Customer-obsessed science
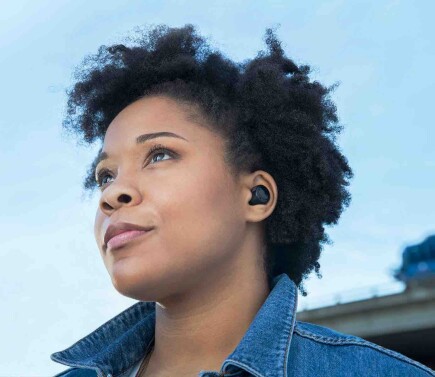
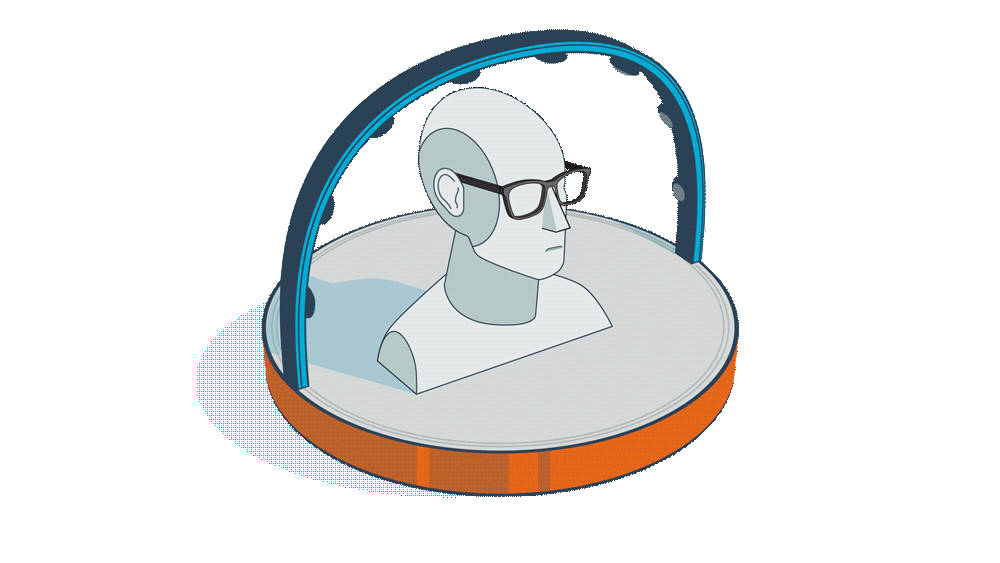
Research areas
-
April 11, 2025Novel three-pronged approach combines claim-level evaluations, chain-of-thought reasoning, and classification of hallucination error types.
-
-
Featured news
-
2025Jailbreaking large-language models (LLMs) involves testing their robustness against adversarial prompts and evaluating their ability to withstand prompt attacks that could elicit unauthorized or malicious responses. In this paper, we present TurboFuzzLLM, a mutation-based fuzzing technique for efficiently finding a collection of effective jailbreaking templates that, when combined with harmful questions
-
CVPR 2025 Workshop on Computer Vision in Sports2025Vision Language Models (VLMs) have demonstrated strong performance in multi-modal tasks by effectively aligning visual and textual representations. However, most video understanding VLM research has been domain-agnostic, leaving the understanding of their transfer learning capability to specialized domains under-explored. In this work, we address this by exploring the adaptability of open-source VLMs to
-
SIGMOD/PODS 20252025Compute elasticity is a primary benefit of using cloud-based data processing platforms such as Amazon EMR, where clusters can be scaled both horizontally and vertically. For example, a query scanning petabytes of data can run faster in a cluster with thousands of nodes compared to one with only a few hundred. However, not all workloads require the same computational power or have the same resource utilization
-
AAAI 2025 Workshop on Advancing LLM-Based Multi-Agent Collaboration2025Large Language Models (LLMs) have revolutionized AI-generated content evaluation, with the LLM-as-a-Judge paradigm becoming increasingly popular. However, current single-LLM evaluation approaches face significant challenges, including inconsistent judgments and inherent biases from pre-training data. To address these limitations, we propose CollabEval, a novel multi-agent evaluation framework that implements
-
2025Traditional segmentation models, while effective in isolated tasks, often fail to generalize to more complex and open-ended segmentation problems, such as free-form, open-vocabulary, and in-the-wild scenarios. To bridge this gap, we propose to scale up image segmentation across diverse datasets and tasks such that the knowledge across different tasks and datasets can be integrated while improving the generalization
Academia
View allWhether you're a faculty member or student, there are number of ways you can engage with Amazon.
View all