Philip Resnik was a computer science undergrad at Harvard when he accompanied a friend to her linguistics class. Through that course, he discovered a fascination with language. Given his background, he naturally approached the topic from a computational perspective.
Now a professor at the University of Maryland in the Department of Linguistics and the Institute for Advanced Computer Studies, Resnik has been doing research in computational linguistics for more than 30 years. One of his goals is to use technology to make progress on social problems. Influenced by his wife, clinical psychologist Rebecca Resnik, he became especially interested in applying computational models to identify linguistic signals related to mental health.
“Language is a crucial window into people's mental state,” Resnik said.
With the support of Amazon’s Machine Learning Research Award (MLRA), he and his colleagues are currently applying machine learning techniques to social media data in an attempt to make predictions about important aspects of mental health, including the risk of suicide.
Developing more sophisticated tools to prevent suicide is a pressing issue in the United States. Suicide was the second leading cause of death among people between the ages of 10 and 34 in 2018, according to the Centers for Disease Control and Prevention (CDC). Among all ages that year, more than 48,000 Americans died by suicide. Resnik noted the COVID-19 pandemic has further increased the urgency of this problem via an “echo pandemic.” That term has been used by some in the mental health community to characterize the long-term mental health effects of sustained isolation, anxiety, and disruption of normal life.
The value of social media data
Machine learning research projects on mental health historically have relied on various types of data, such as health records and clinical interviews. But Resnik and other researchers have found that social media provides an additional layer of information, giving a glimpse into the everyday experiences of patients when they are not being evaluated by a mental healthcare provider.
Mindful of privacy and ethical concerns, Resnik envisions a system where patients who are already seeing a mental health professional are given the option to consent for access to their social media data for this monitoring purpose.
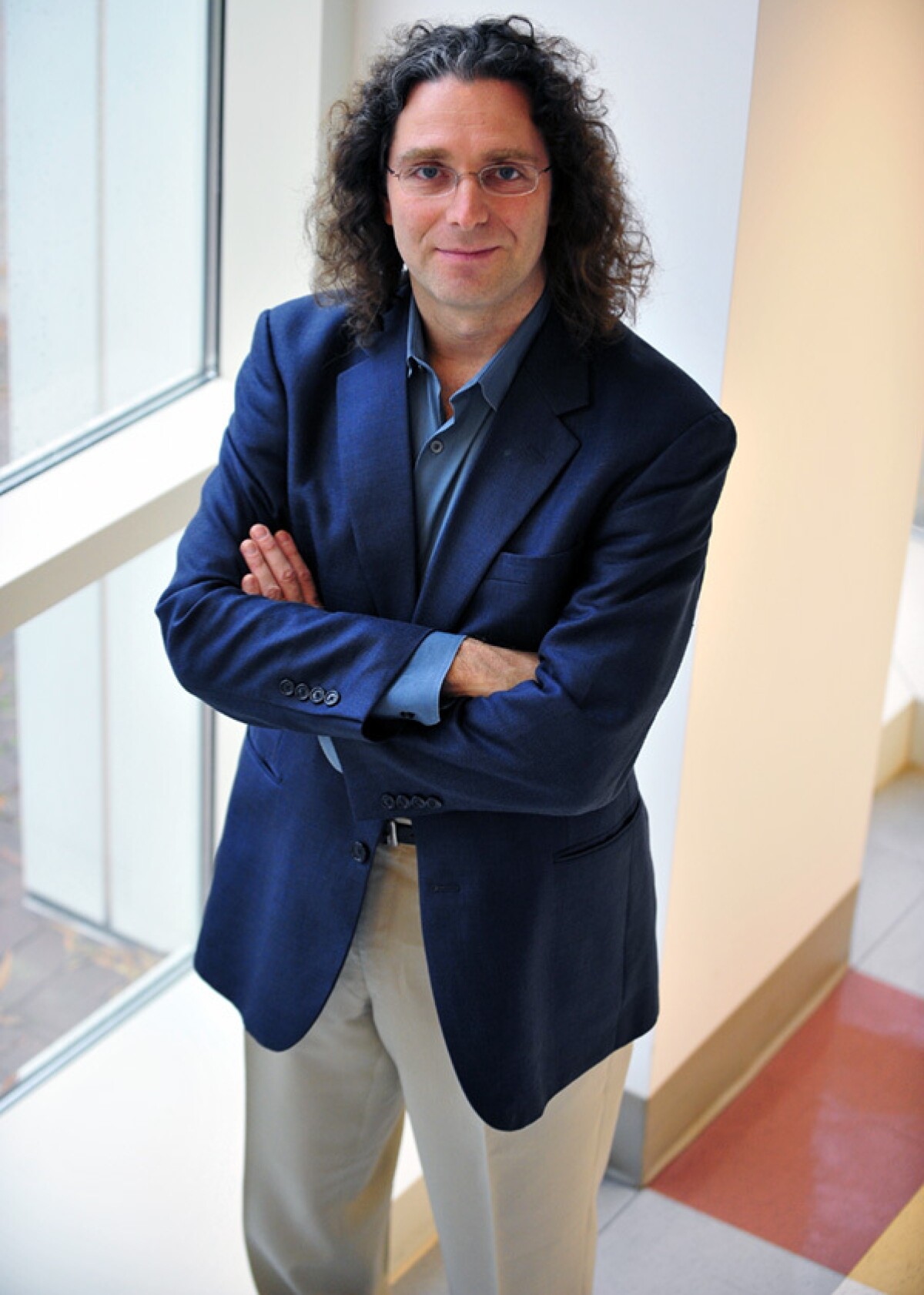
“Healthcare visits, where problems can be identified, are relatively few and far between compared to what so many people are doing every day, posting about their lived experience on social media,” Resnik said. The idea is to use social media data to discover patterns that are predictive, for example, of someone with schizophrenia having a psychotic episode, or someone with depression having a suicidal crisis.
The project is still in the technology research stage, said Resnik, but the ultimate goal is to have a practical impact by allowing mental healthcare professionals to access previously unavailable information about the people that they're helping treat.
These predictions are made possible via supervised machine learning. In this scenario, the model utilizes datasets comprised of social media posts to learn how to identify patterns or properties to make a prediction after being given a large number of correct examples.
In order to do this work, Resnik and colleagues are using social media data donated by volunteers using two sites, “OurDataHelps” and “OurDataHelps: UMD”, as well as data from Reddit. All their work receives careful ethical review and they take extra steps to anonymize the users, such as automatically masking anything that resembles a name or a location.
Prioritizing at-risk individuals
Previous work that used machine learning to make mental health predictions has generally aimed to make binary distinctions. For example: Should this person be flagged as at risk or not? However, Resnik and his team believe that simply flagging people who might require attention is not enough.
In the United States, more than 120 million people live in areas with mental healthcare provider shortages, according to the Bureau of Health Workforce. “This means that even if they know they need help with a mental health problem, they are likely to have a hard time seeing the mental healthcare provider, because there aren’t enough providers,” Resnik noted.
What happens when software identifies even more people that might need help in an already overburdened system? The answer, he said, is to find ways to help prioritize the cases that need the most attention the soonest.
You have a pipeline where, at every stage that you assess the patient, there might be an appropriate intervention. The idea is to find the right level of care across the population, as opposed to simply making a binary distinction.
This is why Resnik’s team shifted their emphasis from simple classification to prioritization. In one approach, a healthcare provider would be informed which patients are more at risk and require the most immediate attention. The system would not only rank the most at-risk individuals, but also rank, for each of them, which social media posts were most indicative of that person’s mental state. This way, when the provider got an alert, they wouldn’t have to go through possibly hundreds of social media updates to better evaluate that person’s condition. Instead, they would be shown the most concerning posts up front.
Resnik and colleagues described this in a recent paper. Although the idea hasn’t yet been put into practice by clinicians, it was developed in consultation with experts from organizations such as the American Association of Suicidology who provided valuable input and feedback into how these technologies should be designed to be both effective and ethical.
Resnik’s team is also working on another approach to patient prioritization, a system that would rely on multiple stages of patient assessment. For example, patients’ social media data could be evaluated unintrusively in the first stage. A subset of individuals then might be invited to go through to a second, interactive, stage, such as responding to questions through an automatic system where their answers and properties of their speech, for example their speaking rate and the quality of their voice, would be evaluated through machine learning techniques. Among those, the individuals at most immediate or serious risk could be directed to a third stage of evaluation that would involve a human being.
Just published: Resnik P., Foreman A., Kuchuk M., Musacchio Schafer, K., Pinkham, B., et al. Naturally occurring language as a source of evidence in suicide prevention. Suicide Life Threat Behav. 2020;00:1–9. https://t.co/Y6y4BJ8bsQ
— Philip Resnik (@psresnik) September 15, 2020
“You have a pipeline where, at every stage that you assess the patient, there might be an appropriate intervention,” Resnik said. “The idea is to find the right level of care across the population, as opposed to simply making a binary distinction.”
Both of these approaches have been supported by the MLRA. “It has been helpful not only in terms of the AWS credits to build infrastructure and the funding for graduate students, but also the engagement with people at Amazon,” said Resnik. “We’ve had active conversations with people inside AWS, who are themselves responsible for building important tools. The relationship that I have, as a researcher, with Amazon has been enormously helpful.”
Building a secure environment for sensitive data
Previous funding from the MLRA also helped sponsor the development of a secure computational environment to house mental health data. This is an important step to advance research in machine learning for mental health, as one of the main obstacles in this field is obtaining access to this very sensitive data.
The goal of this joint project between the University of Maryland and the independent research institution NORC at the University of Chicago: give qualified researchers ethical and secure access to mental health datasets. The resulting Mental Health Data Enclave, hosted on AWS, is designed to let researchers access datasets remotely from their own computers and work with them inside a secure environment, without ever being able to copy or send the data elsewhere.
The enclave will be used this spring for an exercise at the Computational Linguistics and Clinical Psychology Workshop (held in conjunction with NAACL), an event that brings together clinicians and technologists. A sensitive mental health dataset will be shared among different teams, who will work on it within the enclave to solve a problem. The solutions will then be discussed at the workshop.
Resnik said that the AWS award will make it possible for all the teams to ethically access and work on this sensitive data. “I view this as a proof of concept for what I hope will become a lasting paradigm going forward, where we use secure environments to get the community working in a shared way on sensitive data,” he added. “This is the way that real progress has been made for decades in other research areas.” Crucially, though, Resnik observes, research progress is not an end in itself: ultimately it needs to feed into practical and ethical deployment within the mental healthcare ecosystem. As he and collaborating suicide prevention experts noted in a recent article, “The key to progress is closer and more consistent engagement of the suicidology and technology communities.”