For many patients, time moves at a glacial pace during a magnetic resonance imaging (MRI) scan. Those who have had one know the challenge of holding impossibly still inside a buzzing, knocking scanner for anywhere from several minutes to more than an hour.
Jonathan (Jon) Tamir is developing machine learning methods to shorten exam times and extract more data from this essential — but often uncomfortable — imaging process.
MRI machines use the body's response to strong magnetic fields and radiofrequency waves to produce pictures of our insides, helping to detect disease and monitor treatments. Just like any image, an MRI scan begins with raw data. Tamir, who is an assistant professor of electrical and computer engineering at the University of Texas at Austin, wants to improve how that data is acquired and derive better images faster. In 2020, he received an Amazon Machine Learning Research Award from Amazon Web Services (AWS) to support the work.
A lack of 'ground-truth' MRI data
Contrary to how the experience might feel to patients inside them, MRI machines move incredibly fast, collecting thousands of measurements at intervals spanning tens or hundreds of milliseconds. The measurements depend on the order and frequency of how magnetic forces and radiofrequency currents are applied to the area being surveyed. Clinicians run specific sequences tailored to the body part and purpose for the MRI.
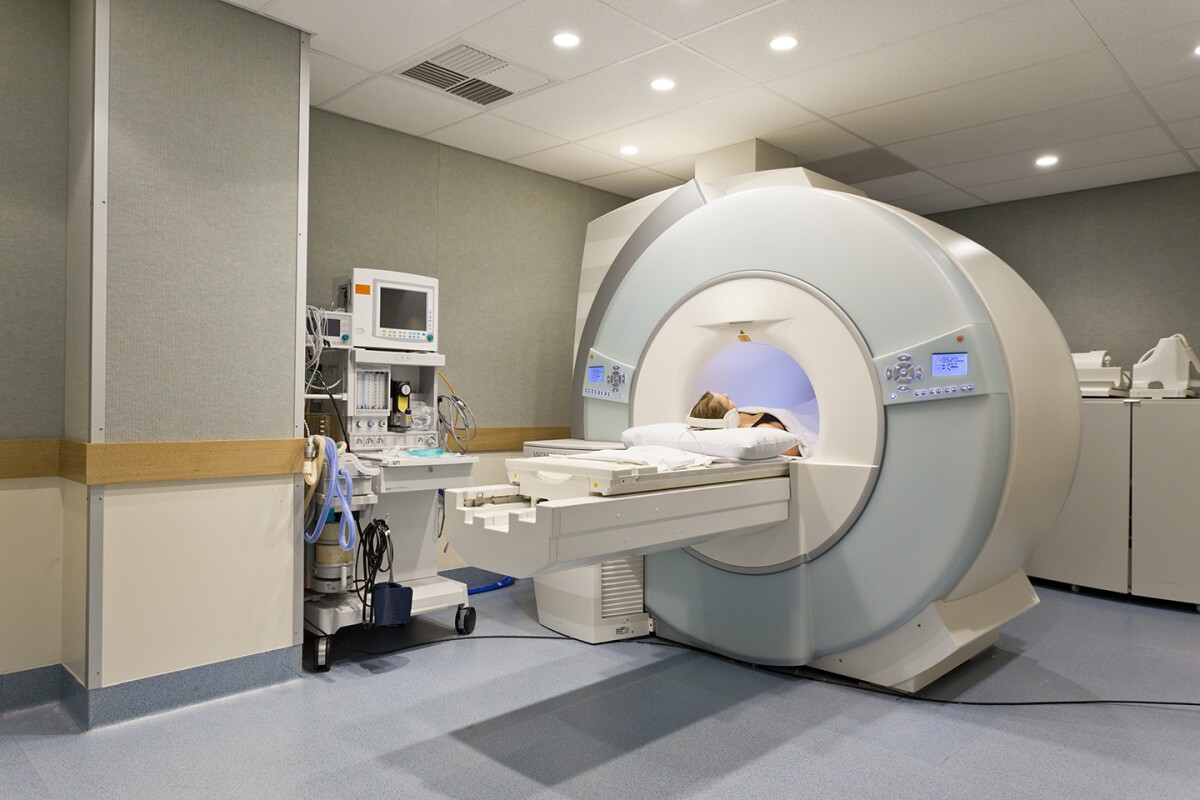
To get the highest possible image quality, an MRI technologist must collect all possible measurements, building from low to high frequency. Each layer of added data results in clearer and more detailed images, but collecting that much data takes far too long. Given the need for expedience, only a subset of the data can be acquired. Which data? "That depends on how we're planning to reconstruct the image," Tamir explained.
At his Computational Sensing and Imaging Lab, Tamir is working with colleagues to optimize both the methods for capturing scans and the image reconstruction algorithms that process the raw information. A key problem: lack of available "ground-truth" data: "That's a very big issue in medical imaging compared to the rest of the machine learning world,” he says.
With millions of MRIs generated each year in the United States alone, it might seem surprising that Tamir and colleagues lack data. The final image of an MRI, however, has been post-processed down to a few megabytes. The raw measurements, on the other hand, might amount to hundreds of megabytes or gigabytes that aren't saved by the scanner.
"Different research groups spend a lot of effort building high-quality datasets of ground-truth data so that researchers can use it to train algorithms," Tamir said. "But these datasets are very, very limited."
Another issue, he added, is the fact that many MRIs aren't static images. They are movies of a biological process, such as a heart beating. An MRI scanner is not fast enough to collect fully sampled data in those cases.
Random sampling
Tamir and colleagues are working on machine learning algorithms that can learn from limited data to fill in the blanks, so to speak, on images. One tactic being explored by Tamir and others is to randomly collect about 25% of the possible data from a scan and train a neural network to reconstruct an entire image based on that under-sampled data. Another strategy is to use machine learning to optimize the sampling trajectory in the first place.
"Random sampling is a very convenient approach, but we could use machine learning to decide the best sampling trajectory and figure out which points are most important," he said.
In “Robust Compressed Sensing MRI with Deep Generative Priors”, which was presented at the Neural Information Processing Systems (NeurIPS) 2021 conference, Tamir and colleagues at UT-Austin demonstrated a deep learning technique that achieves high-quality image reconstructions based on under-sampled scans from New York University’s fastMRI dataset and the MRIData.org dataset from Stanford University and University of California (UC) Berkeley. Both are publicly available for research and education purposes.
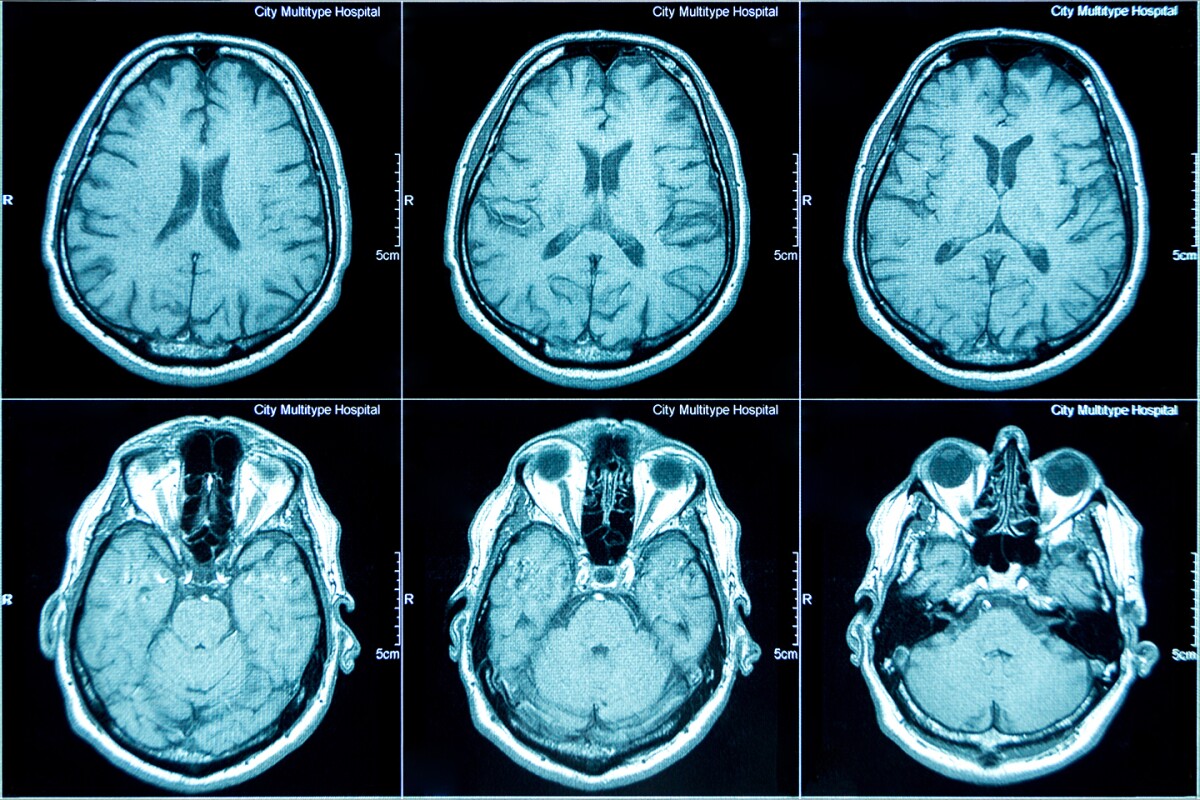
Other approaches to the problem of image reconstruction have utilized end-to-end supervised learning, which performs well when trained on specific anatomy and measurement models but tends to degrade when faced with the aberrations common in clinical practice.
Instead, Tamir and colleagues used distribution learning, in which a probabilistic model learns to approximate images without reference to measurements. In this case, the model can be used both when the measurement process changes, for example, when changing the sampling trajectory, as well as when the imaging anatomy changes, such as when switching from brain scans to knee scans that the model hasn’t seen before.
'"We're really excited to use this as a base model for tackling these bigger issues we’ve been talking about, such as optimally choosing the measurements to collect, and working with less fully available ground-truth data," Tamir said.
Tamir and his colleagues have published three additional papers related to the Amazon Research Award. One focuses on using hyberbolic geometry to represent data; another uses unrolled alternating optimization to speed MRI reconstruction. Tamir has also developed an open-source simulator for MRI that can be run on GPUs in a distributed way to find the best scan parameters for a specific reconstruction.
The road to clinical adoption
A conventional MRI assembles the image via calculations based on the fast Fourier transform, a bedrock algorithm that resolves combinations of different frequencies. "An inverse fast Fourier transform is all it takes to turn the raw data into an image," he said. "That can happen in less than a few milliseconds. It's very simple."
But in his work with machine learning, Tamir is doing those basic operations in an iterative way, performing a Fourier transform operation hundreds or thousands of times and then layering on additional types of computation.
We're not just trying to come up with cool methods that beat the state of the art in this controlled lab environment. We actually want to use it in the hospital, with the goal of improving patient outcomes.
Those calculations are performed in the Amazon Web Services cloud. The ability to do so as quickly as possible is key not only from a research perspective but also a clinical one. That's because even if the method of taking the raw measurements speeds up the MRI, the clinician still must check the quality of the image while the patient is present.
“If we have a fast scan, but now the reconstruction takes 10 minutes or an hour, then that's not going to be clinically feasible," he said. "We're extending this computation, but we need to do it in a way that maintains efficiency."
In addition to AWS cloud services, Tamir has used AWS Lambda to break the image reconstruction down pixel-by-pixel, sending small bits of data to different Lambda nodes, running the computation, and then aggregating the results.
Tamir was already familiar with AWS from his work as a graduate student at UC Berkeley, where he earned his doctorate in electrical engineering. There, he worked with Michael (Miki) Lustig, a professor of electrical engineering and computer science, on using deep learning to reduce knee scan times for patients at Stanford Children's Hospital.
As an undergrad, Tamir explored his interest in digital signal processing through unmanned aerial vehicles (UAVs), working on methods for detecting objects on the ground. After taking Lustig's Principles of MRI course at UC Berkeley, he fell in love with MRI: "It had all of the same mathematical excitement that imaging for UAVs had, but it was also something you could visually see, which was just so cool, and it had a really important societal impact."
Tamir also works with clinicians to understand MRI issues in practice. He and Léorah Freeman, a neurologist who works with multiple sclerosis (MS) patients at UT Health Austin, are trying to figure out how machine learning approaches could make brain scans faster while also detecting attributes that humans might not see.
"Tissues that look healthy to the naked eye on the brain MRI may not be healthy if we were to look at them under the microscope," Freeman said. "When we use artificial intelligence, we can look broadly into the brain and try to identify changes that may not be perceptible to the naked eye that can relate to how a patient is doing, how they're going to do in the future, and how they respond to a therapy."
Tamir and Freeman are starting by scanning the brains of healthy volunteers to establish control images to compare with those of MS patients. He hopes that the machine learning method presented at NeurIPS can be tailored to patients with MS at the Dell Medical School in Austin. It could be five to 10 years, he said, before a given method makes its way into standard MRI protocols. But that is Tamir's main goal: clinical adoption.
"We're not just trying to come up with cool methods that beat the state of the art in this controlled lab environment," he said. "We actually want to use it in the hospital, with the goal of improving patient outcomes.”