Training a machine learning model can be thought of as exploring a landscape that maps settings of the model parameters against average error rate. The goal of training is to find the bottom of the lowest basin in the landscape, or the parameter settings that yield the lowest error rate or “loss” value.
A critical hyperparameter during training is the learning rate, which determines how big an effect the learning from a given batch of training data can have on a model’s parameter settings. It’s common to vary the learning rate throughout training: for instance, we might use a high learning rate at the outset to rapidly explore the whole landscape but slow the learning rate over time to ensure that we don’t leap over a global minimum.
Varying the learning rate is known as learning-rate scheduling, and it’s instrumental in achieving stable convergence and maximum accuracy. Yet crafting optimal schedules often relies on painstaking trial-and-error experimentation. As models grow more complex, manual tuning becomes increasingly unscalable, and human-designed schedules fail to respond to intricate details of the loss landscape, model parameters, and dataset.
At Amazon, we are developing algorithms that can learn to schedule by harnessing data from past experiments. In a sequence of recent papers, we describe three phases of our research:
- Deriving stability guarantees for a simplified problem (non-negative-matrix factorization) and using them to develop a learnable scheduler;
- Extending that approach to deep neural networks; and
- Distilling the results into an efficient heuristic scheduler.
Analyzing stochastic non-negative-matrix factorization
In the first paper, “Efficient learning rate schedules for stochastic non-negative matrix factorization via reinforcement learning”, which we presented at ICLR 2023, we analyze stochastic non-negative-matrix factorization (NMF), a well-studied unsupervised-learning technique. NMF involves decomposing a non-negative matrix into two low-rank non-negative factor matrices.
Due to its popularity and mathematical simplicity, NMF served as an appealing testbed before we tackled more-complex models. Interestingly, our way of posing this well-studied matrix decomposition problem as a learning problem is related to the popular parameter-efficient fine-tuning (PEFT) methods that are used today for more-efficient compression and training of large language models.
In our first paper, we considered an optimization scheme for NMF that uses stochastic gradient descent — the standard machine learning algorithm — to minimize the difference between the original matrix and the matrix reconstituted from the factor matrices. To measure distance, we used the Frobenius norm, which is the square root of the sum of the squares of the individual differences for all matrix entries.
Assuming noisy gradients — that is, noisy estimations of slopes in the loss landscape — we established an upper bound for learning rates that guarantee stability, or convergence to a local minimum under repeated training epochs.
This yielded valuable insights. First, it quantified precisely how the learning rate controls trade-offs between convergence speed and potential divergence. Second, it showed that stability can be assured through proper learning rate initialization and clipping, or capping the extent to which any one model parameter can be modified during model updates.
With convergence guarantees in hand, we shifted our focus to learning what schedules may work well for specific problems. Reinforcement-learning (RL) agents search for and generate sequences of decisions that should lead to a better end state. This can be directly applied to learning-rate schedules that maximize convergence speed, while respecting stability bounds.
Empirically, the automated schedules our RL agent discovered consistently outperformed popular heuristics — such as step decay, which systematically lowers the learning rate after successive epochs — on NMF tasks. This provided a promising proof-of-concept for meta-learned scheduling in simplified domains where stability can be analytically assured.
Tackling deep-neural-network optimization
Given what we had learned about using RL for generating NMF schedules, we next sought to extend the adaptive-scheduling paradigm to deep neural networks. Unfortunately, deriving theoretical guarantees is vastly more difficult for complex nonconvex neural training objectives. Without assurances of stability, the optimization landscape becomes even more treacherous.
Nevertheless, in another 2023 ICLR paper, “Learned learning rate schedules for deep neural network training using reinforcement learning”, we hypothesized that data-driven scheduling could still improve on hand-tuned learning rates and schedules. We used the reinforcement-learning framework we’d developed for NMF to generate schedules for computer vision and natural-language-processing tasks.
The automated schedules successfully reduced training time and improved generalization compared to standard heuristics such as cosine annealing. This demonstrated the empirical viability of our approach even in the absence of stability guarantees. By learning online from data, the scheduler adapted to nuances of the loss landscape and gradient trajectories.
But using RL to find optimal schedules for this problem is still expensive — and it becomes more expensive as model and data sizes increase. So our next step was to distill our approach into a simple and usable algorithm.
The GreedyLR scheduler
At this year’s Conference on Pattern Recognition and Machine Learning (PRML), we won the best-presentation award for a lightweight learned scheduler called GreedyLR that sets the learning rate based on recent improvements in the training loss. In comparisons with popular scheduler and optimizer combinations, GreedyLR performed equivalently or better more than 90% of the time. It also enabled faster convergence than techniques like stochastic line search that adjust the learning rate by solving optimization problems during training.
In each training epoch, GreedyLR adapts the learning rate based on changes in the validation loss. Its core logic is simple: increase the learning rate if the loss improves and decrease it if the loss worsens. But GreedyLR employs additional techniques to make this greedy heuristic work well in practice:
- Its patience parameter prevents overreaction to noisy loss fluctuations.
- A smoothing window calculates the rolling-average validation loss for more-robust comparisons.
- Thresholds prevent needless updates when the loss change is insignificant.
- Cooldown and warmup stages continue increasing or decreasing the learning rate even if the loss trend reverses.
- Configurable upper and lower bounds on the learning-rate range enable it to benefit from human intuition without sacrificing the ability to explore counterintuitive methods.
Overall, these enhancements make GreedyLR respond intelligently to trends in the loss rather than reacting impulsively. The algorithm tunes the learning rate adaptively during training to accelerate convergence without compromising stability.
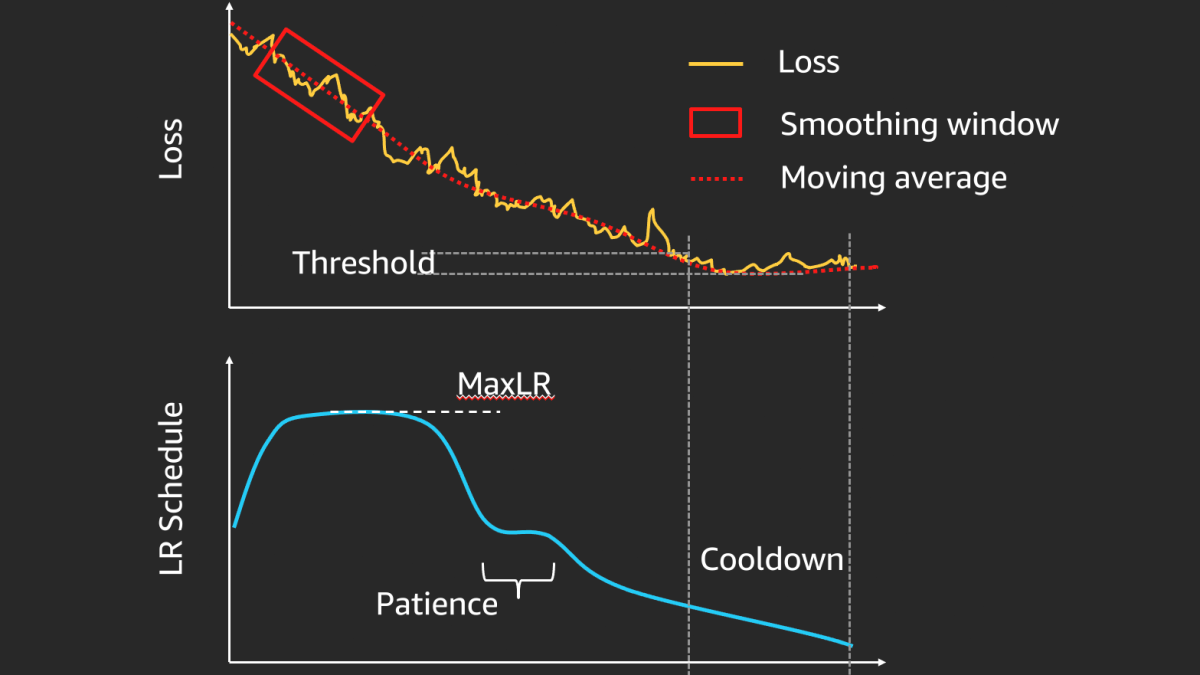
In our experiments, we found that GreedyLR is able to produce diverse, dynamic schedules, as shown in the figures below. Also shown below are standard schedules such as linear, constant, and cosine decay that are popular today:
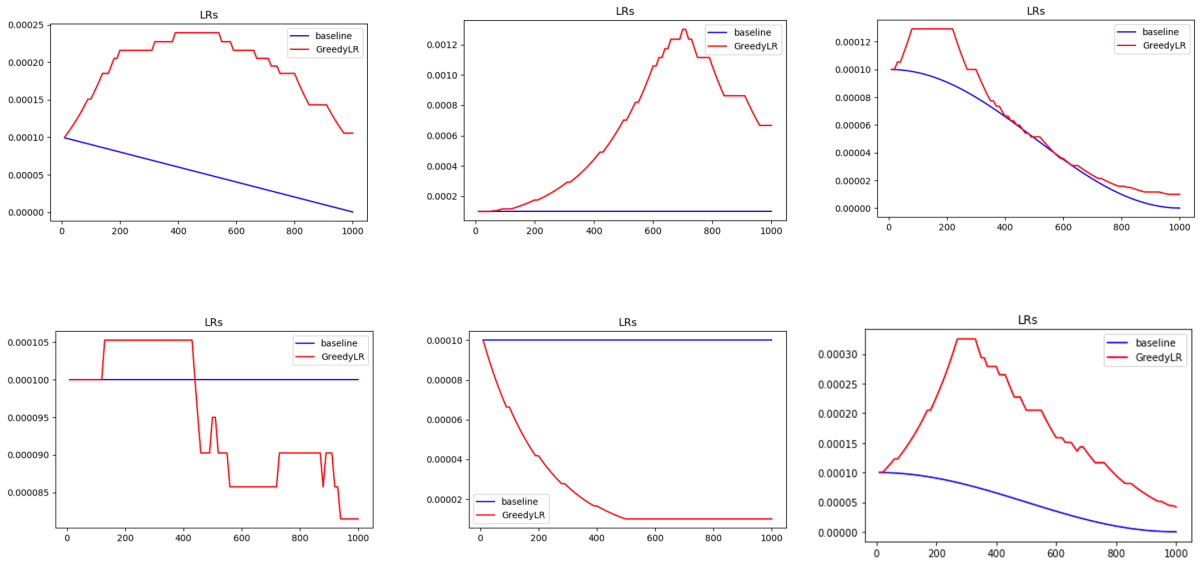
GreedyLR achieved faster convergence, especially for large models, making it a promising general-purpose scheduler. It also performed better than more-advanced methods such as hypergradient descent, which can be considered a first-order version of GreedyLR. While hypergradient descent tries to achieve faster convergence by using gradient descent to learn one learning rate per parameter or parameter group, GreedyLR just uses one global, reactive learning rate. This is particularly interesting since you need a billion learning rates for a billion-parameter model in hypergradient descent, versus a single learning rate for GreedyLR.
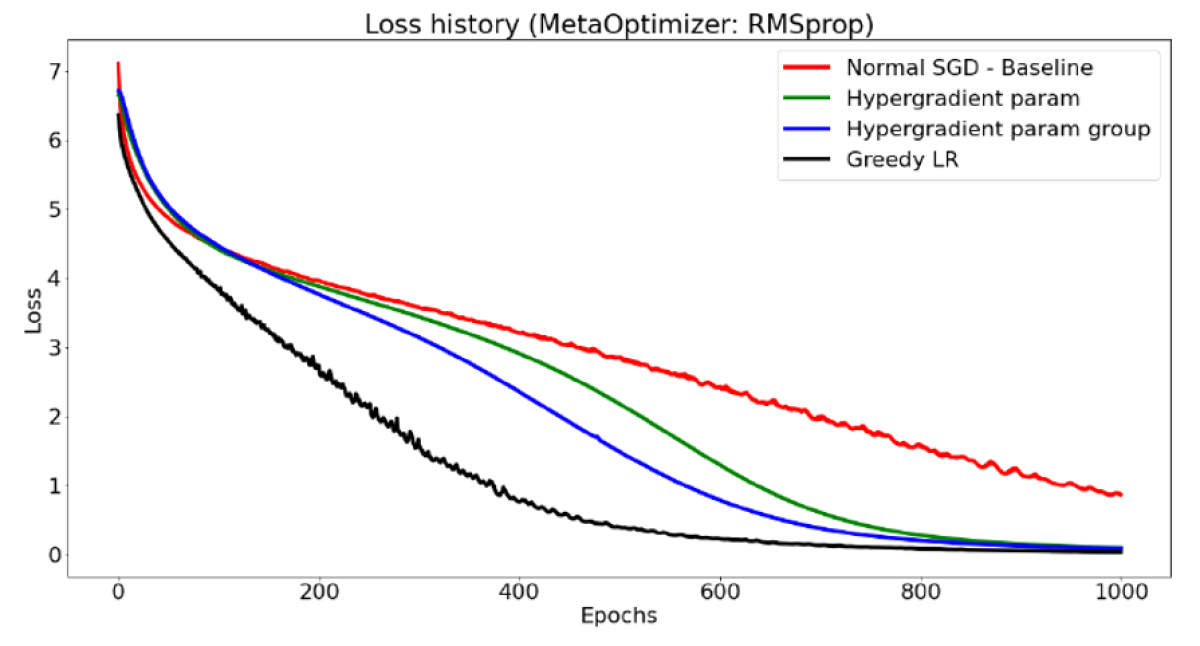
Conclusion and future outlook
Together, these contributions demonstrate the potential for learned optimizers to accelerate deep learning. By automatically adapting to training dynamics, they can find more-optimal solutions than human-designed algorithms reliant on rules of thumb. The ease of use and consistent gains from GreedyLR make it a compelling, general-purpose scheduler ready for wide adoption. We plan to continue improving the efficiency of our learning-based methods to further enhance productivity for deep-learning practitioners.