In June, Amazon Web Services (AWS) announced that John Preskill, the Richard P. Feynman Professor of Theoretical Physics at the California Institute of Technology, an advisor to the National Quantum Initiative, and one of the most respected researchers in the field of quantum information science, would be joining Amazon’s quantum computing research effort as an Amazon Scholar.
Quantum computing is an emerging technology with the potential to deliver large speedups — even exponential speedups — over classical computing on some computational problems.
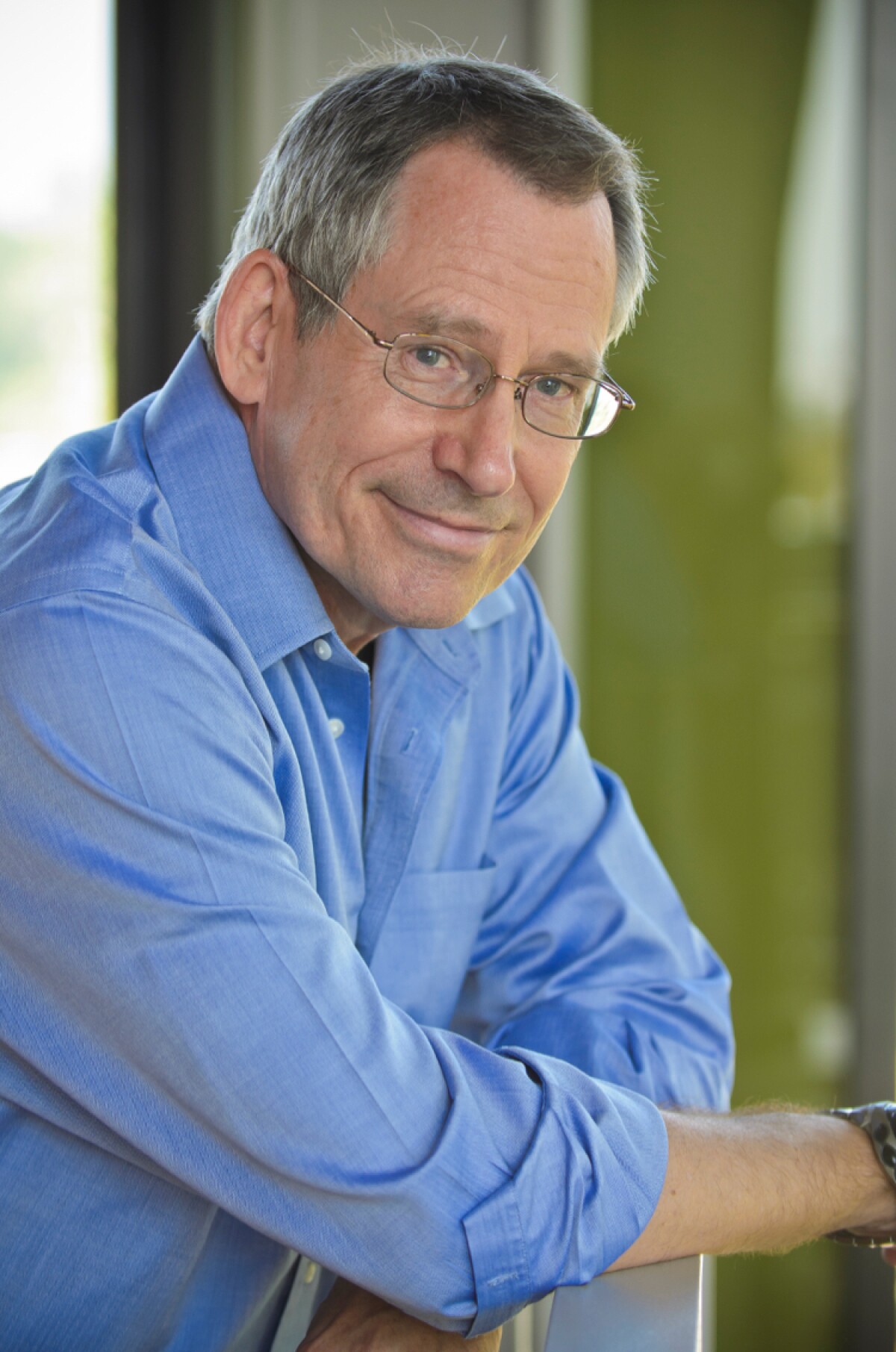
Where a bit in an ordinary computer can take on the values 0 or 1, a quantum bit, or qubit, can take on the values 0, 1, or, in a state known as superposition, a combination of the two. Quantum computing depends on preserving both superposition and entanglement, a fragile condition in which the qubits’ quantum states are dependent on each other.
The goal of the AWS Center for Quantum Computing, on the Caltech campus, is to develop and build quantum computing technologies and deliver them onto the AWS cloud. At the center, Preskill will be joining his Caltech colleagues Oskar Painter and Fernando Brandao, the heads of AWS’s Quantum Hardware and Quantum Algorithms programs, respectively, and Gil Refael, the Taylor W. Lawrence Professor of Theoretical Physics at Caltech and, like Preskill, an Amazon Scholar.
Other Amazon Scholars contributing to the AWS quantum computing effort are Amir Safavi-Naeini, an assistant professor of applied physics at Stanford University, and Liang Jiang, a professor of molecular engineering at the University of Chicago.
Amazon Science asked Preskill three questions about the challenges of quantum computing and why he’s excited about AWS’s approach to meeting them.
Q: Why is quantum computing so hard?
What makes it so hard is we want our hardware to simultaneously satisfy a set of criteria that are nearly incompatible.
On the one hand, we need to keep the qubits almost perfectly isolated from the outside world. But not really, because we want to control the computation. Eventually, we’ve got to measure the qubits, and we've got to be able to tell them what to do. We're going have to have some control circuitry that determines what actual algorithm we’re running.
So why is it so important to keep them isolated from the outside world? It's because a very fundamental difference between quantum information and ordinary information expressed in bits is that you can't observe a quantum state without disturbing it. This is a manifestation of the uncertainty principle of quantum mechanics. Whenever you acquire information about a quantum state, there's some unavoidable, uncontrollable disturbance of the state.
So in the computation, we don't want to look at the state until the very end, when we're going to read it out. But even if we're not looking at it ourselves, the environment is looking at it. If the environment is interacting with the quantum system that encodes the information that we're processing, then there's some leakage of information to the outside, and that means some disturbance of the quantum state that we're trying to process.
Explore our new quantum technologies research section
Quantum computing has the potential to solve computational problems that are beyond the reach of today's classical computers. Find the latest quantum news, research papers, and more.
So really, we need to keep the quantum computer almost perfectly isolated from the outside world, or else it's going to fail. It's going to have errors. And that sounds ridiculously hard, because hardware is never going to be perfect. And that's where the idea of quantum error correction comes to the rescue.
The essence of the idea is that if you want to protect the quantum information, you have to store it in a very nonlocal way by means of what we call entanglement. Which is, of course, the origin of the quantum computer’s magic to begin with. A highly entangled state has the property that when you have the state shared among many parts of a system, you can look at the parts one at a time, and that doesn't reveal any of the information that is carried by the system, because it's really stored in these unusual nonlocal quantum correlations among the parts. And the environment interacts with the parts kind of locally, one at a time.
If we store the information in the form of this highly entangled state, the environment doesn't find out what the state is. And that's why we're able to protect it. And we've also figured out how to process information that's encoded in this very entangled, nonlocal way. That's how the idea of quantum error correction works. What makes it expensive is in order to get very good protection, we have to have the information shared among many qubits.
Q: Today’s error correction schemes can call for sharing the information of just one logical qubit — the one qubit actually involved in the quantum computation — across thousands of additional qubits. That sounds incredibly daunting, if your goal is to perform computations that involve dozens of logical qubits.
Well, that's why, as much as we can, we would like to incorporate the error resistance into the hardware itself rather than the software. The way we usually think about quantum error correction is we’ve got these noisy qubits — it's not to disparage them or anything: they're the best qubits we've got in a particular platform. But they're not really good enough for scaling up to solving really hard problems. So the solution which at least theoretically we know should work is that we use a code. That is, the information that we want to protect is encoded in the collective state of many qubits instead of just the individual qubits.
We're interested in what is fundamentally different between classical systems and quantum systems. And I don't know a statement that more dramatically expresses the difference than saying that there are problems that are easy quantumly and hard classically.
But the alternative approach is to try to use error correction ideas in the design of the hardware itself. Can we use an encoding that has some kind of intrinsic noise resistance at the physical level?
The original idea for doing this came from one of my Caltech colleagues, Alexei Kitaev, and his idea was that you could just design a material that sort of has its own strong quantum entanglement. Now people call these topological materials; what's important about them is they're highly entangled. And so the information is spread out in this very nonlocal way, which makes it hard to read the information locally.
Making a topological material is something people are trying to do. I think the idea is still brilliant, and maybe in the end it will be a game-changing idea. But so far it's just been too hard to make the materials that have the right properties.
A better bet for now might be to do something in-between. We want to have some protection at the hardware level, but not go as far as these topological materials. But if we can just make the error rate of the physical qubits lower, then we won't need so much overhead from the software protection on top.
Q: For a theorist like you, what’s the appeal of working on a project whose goal is to develop new technologies?
My training was in particle physics and cosmology, but in the mid-nineties, I got really excited because I heard about the possibility that if you could build a quantum computer, you could factor large numbers. As physicists, of course, we're interested in what is fundamentally different between classical systems and quantum systems. And I don't know a statement that more dramatically expresses the difference than saying that there are problems that are easy quantumly and hard classically.
The situation is we don't know much about what happens when a quantum system is very profoundly entangled, and the reason we don't know is because we can't simulate it on our computers. Our classical computers just can't do it. And that means that as theorists, we don't really have the tools to explain how those systems behave.
I have done a lot of work on these quantum error correcting codes. It was one of my main focuses for almost 15 years. There were a lot of issues of principle that I thought were important to address. Things like, What do you really need to know about noise for these things to work? This is still an important question, because we had to make some assumptions about the noise and the hardware to make progress.
I said the environment looks at the system locally, sort of one part at a time. That's actually an assumption. It's up to the environment to figure out how it wants to look at it. As physicists, we tend to think physics is kind of local, and things interact with other nearby things. But until we’re actually doing it in the lab, we won't really be sure how good that assumption is.
So this is the new frontier of the physical sciences, exploring these more and more complex systems of many particles interacting quantum mechanically, becoming highly entangled. Sometimes I call it the entanglement frontier. And I'm excited about what we can learn about physics by exploring that. I really think in AWS we are looking ahead to the big challenges. I'm pretty jazzed about this.
On November 2, 2020, John Preskill joined Simone Severini, the director of AWS Quantum Computing, for an interview with Simon Elisha, host of the Official AWS Podcast.