At this year’s Quantum Information Processing Conference (QIP), members of Amazon Web Services' Quantum Technologies group are coauthors on three papers, which indicate the breadth of the group’s research interests.
In “Mind the gap: Achieving a super-Grover quantum speedup by jumping to the end”, Amazon research scientist Alexander Dalzell, Amazon quantum research scientist Nicola Pancotti, Earl Campbell of the University of Sheffield and Riverlane, and I present a quantum algorithm that improves on the efficiency of Grover’s algorithm, one of the few quantum algorithms to offer provable speedups relative to conventional algorithms. Although the improvement on Grover’s algorithm is small, it breaks a performance barrier that hadn’t previously been broken, and it points to a methodology that could enable still greater improvements.
In “A streamlined quantum algorithm for topological data analysis with exponentially fewer qubits”, Amazon research scientist Sam McArdle, Mario Berta of Aachen University, and András Gilyén of the Alfréd Rényi Institute of Mathematics in Budapest consider topological data analysis, a technique for analyzing big data. They present a new quantum algorithm for topological data analysis that, compared to the existing quantum algorithm, enables a quadratic speedup and an exponentially more efficient use of quantum memory.
For “Sparse random Hamiltonians are quantumly easy”, Chi-Fang (Anthony) Chen, a Caltech graduate student who was an Amazon intern when the work was done, won the conference's best-student-paper award. He's joined on the paper by Alex Dalzell and me, Mario Berta, and Caltech's Joel Tropp. The paper investigates the use of quantum computers to simulate physical properties of quantum systems. We prove that a particular model of physical systems — specifically, sparse, random Hamiltonians — can, with high probability, be efficiently simulated on a quantum computer.
Super-Grover quantum speedup
Grover’s algorithm is one of the few quantum algorithms that are known to provide speedups relative to classical computing. For instance, for the 3-SAT problem, which involves finding values for N variables that satisfy the constraints of an expression in formal logic, the running time of a brute-force classical algorithm is proportional to 2N; the running time of Grover’s algorithm is proportional to 2N/2.
Adiabatic quantum computing is an approach to quantum computing in which a quantum system is prepared so that, in its lowest-energy state (the “ground state”), it encodes the solution to a relatively simple problem. Then, some parameter of the system — say, the strength of a magnetic field — is gradually changed, so that the system encodes a more complex problem. If the system stays in its ground state through those changes, it will end up encoding the solution to the complex problem.
As the parameter is changed, however, the gaps between the system’s ground state and its first excited states vary, sometimes becoming infinitesimally small. If the parameter changes too quickly, the system may leap into one of its excited states, ruining the computation.
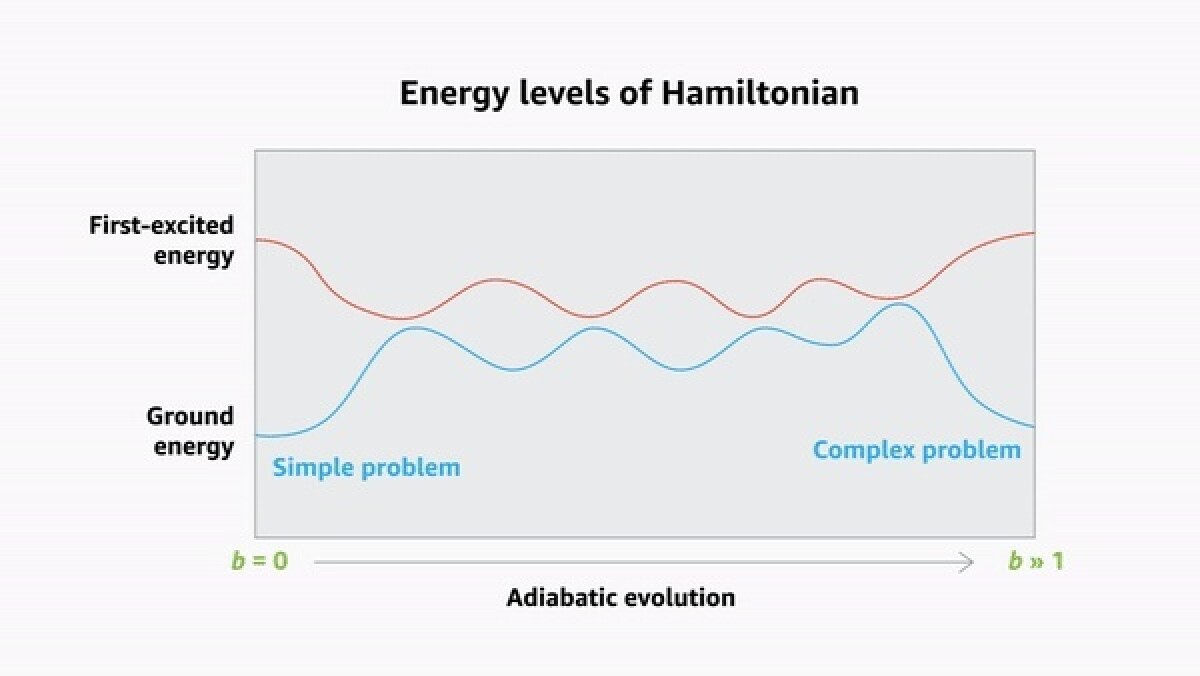
In “Mind the gap: Achieving a super-Grover quantum speedup by jumping to the end”, we show that for an important class of optimization problems, it’s possible to compute an initial jump in the parameter setting that runs no risk of kicking the system into a higher energy state. Then, a second jump takes the parameter all the way to its maximum value.
Most of the time this will fail, but every once in a while, it will work: the system will stay in its ground state, solving the problem. The larger the initial jump, the greater the increase in success rate.
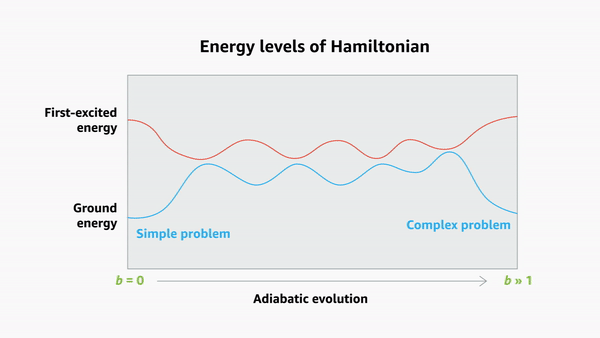
Our paper proves that the algorithm has an infinitesimal but quantifiable advantage over Grover’s algorithm, and it reports a set of numerical experiments to determine the practicality of the approach. Those experiments suggest that the method, in fact, increases efficiency more than could be mathematically proven, although still too little to yield large practical benefits. The hope is that the method may lead to further improvements that could make a practical difference to quantum computers of the future.
Topological data analysis
Topology is a branch of mathematics that treats geometry at a high level of abstraction: on a topological description, any two objects with the same number of holes in them (say, a coffee cup and a donut) are identical.
Mapping big data to a topological object — or manifold — can enable analyses that are difficult at lower levels of abstraction. Because topological descriptions are invariant to shape transformations, for instance, they are robust against noise in the data.
Topological data analysis often involves the computation of persistent Betti numbers, which characterize the number of holes in the manifold, a property that can carry important implications about the underlying data. In “A streamlined quantum algorithm for topological data analysis with exponentially fewer qubits”, the authors propose a new quantum algorithm for computing persistent Betti numbers. It offers a quadratic speedup relative to classical algorithms and uses quantum memory exponentially more efficiently than existing quantum algorithms.
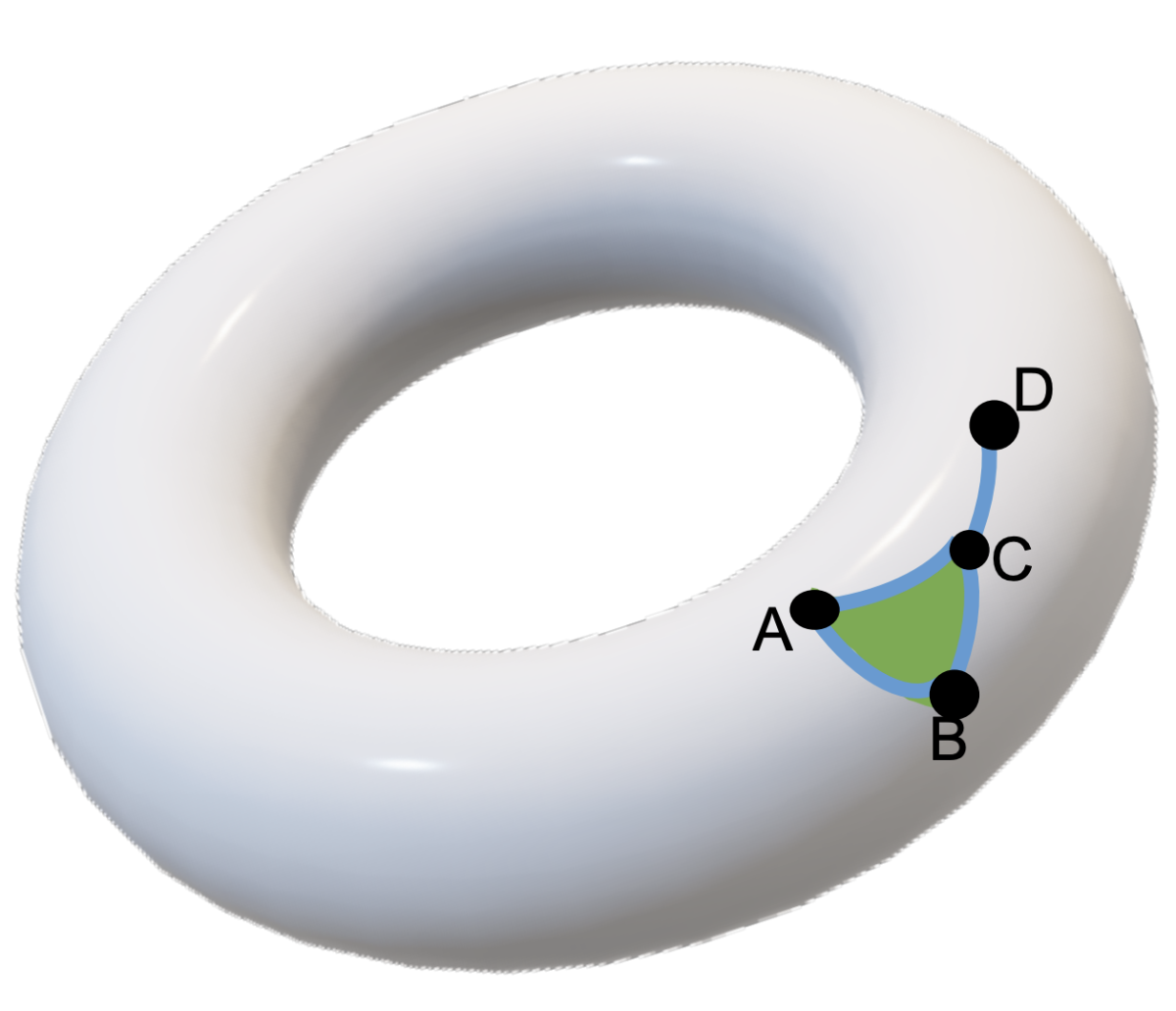
Data can be represented as points in a multidimensional space, and topological mapping can be thought of as drawing line segments between points in order to produce a surface, much the way animators create mesh outlines of 3-D objects. The maximum length of the lines defines the length scale of the mapping.
At short enough length scales, the data would be mapped to a large number of triangles, tetrahedra, and their higher-dimensional analogues, which are known as simplices. As the length scale increases, simplices link up to form larger complexes, and holes in the resulting manifold gradually disappear. The persistent Betti number is the number of holes that persist across a range of longer length scales.
The researchers’ chief insight is, though the dimension of the representational space may be high, in most practical cases, the dimension of the holes is much lower. The researchers define a set of boundary operators, which find the boundaries (e.g., the surfaces of 3-D shapes) of complexes (combinations of simplices) in the representational space. In turn, the boundary operators (or more precisely, their eigenvectors) provide a new geometric description of the space, in which regions of the space are classified as holes or not-holes.
Since the holes are typically low dimensional, so is the space, which enables the researchers to introduce an exponentially more compact mapping of simplices to qubits, dramatically reducing the spatial resources required for the algorithm.
Sparse random Hamiltonians
The range of problems on which quantum computing might enable useful speedups, compared to classical computing, is still unclear. But one area where quantum computing is likely to offer advantages is in the simulation of quantum systems, such as molecules. Such simulations could yield insights in biochemistry and materials science, among other things.
Often, in quantum simulation, we're interested in quantum systems' low-energy properties. But in general, it’s difficult to prove that a given quantum algorithm can prepare a quantum system in a low-energy state.
The energy of a quantum system is defined by its Hamiltonian, which can be represented as a matrix. In “Sparse random Hamiltonians are quantumly easy”, we show that for almost any Hamiltonian matrix that is sparse — meaning it has few nonzero entries — and random — meaning the locations of the nonzero entries are randomly assigned — it is possible to prepare a low-energy state.
Moreover, we show that the way to prepare such a state is simply to initialize the quantum memory that stores the model to a random state (known as preparing a maximally mixed state).
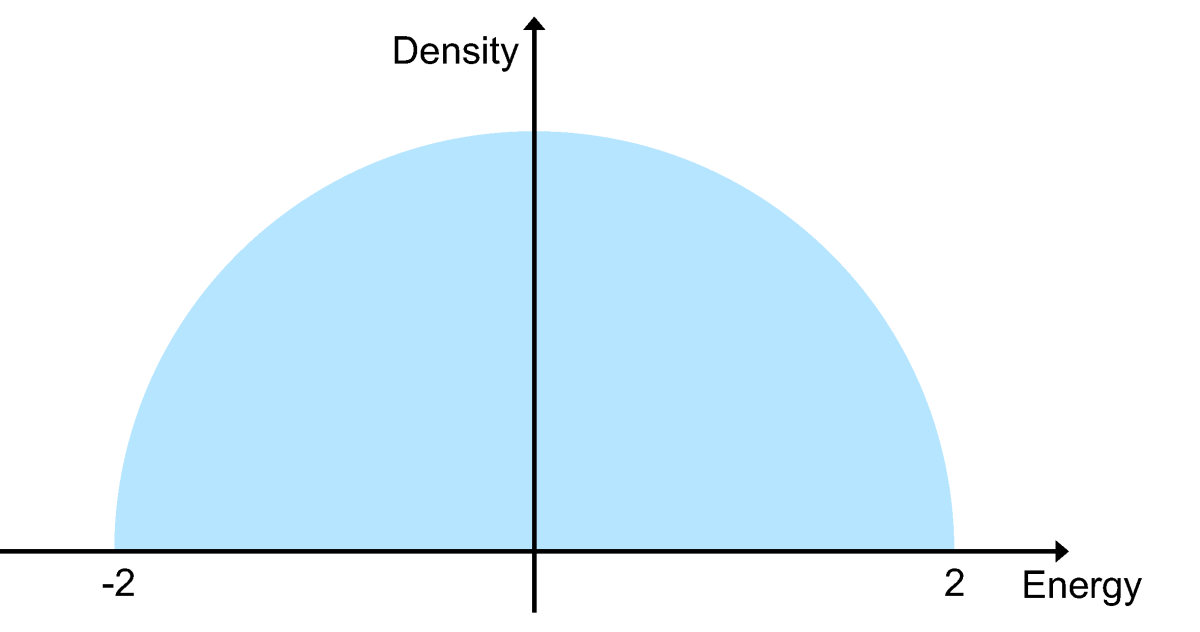
The key to our proof is to generalize a well-known result for dense matrices — Wigner's semicircle distribution for Gaussian unitary ensembles (GUEs) — to sparse matrices. Computing the energy level of a quantum system from its Hamiltonian involves calculating the eigenvalues of the Hamiltonian matrix, a standard operation in linear algebra. Wigner showed that the eigenvalues of random dense matrices form a semicircular distribution. That is, the possible eigenvalues of random matrices don’t trail off to infinity in a long tail; instead, they have sharp demarcation points. There are no possible values above and below some clearly defined thresholds.
Dense Hamiltonians, however, are rare in nature. The Hamiltonians describing most of the physical systems that physicists and chemists care about are sparse. By showing that sparse Hamiltonians conform to the same semicircular distribution that dense Hamiltonians do, we prove that the number of experiments required to measure a low-energy state of a quantum simulation will not proliferate exponentially.
In the paper, we also show that any low-energy state must have non-negligible quantum circuit complexity, suggesting that it could not be computed efficiently by a classical computer — an argument for the necessity of using quantum computers to simulate quantum systems.