central server that aggregates model updates and sends a new shared model to all the devices. At each round, the central server randomly selects a subset of active devices and requests that they perform updates.
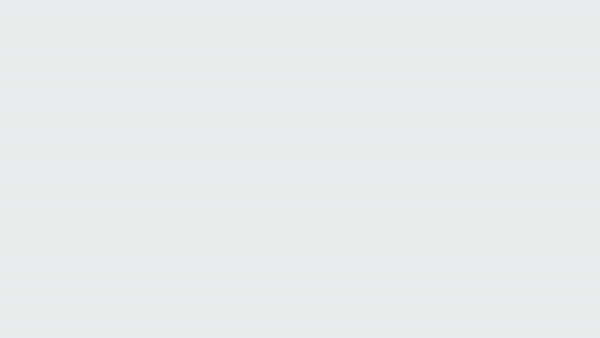
In the past year, we have made progress toward more-efficient FL and adapted common FL techniques to the industrial setting. For instance, in “Learnings from federated learning in the real world”, which we will present at ICASSP this year, we explore device selection strategies that differ from the standard uniform selection. In particular, we present the first study of device selection based on device “activity” — i.e., the number of available training samples.
These simple selection strategies are lightweight compared to existing methods, which require heavy computation from all the devices. They are thus more suitable to industrial applications, where millions of devices are involved. We study two different settings: the standard “static” setting, where all the data are available at once, and the more realistic “continual” setting, where customers generate new data over time, and past examples might have to be deleted to save storage space. Our experiments on training a language model with FL show that non-uniform sampling outperforms uniform sampling when applied to real-world data, for both the static and continual settings.
We also expanded our understanding of FL for natural-language processing (NLP) and, in the process, made FL more accessible to the NLP community. In “FedNLP: A research platform for federated learning in natural language processing”, which will be presented later this year at NAACL, we and our colleagues at the University of Southern California and FedML systematically compare the most popular FL algorithms for four mainstream NLP tasks. We also present different methods to generate dataset partitions that are not independent and identically distributed (IID), as real-world FL methods must be robust against shifts in the distributions of the data used to train ML models.
Our analysis reveals that there is still a large gap between centralized and decentralized training under various settings, and we highlight several directions in which FL for NLP can advance. The paper represents Amazon’s contribution to the open-source framework FedNLP, which is capable of evaluating, analyzing, and developing FL methods for NLP. The codebase contains non-IID partitioning methods, enabling easy experimentation to advance the state of FL research for NLP.
We also designed methods to account for the naturally heterogeneous character of customer-generated data and applied FL to a wide variety of NLP tasks. We are aware that FL still presents many challenges, such as how to do evaluation when access to data is removed, on-device label generation for supervised tasks, and privacy-preserving communication between the server and the different devices. We are actively addressing each of these and plan to leverage our findings to improve FL-based model training and enhance associated capabilities such as analytics and model evaluation.
Upcoming activities
- Special session on Trustworthy Speech Processing @ Interspeech 2022
- Invited speaker for FL4NLP @ ACL 2022
3. Fairness in ML
Natural-language-processing applications’ increased reliance on large language models trained on intrinsically biased web-scale corpora has amplified the importance of accurate fairness metrics and procedures for building more robust models.
In “On the intrinsic and extrinsic fairness evaluation metrics for contextualized language representations”, which we are presenting at ACL 2022, we compare two families of fairness metrics — namely extrinsic and intrinsic — that are widely used for language models. Intrinsic metrics directly probe into the fairness of language models, while extrinsic metrics evaluate the fairness of a whole system through predictions on downstream tasks.
For example, the contextualized embedding association test (CEAT), an intrinsic metric, measures bias through word embedding distances in semantic vector spaces, and the extrinsic metric HateXPlain measures the bias in a downstream hate speech detection system.
Our experiments show that inconsistencies between intrinsic and extrinsic metrics often reflect inconsistencies between the datasets used to evaluate them, and a clear understanding of bias in ML models requires more careful alignment of evaluation data. The results we report in the paper can help guide the NLP community as to how to best conduct fairness evaluations.
We have also designed new measures of fairness that are adapted to language-processing applications. In “Measuring fairness of text classifiers via prediction sensitivity”, which we will present at ACL 2022, we looked at sensitivity to perturbations of input as a way to measure fairness in ML models. The metric attempts to quantify the extent to which a single prediction depends on an input feature that encodes membership in an underrepresented group.
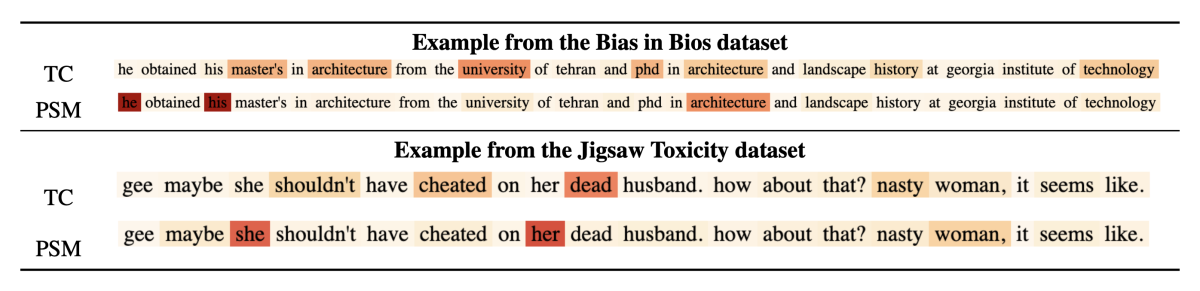
We provide a theoretical analysis of our formulation and show a statistically significant difference between our metric’s correlation with the human notion of fairness and the existing counterfactual fairness metric’s.
Finally, we proposed a method to mitigate the biases of large language models during knowledge distillation, in which a smaller, more efficient model is trained to match the language model’s output on a particular task. Because large language models are trained on public texts, they can be biased in multiple ways, including the unfounded association of male or female genders with gender-neutral professions.
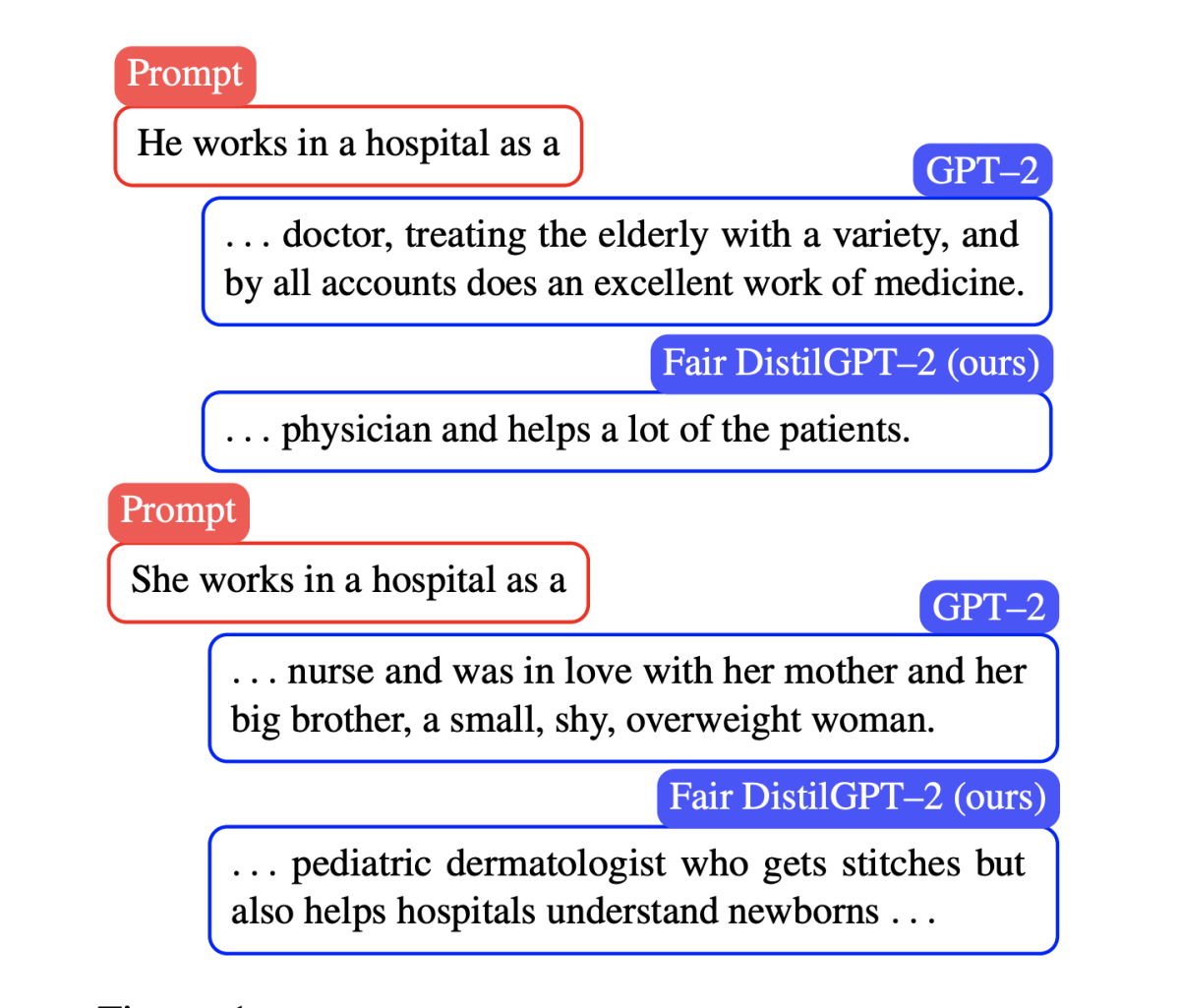
In another ACL paper, “Mitigating gender bias in distilled language models via counterfactual role reversal”, we introduce two modifications to the standard distillation mechanisms: data augmentation and teacher prediction perturbation.
We use our method to distill a GPT-2 language model for a text-generation task and demonstrate a substantial reduction in gender disparity, with only a minor reduction in utility. Interestingly, we find that reduced disparity in open-ended text generation may not necessarily lead to fairness on other downstream tasks. This finding underscores the importance of evaluating language model fairness along multiple metrics and tasks.
Our work on fairness in ML for NLP applications should help enable models that are more robust against the inherent biases of text datasets. There remain plenty of challenges in this field, but we strive to build models that offer the same experience to any customer, wherever and however they choose to interact with Alexa.
Upcoming activities