Clouds are famously hard to pin down. This is problematic in a world in which climate science is key to our future existence, and it is high time clouds coughed up their secrets. So it is fortunate that advances in machine learning and — no pun intended — cloud computing are finally starting to dissipate their mystery.
“The combination of machine learning and climate sciences is about to really take off,” says Philip Stier.
And he should know. A professor of atmospheric physics, Stier is head of Atmospheric, Oceanic and Planetary Physics at the University of Oxford, where he also leads the Climate Processes Group. “The role of clouds remains one of the biggest uncertainties in climate science: how clouds respond to changes in pollution and to warming itself,” says Stier, who is dedicating his career to tackling this uncertainty.
To that end, in 2018 Stier’s group was granted an Amazon Machine Learning Research Award to support the work of a new program called iMIRACLI (innovative MachIne leaRning to constrain Aerosol-cloud CLimate Impacts), a European Union-funded graduate program that Stier leads, designed to bring together pioneering climate scientists, machine learning experts, and industry partners to help build the next generation of climate data scientists. “We are creating a new cohort of people that are literate in both climate science and data science,” says Stier.
The idea is that a merging of AI, ML, and climate science can deliver breakthroughs in our understanding of the impact on climate of aerosol-cloud interactions. This is crucial because clouds reflect the sun’s heat back into space, producing a cooling effect. And because cloud droplets can only coalesce around atmospheric aerosols — be they natural aerosols or emitted by human activity — more aerosols mean brighter, more reflective clouds.
Truly big data
As a physicist and climate scientist, Stier works with big data worthy of the name.
“Machine learning people often say, ‘Oh yeah, we work with big data,’ but the climate data sets we have are truly massive. We're talking about satellites downlinking terabytes per day, and we must make sense of it all.”
But the complex nature of cloud behavior creates significant uncertainties in climate models. “We understand the greenhouse effect very well. With respect to the radiative forcing — warming — caused by greenhouse gases, particularly CO2, the uncertainty is relatively small,” says Stier. “The effects of atmospheric aerosols are much more uncertain, partly because you need to know not only the composition or the concentration, but also the particle size, shape and so on.” Aerosols are also short-lived compared with CO2, typically lasting a week before falling out of the sky, while being continually replaced by ongoing emissions.
Air pollution kills millions of people every year, so we want to get rid of it, but there is a risk that by cleaning up air pollution we will accelerate global warming — it’s a moral dilemma.
Trying to model and predict the cooling effect of clouds is extremely tough, but Stier and his colleagues estimate that the aerosols pumped into the atmosphere by human activity are currently offsetting between a fifth and a half of the warming caused by greenhouse gases. “It is important to accurately quantify this cooling effect. Air pollution kills millions of people every year, so we want to get rid of it, but there is a risk that by cleaning up air pollution we will accelerate global warming — it’s a moral dilemma.”
The iMIRACLI team is taking several approaches, utilizing the power of machine learning. One early strand of research involved a phenomenon in stratocumulus clouds, called pockets of open cells (POCs). They occur when regions of large, blanket clouds break up to form pockets of scattered clouds. This change in cloud cover can dramatically reduce the amount of heat reflected into space.
“A number of papers suggested that if aerosols could change the default state of these POCs from being more open to closed, the cooling effect could be huge,” says Stier. “But none of these papers has analyzed the occurrence of POCs statistically, because you would have to go through a huge amount of satellite data and, by human eye, this is far too laborious.”
So Duncan Watson-Parris, a postdoc and iMIRACLI course director, led the development of an object-detection model based a convolutional neural network, and trained the model using a set of human-annotated POCs in satellite images. Then they let the model zoom through 20 years of NASA’s high-resolution satellite imagery. The model ran on the AWS cloud, using scalable, high performance EC2 P2 instances. It was made easier thanks to the high-speed “Janet” connection between AWS cloud and JASMIN, the UK's data analysis facility for environmental science in Harwell, where the satellite data was stored.
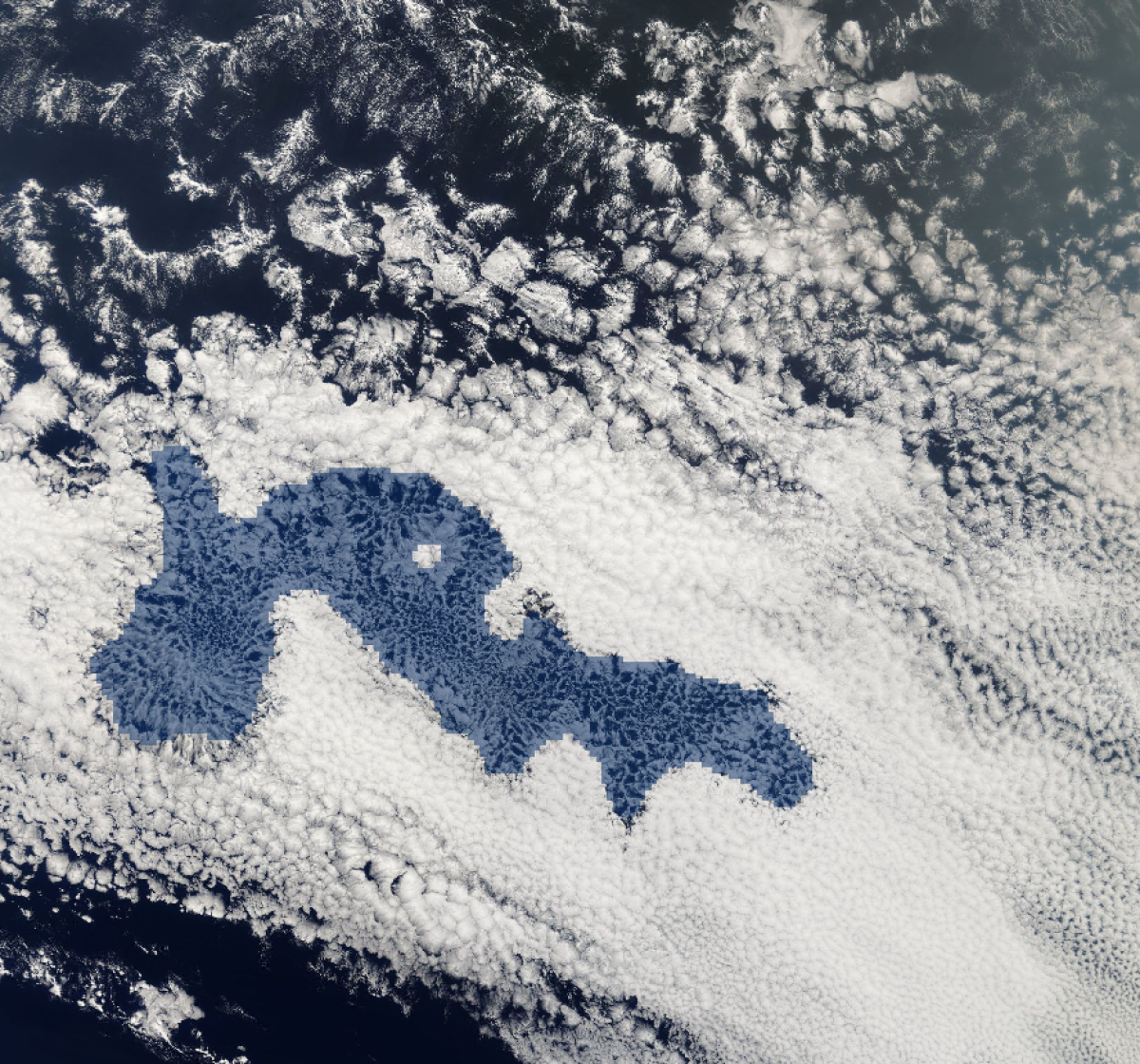
The model identified 8,500 instances of POCs in the data set, creating the world’s first comprehensive database of POCs. Analysing these data, the team concluded that the global radiative effect of POCs was actually very small.
“It turned out that POCs are pretty rare and probably wouldn’t have large climate impacts,” says Watson-Parris. “However, the transition from closed to open cellular convection more generally is a crucial challenge in understanding how clouds will respond to the warming climate and, in turn, what effect that will have on warming. So right now on AWS we are combining our POC model with more recent work on detecting night-time clouds to track the transition from closed to open stratocumulus clouds.”
Simple sophistication
Another challenge that the iMIRACLI team is using AWS to address is the enormous amount of computing resources it can take to run climate and other Earth-system models. Such models may have hundreds of tuneable parameters, require immense compute power, and generate terabytes of output. In short, they are unwieldy. To this end, the team has developed what they call a scalable Earth System Emulator — a tool for emulating and validating a variety of more complex models and outputs.
“What policymakers often want is a single number or just one line plotted, to answer questions such as ‘If the amount of atmospheric sulphur dioxide doubled, what would the impact be on the temperature in 2050?’” The problem is, explains Stier, complex climate models are not, by definition, simple creatures.
“So the ability to emulate the behavior of these complex models with something much more simple is very useful,” He says. Running on AWS’s deep learning machine instances, the emulator has already been successfully put to use in the UK Met Office’s climate model, improving the calibration of its physics and reducing uncertainties in the effect of atmospheric aerosols on historical and future warming.
The Earth System Emulator is an open-source tool based in the cloud. Cloud services are a key aspect of climate science’s future, says Stier.
Our personal #RaceToZero @OxfordNetZero is accelerating! The trench to the end of the garden is done (my last two weekends) and our heat pump is being installed this week! Gas hob will go next week and the gas line capped, leaving only renewable electricity... @ClimateOxford pic.twitter.com/ra3HC5VvIr
— Philip Stier (@PhilipStier) July 8, 2021
“The real issue in our field is the colossal data sets, which are now so big that we can't move the data around so much anymore. Ideally, we want to compute in the cloud, and have the analysis and ML tools right where the data are. AWS, for example, is already archiving satellite data, so without really moving the data we can work at a computing scale beyond the installed capacity of most universities. It’s a big change.”
Watson-Parris agrees, adding that cloud-based services also open climate science to a wider variety of researchers.
“Platforms like Amazon SageMaker lower the barrier to entry for scientists who don’t necessarily have the computational or machine learning background to set up their own deep learning instances,” he says. “That helps to increase access to climate data, which can otherwise be somewhat siloed in the large, well-funded centers in the Global North.”
It will certainly take a worldwide effort to clear the uncertainty around the present and future effects of clouds on the Earth’s climate, and Stier is gratified to be in the vanguard of this work.
“It's a very big challenge, but a fascinating one,” he says. “Only when we fully understand all the factors controlling clouds — including their exact response to pollution, but also to environmental factors — will I be happy to retire.”