The Amazon Research Awards is a program that provides unrestricted funds and AWS Promotional Credits to academic researchers investigating research topics across a number of disciplines.
Today, we’re publicly announcing 75 award recipients who represent 52 universities in 17 countries. Each award is intended to support the work of one to two graduate students or postdoctoral students for one year, under the supervision of a faculty member.
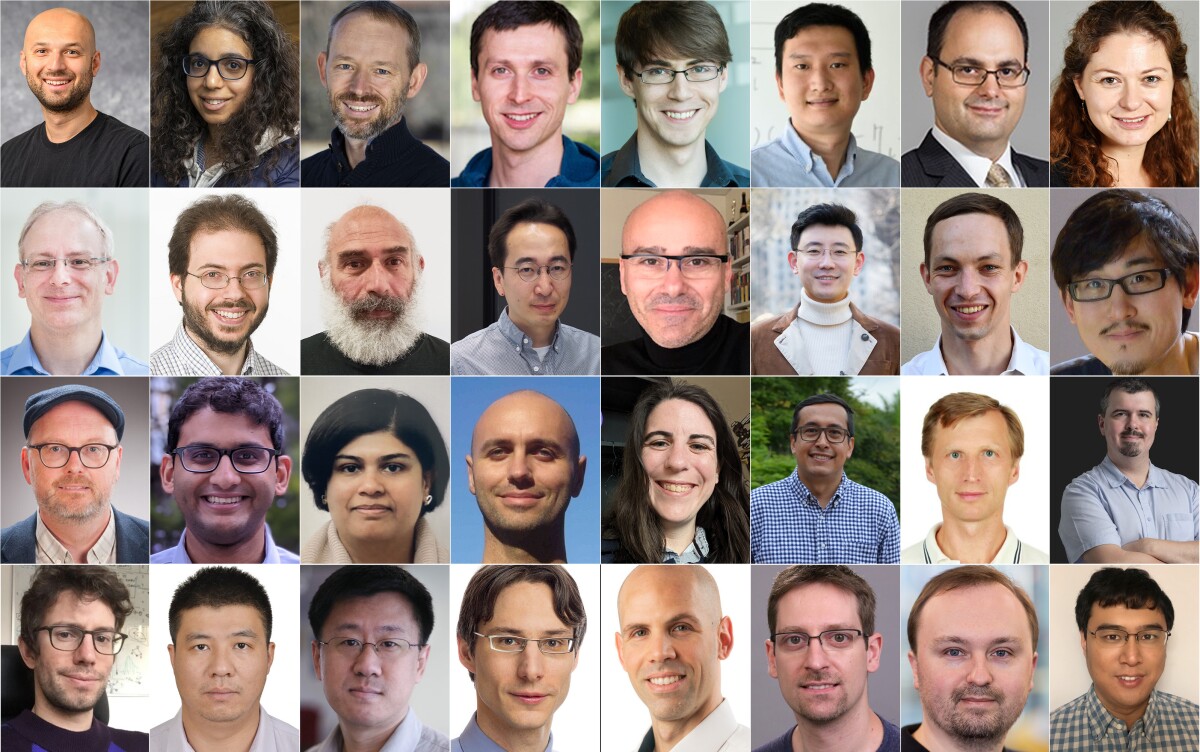
This announcement includes awards funded under seven call for proposals during the Fall 2021 cycle: AI for Information Security, Amazon Device Security and Privacy, Amazon Payments, AWS Automated Reasoning, Data for Social Sustainability, Prime Video, and Robotics. Proposals were reviewed for the quality of their scientific content, their creativity, and their potential to impact both the research community and society more generally. Theoretical advances, creative new ideas, and practical applications were all considered.
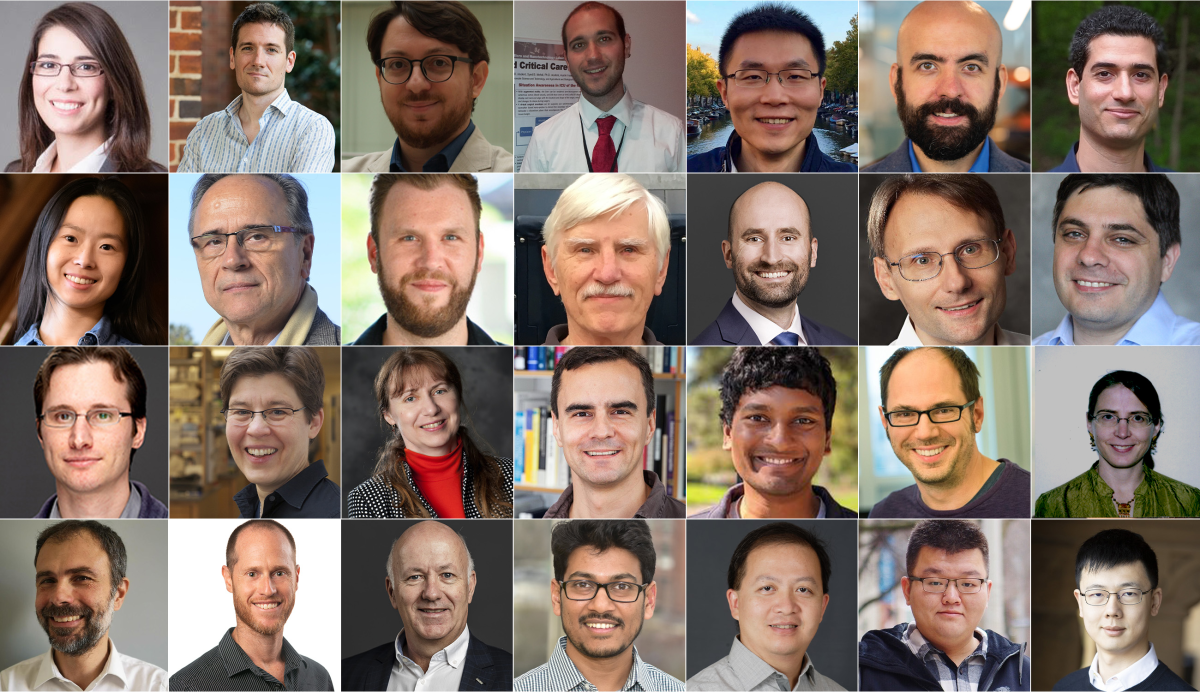
Recipients have access to more than 300 Amazon public datasets and can utilize AWS AI/ML services and tools through their AWS Promotional Credits. Recipients also are assigned an Amazon research contact who offers consultation and advice, along with opportunities to participate in Amazon events and training sessions.
Additionally, Amazon encourages the publication of research results, presentations of research at Amazon offices worldwide, and the release of related code under open-source licenses.
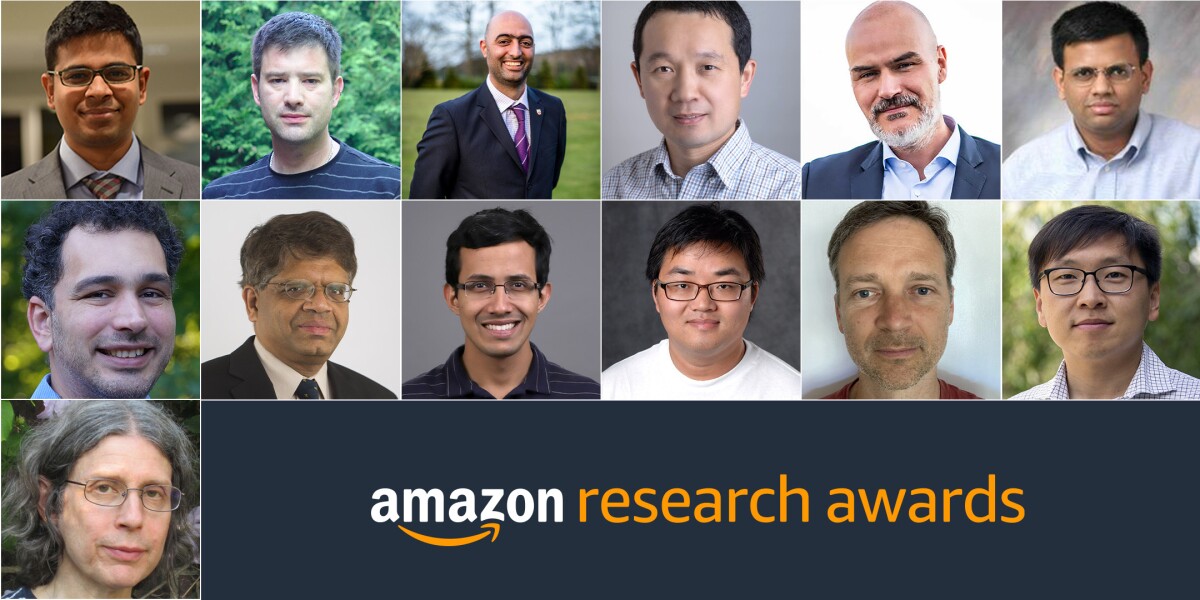
"Research in automated reasoning is deeply intertwined with a broad range of other research areas, touching machine learning, hardware and software engineering, robotics, and life sciences," said Daniel Kroening, an Automated Reasoning Group senior principal scientist. "The 2021 Amazon Research Awards reflect this breadth, and the interdisciplinary nature of research that is necessary to take computing one step closer to that magic spark that drives human reasoning."
ARA funds proposals up to four times a year in a variety of research areas. Applicants are encouraged to visit the ARA call for proposals page for more information or send an email to be notified of future open calls.
The table below lists, in alphabetical order, Fall 2021 cycle call-for-proposal recipients.
Recipient | University | Research title |
Aws Albarghouthi | University of Wisconsin-Madison | Teaching SMT Solvers Probability Theory |
Nada Amin | Harvard University | Extensible Models and Proofs |
Nora Ayanian | Brown University | Large-Scale Labeled Multi-Agent Pathfinding for Warehouses |
Clark Barrett | Stanford University | HydraScale: Solving SMT Queries in the Serverless Cloud |
Ivan Beschastnikh | University of British Columbia | Compiling Distributed System Models into Implementations |
Nicola Bezzo | University of Virginia | Towards Safe and Agile Robot Navigation in Occluding and Dynamic Environments |
William Bowman | University of British Columbia | Static reasoning for memory in compilers and intermediate languages |
Yinzhi Cao | Johns Hopkins University | Automatic Static Resource Analysis for Serverless Computing |
Luca Carlone | Massachusetts Institute of Technology | Real-time Spatial AI for Robotics |
Trevor Carlson | National University of Singapore | Accelerating SAT Solving with a Flexible FPGA-Programming Platform |
Marsha Chechik | University Of Toronto | Unsatisfiability Proofs for Monotonic Theories |
Venanzio Cichella | University Of Iowa | Concurrent allocation and planning for large-scale multi-robot systems |
Cas Cremers | CISPA Helmholtz Center for Information Security | KeyLife: Automated Formal Analysis for Key Lifecycles in Security Protocols with Policies, Delegation, and Compromise |
Elizabeth Croft | Monash University | Help me!: Humans supporting robots through Augmented Reality |
Jia Deng | Princeton University | Optimization-Inspired Neural Networks for Visual SLAM |
Derek Dreyer | MPI - SWS | RefinedRust: Automating the Verification of Rust Programs in the Presence of Unsafe Code |
Tudor Dumitras | University of Maryland, College Park | Mitigating the impact of behavior variability and label noise on ML-based malware detectors |
Nima Fazeli | University of Michigan | Object Manipulation with High-Resolution Tactile Sensors |
Earlence Fernandes | University of Wisconsin-Madison | Verifiable Distributed Computation |
Marcelo Frias | Buenos Aires Institute of Technology | Modular Bounded Verification with Expressive Contracts |
Sicun Gao | University of California, San Diego | Interior Search Methods in SMT |
Maani Ghaffari-Jadidi | University of Michigan | Robust low-cost dead reckoning and localization for home robotics using invariant state estimation |
Roberto Giacobazzi | University of Verona | Implicit program analysis |
Ronghui Gu | Columbia University | Learning Inductive Invariants for Real Distributed Protocols |
Grace Gu | University of California, Berkeley | Deep learning-enabled robust grasping for pneumatic actuators |
Leonidas Guibas | Stanford University | GeneralPurpose 3D Perception of Object Functionality |
Arie Gurfinkel | University of Waterloo | Formal Proofs for Trusted Execution Environments |
Hamed Haddadi | Imperial College London | Auditable Model Privacy using TEEs |
Felix Heide | Princeton University | Inverse Neural Rendering |
Ralph Hollis | Carnegie Mellon University | Low Cost Dynamic Mobile Robots for Research and Teaching |
Hongxin Hu | SUNY, Buffalo | Explaining Learning-based Intrusion Detection Systems for Active Intrusion Responses |
Jean-Baptiste Jeannin | University of Michigan-Ann Arbor | Automatic Verification of Distributed Systems Implementations |
Robert Katzschmann | ETH Zurich | Design and Control Optimization of Soft Gripper Mechanisms for Manipulation |
Anirudh Sivaraman Kaushalram | New York University | Observing and controlling microservice deployments |
Steve Ko | Simon Fraser University | Practical Symbolic Execution for Rust |
Sven Koenig | University of Southern California | Hybrid Search- and Traffic-Based MAPF Systems for Fulfillment Centers |
George Konidaris | Brown University | Learning Composable Manipulation Skills |
Emmanuel Letouzé | Pompeu Fabra University | Leveraging Digital Data for Monitoring Human Rights and Social Dynamics Along and Around Value Chains |
Sergey Levine | University of California, Berkeley | Robotic Learning with Reusable Data |
Jennifer Lewis | Harvard University | Computational Co-Design of Dexterous Rigid-Soft Grippers With Intrinsic Tactile-Sensing-Based Control |
Maja Matarić | University of Southern California | Learning User Preferences for In-Home Robots Through In Situ Augmented Reality |
James Noble | Victoria University Of Wellington | “Programming Made Hard” Made Easier: Improving Dafny’s Human Factors |
Rohan Padhye | Carnegie Mellon University | Coverage-Guided Property-Based Testing of Concurrent Programs |
Jan Peters | TU Darmstadt | Learning Robot Manipulation from Tactile Feedback |
Lerrel Pinto | New York University | Visual Imitation in the Wild through Decoupled Representation Learning |
Robert Platt | Northeastern University | On-robot manipulation learning via equivariant models |
Nancy Pollard | Carnegie Mellon | Contact Areas for Manipulation Capture, Retargeting, and Hand Design |
Pavithra Prabhakar | Kansas State University | Conformance Checking of Evolving ML Software Systems |
Francesco Ranzato | University of Verona | Implicit program analysis |
Sanjay Rao | Purdue University | Answering counterfactuals from offline data for video streaming |
Bruno Ribeiro | Purdue University | Answering counterfactuals from offline data for video streaming |
Talia Ringer | University of Illinois Urbana-Champaign | Neurosymbolic Proof Synthesis & Repair |
Alessandro Rizzo | Politecnico di Torino | Physics-Informed Machine Learning for Trustworthy Control of Autonomous Robots |
Camilo Rocha | Pontificia Universidad Javeriana Cali | Probabilistic and Symbolic Tools for P Program Verification |
Andrei Sabelfeld | Chalmers University of Technology | DeepCrawl: Automated Reasoning for Deep Web Crawling |
Oren Salzman | Technion - Israel Institute of Technology | Increasing throughput in automated warehouses via environment manipulation |
Ilya Sergey | National University of Singapore | Scaling Automated Verification of Distributed Protocols with Specification Transformation and Synthesis |
Michele Sevegnani | University of Glasgow | From Whiteboards to Models: Diagrammatic Formal Modelling for Everyone |
Roland Siegwart | ETH Zurich | Autonomous Navigation of Aerial Robotic Manipulators in Unstructured Indoor and Outdoor Environments |
Ramesh Sitaraman | University of Massachusetts Amherst | Design and Evaluation of ABR Algorithms for High-Performance Video Delivery |
Fu Song | ShanghaiTech University | Efficient and Precise Verification for Constant-Time and Time-Balancing of Cryptosystems |
Zhendong Su | ETH Zurich | Practical Techniques for Reliable, Robust and Performant SMT Solvers |
Jiliang Tang | Michigan State University | Taming Graph Anomaly Detection via Graph Neural Networks |
Pratap Tokekar | University of Maryland, College Park | Multi-Robot Coordination through the Lens of Risk |
Daniel Varro | McGill University | Graph Solver as a Service |
Yakir Vizel | Technion - Israel Institute of Technology | Quantified Invariants |
David Wagner | University of California, Berkeley | Machine Learning for Malware Detection: Robustness against Concept Drift |
James Wang | Pennsylvania State University | Affective and Social Interaction between Human and Intelligent Machine in Daily Activities |
Shenlong Wang | University of Illinois Urbana-Champaign | Safely Test Autonomous Vehicles with Augmented Reality |
Thomas Wies | New York University | A Modular Library of Verified Concurrent Search Structure Algorithms |
Anton Wijs | Eindhoven University of Technology | Many-Core Acceleration of State Space Construction and Analysis |
Xinyu Xing | Northwestern University | Battling Noisy-label Classification |
Meng Xu | University Of Waterloo | Finding Specification Blind Spots with Fuzz Testing |
Yuke Zhu | University of Texas at Austin | Interactive Learning Framework for Building Structured Object Models from Play |
Andrew Zisserman | University of Oxford | Audio-Visual Synchronisation for General Videos |