Customer-obsessed science
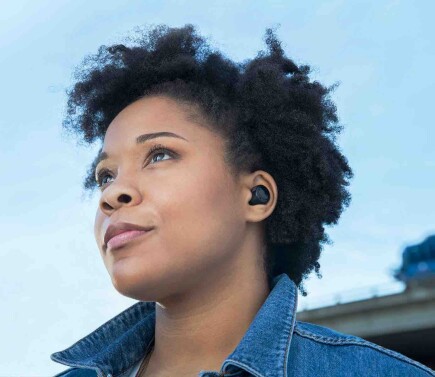
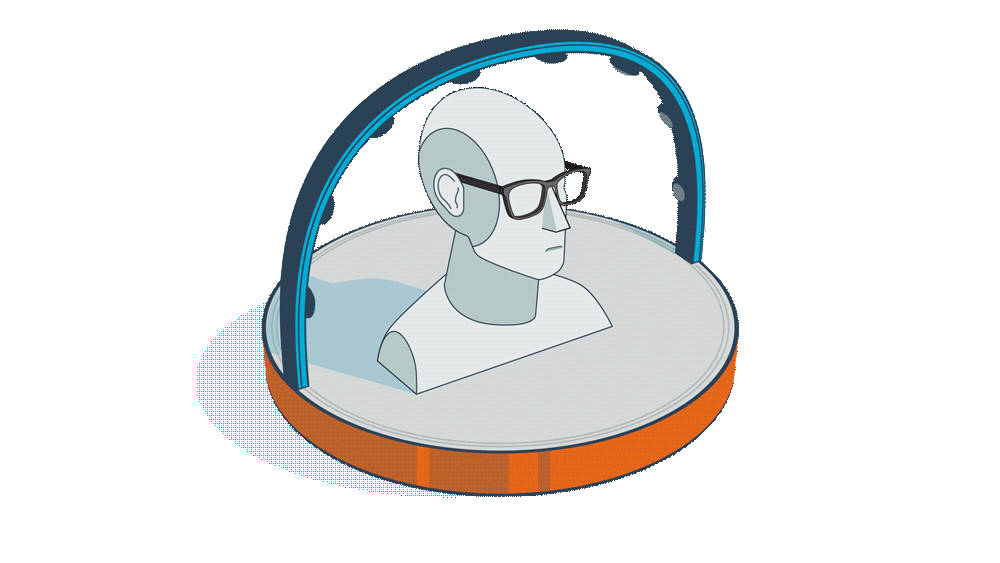
Research areas
-
February 27, 2025Prototype is the first realization of a scalable, hardware-efficient quantum computing architecture based on bosonic quantum error correction.
Featured news
-
NeurIPS 2024 Workshop on Tackling Climate Change with Machine Learning, Environmental Science and Technology2025Accurately quantifying greenhouse gas (GHG) emissions is crucial for organizations to measure and mitigate their environmental impact. Life cycle assessment (LCA) estimates the environmental impacts throughout a product’s entire lifecycle, from raw material extraction to end-of-life. Measuring the emissions outside of a product owner’s control is challenging, and practitioners rely on emission factors (
-
2025Composed Image Retrieval (CIR) is a complex task that aims to retrieve images based on a multimodal query. Typical training data consists of triplets containing a reference image, a textual description of desired modifications, and the target image, which are expensive and time-consuming to acquire. The scarcity of CIR datasets has led to zero-shot approaches utilizing synthetic triplets or leveraging vision-language
-
2025Open domain question answering systems frequently rely on information retrieved from large collections of text (such as the Web) to answer questions. However, such collections of text often contain conflicting information, and indiscriminately depending on this information may result in untruthful and inaccurate answers. To understand the gravity of this problem, we collect a human-annotated dataset, Question
-
AISTATS 20252025We study the well-motivated problem of online distribution shift in which the data arrive in batches and the distribution of each batch can change arbitrarily over time. Since the shifts can be large or small, abrupt or gradual, the length of the relevant historical data to learn from may vary over time, which poses a major challenge in designing algorithms that can automatically adapt to the best “attention
-
2025We introduce AnoLLM, a novel framework that leverages large language models (LLMs) for unsupervised tabular anomaly detection. By converting tabular data into a standardized text format, we further adapt a pre-trained LLM with this serialized data, and assign anomaly scores based on the negative log likelihood generated by the LLM. Unlike traditional methods that can require extensive feature engineering
Academia
View allWhether you're a faculty member or student, there are number of ways you can engage with Amazon.
View all