Customer-obsessed science
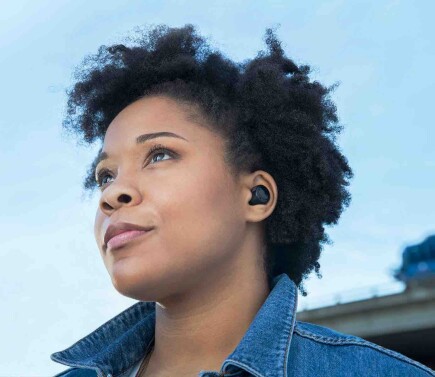
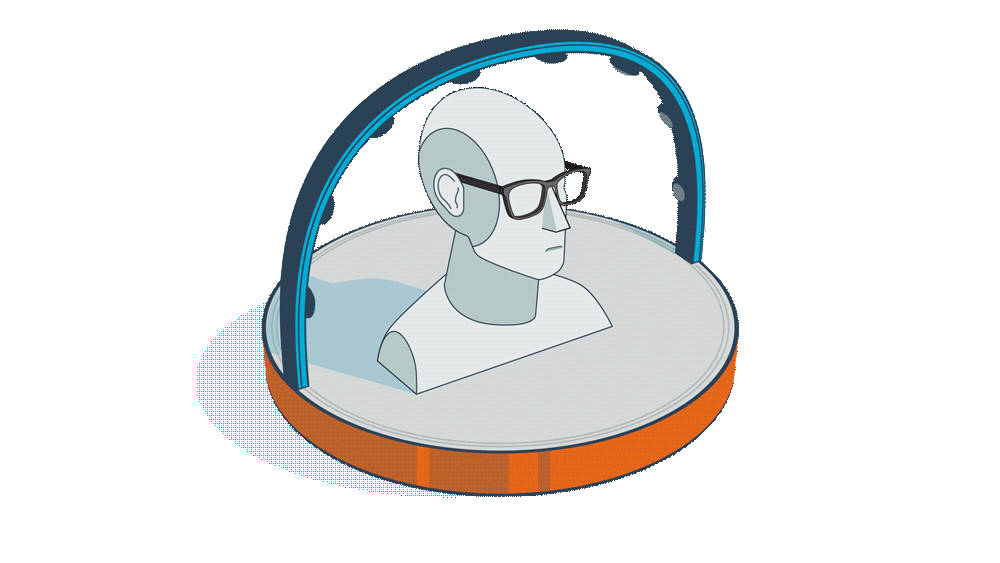
Research areas
-
July 29, 2025New cost-to-serve-software metric that accounts for the full software development lifecycle helps determine which software development innovations provide quantifiable value.
Featured news
-
Enterprise accounting data is complex, ambiguous, and shaped by evolving systems and regulations. The institutional knowledge needed to reason over the data is sparse, scattered and rarely structurally documented—posing major challenges for LLM agents. We introduce a multi-agent financial research framework that mimics a junior analyst’s onboarding and growth. The Analyst Agent learns proactively from repeated
-
2025In this paper, we investigate the problem of quantifying fairness in Retrieval-Augmented Generation (RAG) systems, particularly for complex cognitive tasks that go beyond factual question-answering. While RAG systems have demonstrated effectiveness in information extraction tasks, their fairness implications for cognitively complex tasks - including ideation, content creation, and analytical reasoning —
-
Speculative decoding has emerged as a promising approach to accelerating large language model (LLM) generation using a fast drafter while maintaining alignment with the target model’s distribution. However, existing approaches face a tradeoff: external drafters offer flexibility but can suffer from slower drafting, while self-speculation methods use drafters tailored to the target model but require re-training
-
As large language models increasingly gain popularity in real-world applications, processing extremely long contexts, often exceeding the model’s pre-trained context limits, has emerged as a critical challenge. While existing approaches to efficient long-context processing show promise, recurrent compression-based methods struggle with information preservation, whereas random access approaches require substantial
-
2025Diffusion Policies are effective at learning closed-loop manipulation policies from human demonstrations but generalize poorly to novel arrangements of objects in 3D space, hurting real-world performance. To address this issue, we propose Spherical Diffusion Policy (SDP), an SE(3) equivariant diffusion policy that adapts trajectories according to 3D transformations of the scene. Such equivariance is achieved
Academia
View allWhether you're a faculty member or student, there are number of ways you can engage with Amazon.
View all