Dive into Deep Learning (D2L.ai) is an open-source textbook that makes deep learning accessible to everyone. It features interactive Jupyter notebooks with self-contained code in PyTorch, JAX, TensorFlow, and MXNet, as well as real-world examples, exposition figures, and math. So far, D2L has been adopted by more than 400 universities around the world, such as the University of Cambridge, Stanford University, the Massachusetts Institute of Technology, Carnegie Mellon University, and Tsinghua University.
The latest updates to "Dive into Deep Learning"
Learn about the newest additions to the popular open-source, interactive book, including the addition of a Google JAX implementation and three new chapters in volume 2.
As a result of the book’s widespread adoption, a community of contributors has formed to work on translations in various languages, including Chinese, Japanese, Korean, Portuguese, Turkish, and Vietnamese. To efficiently handle these multiple languages, we have developed the Auto Machine Translation and Synchronization (AMTS) system using Amazon Translate, which aims to reduce the workload of human translators by 80%. The AMTS can be applied to all the languages for translation, and each language-specific sub-AMTS pipeline has its own unique features based on language characteristics and translator preferences.
In this blog post, we will discuss how we build the AMTS framework architecture, its sub-pipelines, and the building blocks of the sub-pipeline. We will demonstrate and analyze the translations between two language pairs: English ↔ Chinese and English ↔ Spanish. Through these analyses, we will recommend best practices for ensuring translation quality and efficiency.
Framework overview
Customers can use Amazon Translate’s Active Custom Translation (ACT) feature to customize translation output on the fly by providing tailored translation examples in the form of parallel data. Parallel data consists of a collection of textual examples in a source language and the desired translations in one or more target languages. During translation, ACT automatically selects the most relevant segments from the parallel data and updates the translation model on the fly based on those segment pairs. This results in translations that better match the style and content of the parallel data.
The AMTS framework consists of multiple sub-pipelines, each of which handles one language translation — English to Chinese, English to Spanish, etc. Multiple translation sub-pipelines can be processed in parallel.
Fundamentally, the sub-pipeline consists of the following steps:
- Prepare parallel data: The parallel data consists of a list of textual example pairs, in a source language (e.g., English) and a target language (e.g., Chinese). With AMTS, we first prepare the two language datasets and then combine them into one-to-one pairs.
- Translate through batch jobs: We use the Amazon Translate API call CreateParallelData to import the input file from the Amazon Simple Storage Service (S3) and create a parallel-data resource in Amazon Translate, ready for batch translation jobs. With the parallel-data resource built in the last step, we customize Amazon Translate and use its asynchronous batch process operation to translate a set of documents in the source language in bulk. The translated documents in the target language are stored in Amazon S3.
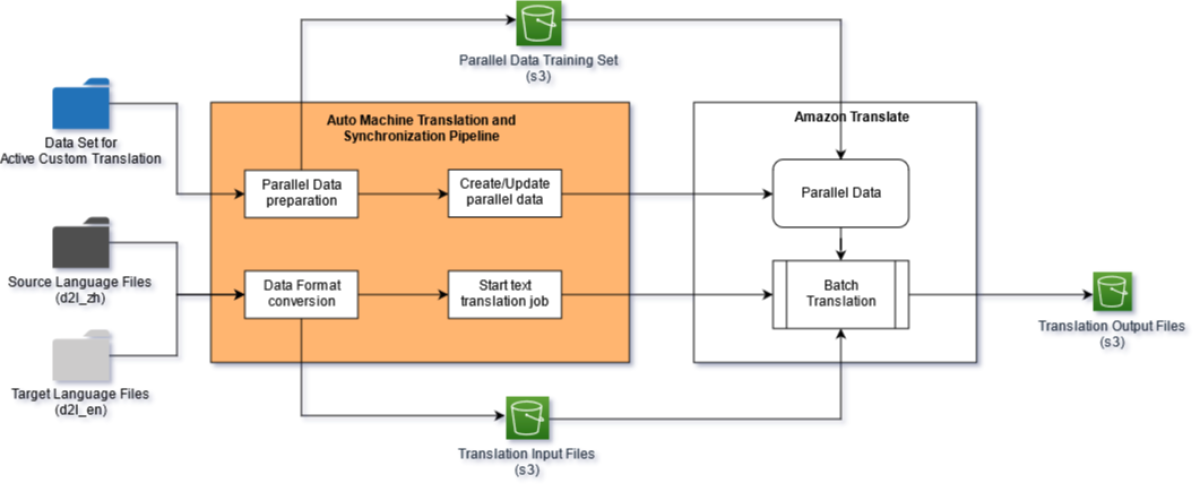
Parallel-data preparation and creation
In the parallel-data preparation step, we build the parallel-data set out of the source documents (sections of the D2L-enbook) and translations produced by professional human translators (e.g., parallel sections from the D2L-zh book). The software module extracts the text from both documents — ignoring code and picture blocks — and pairs them up, storing them in a CSV file. Examples of parallel data are shown in the table below.
English | Chinese |
Nonetheless, language models are of great service even in their limited form. For instance, the phrases "to recognize speech" and "to wreck a nice beach" sound very similar. This can cause ambiguity in speech recognition, which is easily resolved through a language model that rejects the second translation as outlandish. Likewise, in a document summarization algorithm it is worthwhile knowing that "dog bites man" is much more frequent than "man bites dog", or that "I want to eat grandma" is a rather disturbing statement, whereas "I want to eat, grandma" is much more benign. | 尽管如此,语言模型依然是非常有用的。例如,短语“to recognize speech”和“to wreck a nice beach”读音上听起来非常相似。这种相似性会导致语音识别中的歧义,但是这很容易通过语言模型来解决,因为第二句的语义很奇怪。同样,在文档摘要生成算法中,“狗咬人”比“人咬狗”出现的频率要高得多,或者“我想吃奶奶”是一个相当匪夷所思的语句,而“我想吃,奶奶”则要正常得多。 |
Machine translation refers to the automatic translation of a sequence from one language to another. In fact, this field may date back to 1940s soon after digital computers were invented, especially by considering the use of computers for cracking language codes in World War II. For decades, statistical approaches had been dominant in this field before the rise of end-to-end learning using neural networks. The latter is often called neural machine translation to distinguish itself from statistical machine translation that involves statistical analysis in components such as the translation model and the language model. | 机器翻译(machine translation)指的是将序列从一种语言自动翻译成另一种语言。事实上,这个研究领域可以追溯到数字计算机发明后不久的20世纪40年代,特别是在第二次世界大战中使用计算机破解语言编码。几十年来,在使用神经网络进行端到端学习的兴起之前,统计学方法在这一领域一直占据主导地位 |
Emphasizing end-to-end learning, this book will focus on neural machine translation methods. Different from our language model problem in the last section, whose corpus is in one single language, machine translation datasets are composed of pairs of text sequences that are in the source language and the target language, respectively. Thus, instead of reusing the preprocessing routine for language modeling, we need a different way to preprocess machine translation datasets. In the following, we show how to load the preprocessed data into mini batches for training. | 本书的关注点是神经网络机器翻译方法,强调的是端到端的学习。与 上节中的语料库是单一语言的语言模型问题存在不同,机器翻译的数据集是由源语言和目标语言的文本序列对组成的。因此,我们需要一种完全不同的方法来预处理机器翻译数据集,而不是复用语言模型的预处理程序。下面,我们看一下如何将预处理后的数据加载到小批量中用于训练 |
When the parallel data file is created and ready to use, we upload it to a folder in an S3 bucket and use CreateParallelData to kick off a creation job in Amazon Translate. If we only want to update an existing parallel-data resource with new inputs, the UpdateParallelData API call is the right one to make.
Once the job is completed, we can find the parallel-data resource in the Amazon Translate management console. The resource can be further managed in the AWS Console through the download, update, and delete buttons, as well as through AWS CLI and the public API.
Asynchronous batch translation with parallel data
After the parallel-data resource is created, the next step in the sub-pipeline is to use the Amazon Translate StartTextTranslationJob API call to initiate a batch asynchronous translation. The sub-pipeline uploads the source files into an Amazon S3 bucket folder.
One batch job can handle translation of multiple source documents, and the output files will be put in another S3 bucket folder. In addition to the input and output data configurations, the source language, target language, and prepared parallel-data resource are also specified as parameters in the API invocation.
src_lang = "en" tgt_lang = "zh" src_fdr = "input-short-test-en2zh" pd_name = "d2l-parallel-data_v2" response = translate_client.start_text_translation_job( JobName='D2L1', InputDataConfig={ 'S3Uri': 's3://'+S3_BUCKET+'/'+src_fdr+'/', 'ContentType': 'text/html' }, OutputDataConfig={ 'S3Uri': 's3://'+S3_BUCKET+'/output/', }, DataAccessRoleArn=ROLE_ARN, SourceLanguageCode=src_lang, TargetLanguageCodes=[tgt_lang, ], ParallelDataNames=pd_name )
Depending on the number of input files, the job takes minutes to hours to complete. We can find the job configurations and statuses, including the output file location, on the Amazon Translate management console.
The translated documents are available in the output S3 folder, with the filename <target language>.<source filename>. Users can download them and perform further evaluation.
Using parallel data yields better translation
To evaluate translation performance in each sub-pipeline, we selected five articles from the English version of D2L and translated them into Chinese through the en-zh sub-pipeline. Then we calculated the BLEU score of each translated document. The BLEU (BiLingual Evaluation Understudy) score calculates the similarity of the AMTS translated output to the reference translation by human translator. The number is between 0 and 1; the higher the score, the better the quality of the translation.
We then compare the AMTS-generated results with the translation of the same document using the traditional method (without parallel data). The traditional method is implemented by the TranslateText API call, whose parameters include the name of the source text and the source and target languages.
src_lang = "en" tgt_lang = "zh" response = translate_client.translate_text( Text = text, TerminologyNames = [], SourceLanguageCode = src_lang, TargetLanguageCode = tgt_lang )
The translation results are compared in the following table, for both English-to-Chinese and Chinese-to-English translation. We observe that the translation with parallel data shows improvement over the traditional method.
Article | EN to ZH | ZH to EN | ||
Without ACT | With ACT | Without ACT | With ACT | |
approx-training | 0.553 | 0.549 | 0.717 | 0.747 |
bert-dataset | 0.548 | 0.612 | 0.771 | 0.831 |
language-models-and-dataset | 0.502 | 0.518 | 0.683 | 0.736 |
machine-translation-and-dataset | 0.519 | 0.546 | 0.706 | 0.788 |
sentiment-analysis-and-dataset | 0.558 | 0.631 | 0.725 | 0.828 |
Average | 0.536 | 0.5712 | 0.7204 | 0.786 |
Fine-tuning the parallel data to improve translation quality
To further improve the translation quality, we construct the parallel-data pairs in a more granular manner. Instead of extracting parallel paragraphs from source and reference documents and pairing them up, we further split each paragraph into multiple sentences and use sentence pairs as training examples.
EN | ZH |
Likewise, in a document summarization algorithm it is worthwhile knowing that "dog bites man" is much more frequent than "man bites dog", or that "I want to eat grandma" is a rather disturbing statement, whereas "I want to eat, grandma" is much more benign | 同样,在文档摘要生成算法中,“狗咬人”比“人咬狗”出现的频率要高得多,或者“我想吃奶奶”是一个相当匪夷所思的语句,而“我想吃,奶奶”则要正常得多 |
For decades, statistical approaches had been dominant in this field before the rise of end-to-end learning using neural networks | 几十年来,在使用神经网络进行端到端学习的兴起之前,统计学方法在这一领域一直占据主导地位 |
In the following, we show how to load the preprocessed data into minibatches for training | 下面,我们看一下如何将预处理后的数据加载到小批量中用于训练 |
We tested both the paragraph pair and sentence pair methods and found that more-granular data (sentence pairs) yields better translation quality than less-granular data (paragraph paragraphs). The comparison is shown in the table below for English ↔ Chinese translation.
Article | EN to ZH | ZH to EN | ||
ACT by "pair of paragraph" | ACT by "pair of sentence" | ACT by "pair of paragraph" | ACT by "pair of sentence" | |
approx-training | 0.549 | 0.589 | 0.747 | 0.77 |
bert-dataset | 0.612 | 0.689 | 0.831 | 0.9 |
language-models-and-dataset | 0.518 | 0.607 | 0.736 | 0.806 |
machine-translation-and-dataset | 0.546 | 0.599 | 0.788 | 0.89 |
sentiment-analysis-and-dataset | 0.631 | 0.712 | 0.828 | 0.862 |
Average | 0.5712 | 0.6392 | 0.786 | 0.8456 |
Extend usage of parallel data to general machine translation
To extend the usability of parallel data to general machine translation, we need to construct parallel-data sets from a large volume of translated documents. To maximize translation accuracy, the parallel datasets should have the same contexts and subjects as the documents to be translated.
We tested this approach in the English ↔ Spanish sub-pipeline. The parallel data pairs were built from English ↔ Spanish articles crawled from the web using the keyword “machine learning”.
We applied this parallel data in translating an English article (abbreviated DLvsML in the results table) into Spanish and compared the results with those of traditional translation, without parallel data. The BLEU scores show that parallel data with the same subject (“machine learning”) does help to improve the performance of general machine translation.
EN to ES | ES to EN | |||
Without ACT | With ACT | Without ACT | With ACT | |
DLvsML | 0.792 | 0.824 | 0.809 | 0.827 |
The relative fluency of translations from English to Spanish, with and without ACT, can be seen in the table below.
EN source text | ES reference text (human translation) | ES translation without ACT | ES translation with ACT |
Moves through the learning process by resolving the problem on an end-to-end basis. | Pasa por el proceso de aprendizaje mediante la resolución del problema de un extremo a otro. | Avanza en el proceso de aprendizaje resolviendo el problema de un extremo a otro. | Avanza el proceso de aprendizaje resolviendo el problema de forma integral. |
Deep learning use cases | Casos de uso del aprendizaje profundo | Casos de uso de aprendizaje profundo | Casos prácticos de aprendizaje profundo |
Image caption generation | Generación de subtítulos para imágenes | Generación de leyendas de imágenes | Generación de subtítulos de imagen |
Conclusion and best practices
In this post, we introduced the Auto Machine Translation and Synchronization (AMTS) framework and pipelines and their application to English ↔ Chinese and English ↔ Spanish D2L.ai auto-translation. We also discussed best practices for using the Amazon Translate service in the translation pipeline, particularly the advantages of the Active Custom Translation (ACT) feature with parallel data.
- Leveraging the Amazon Translate service, the AMTS pipeline provides fluent translations. Informal qualitative assessments suggest that the translated texts read naturally and are mostly grammatically correct.
- In general, the ACT feature with parallel data improves translation quality in the AMTS sub-pipeline. We show that using the ACT feature leads to better performance than using the traditional Amazon Translate real-time translation service.
- The more granular the parallel data pairs are, the better the translation performance. We recommend constructing the parallel data as pairs of sentences, rather than pairs of paragraphs.
We are working on further improving the AMTS framework to improve translation quality for other languages. Your feedback is always welcome.