Amazon's contributions to this year's European Conference on Computer Vision (ECCV) reflect the diversity of the company's research interests. Below is a quick guide to the topics and methods of a dozen ECCV papers whose authors include Amazon scientists.
Fine-grained fashion representation learning by online deep clustering
Yang (Andrew) Jiao, Ning Xie, Yan Gao, Chien-Chih Wang, Yi Sun
Fashions are characterized by both global attributes, such as “skirt length”, and local attributes, such as “neckline style”. Accurate representations of such attributes are essential to tasks like fashion retrieval and fashion recommendation, but learning representations of each attribute independently ignores shared visual statistics among the attributes. Instead, the researchers treat representation learning as a multitask learning problem, enforcing cluster-level constraints on global structure. The learned representations improve fashion retrieval by a large margin.
GLASS: Global to local attention for scene-text spotting
Roi Ronen, Shahar Tsiper, Oron Anschel, Inbal Lavi, Amir Markovitz, R. Manmatha
Modern text-spotting models combine text detection and recognition into a single end-to-end framework, in which both tasks often rely on a shared global feature map. Such models, however, struggle to recognize text across scale variations (smaller or larger text) and arbitrary word rotation angles. The researchers propose a novel attention mechanism for text spotting, called GLASS, that fuses together global and local features. The global features are extracted from the shared backbone, while the local features are computed individually on resized, high-resolution word crops with upright orientation. GLASS achieves state-of-the-art results on multiple public benchmarks, and the researchers show that it can be integrated with other text-spotting solutions, improving their performance.
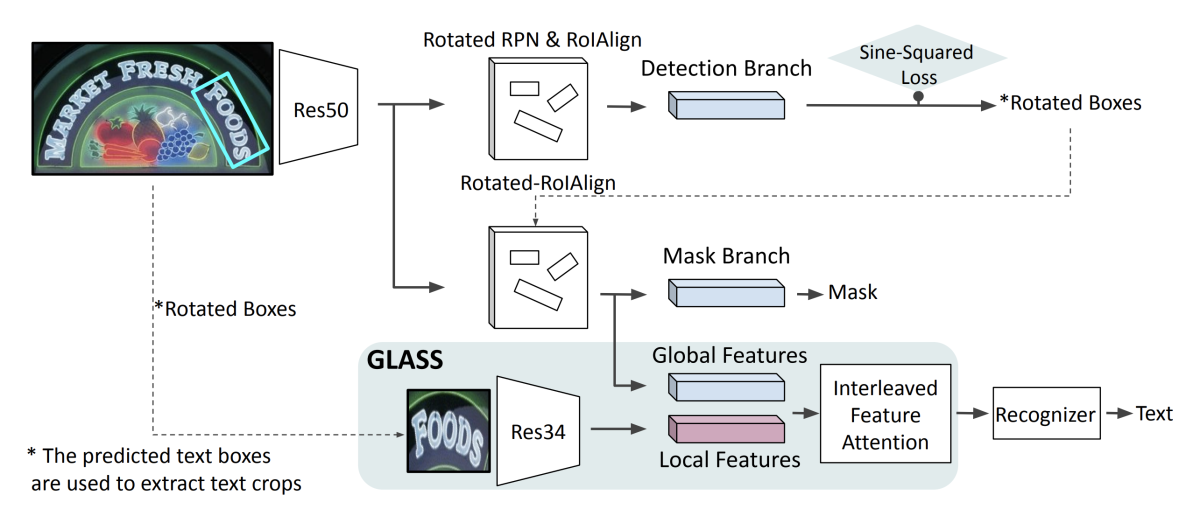
Large scale real-world multi-person tracking
Bing Shuai, Alessandro Bergamo, Uta Buechler, Andrew Berneshawi, Alyssa Boden, Joseph Tighe
This paper presents a new multi-person tracking dataset — PersonPath22 — which is more than an order of magnitude larger than existing high-quality multi-object tracking datasets. The PersonPath22 dataset is specifically sourced to provide a wide variety of conditions, and its annotations include rich metadata that allows the performance of a tracker to be evaluated along these different dimensions. Its large-scale real-world training and test data enable the community to better understand the performance of multi-person tracking systems in a range of scenarios and conditions.
MaCLR: Motion-aware contrastive Learning of representations for videos
Fanyi Xiao, Joseph Tighe, Davide Modolo
Attempts to use self-supervised learning for video have had some success, but existing approaches don’t make explicit use of motion information derived from the temporal sequence, which is important for supervised action recognition tasks. The researchers propose a self-supervised video representation-learning method that explicitly models motion cues during training. The method, MaCLR, consists of two pathways, visual and motion, connected by a novel cross-modal contrastive objective that enables the motion pathway to guide the visual pathway toward relevant motion cues.
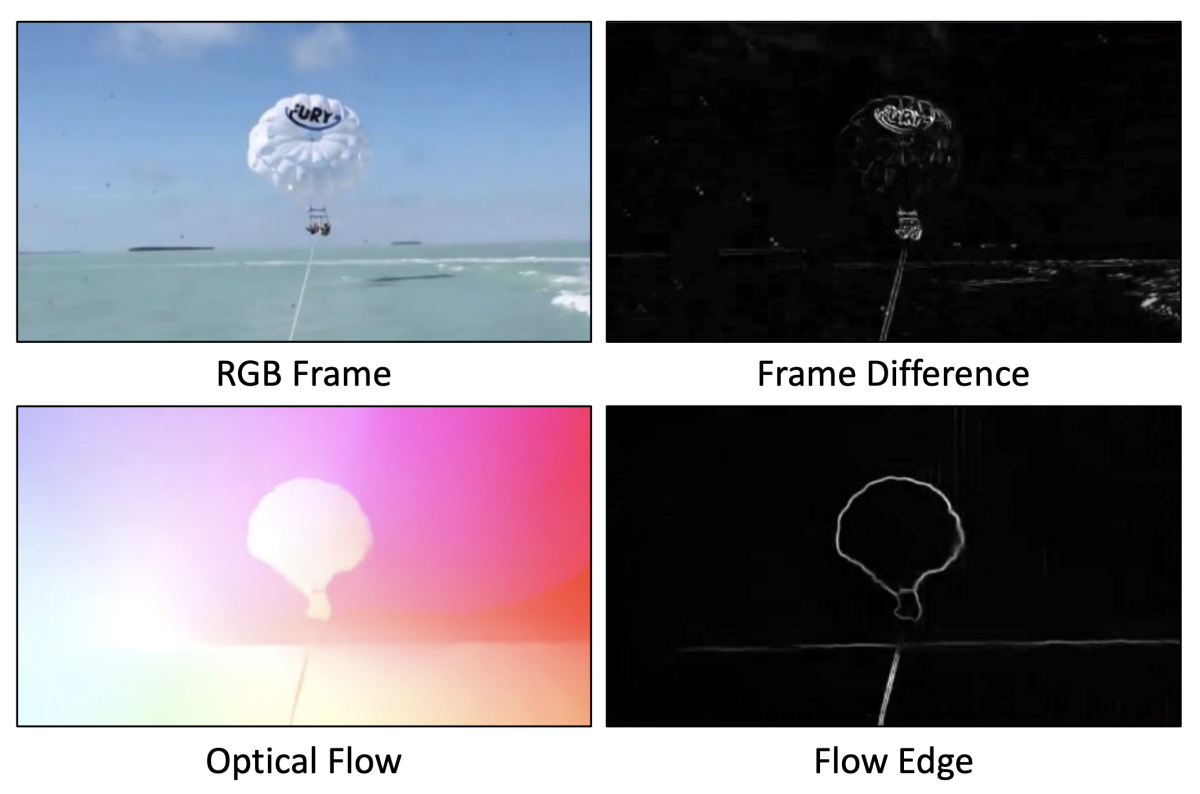
PSS: Progressive sample selection for open-world visual representation learning
Tianyue Cao, Yongxin Wang, Yifan Xing, Tianjun Xiao, Tong He, Zheng Zhang, Hao Zhou, Joseph Tighe
In computer vision, open-world representation learning is the challenge of learning representations for categories of images not seen during training. Existing approaches make unrealistic assumptions, such as foreknowledge of the number of categories the unseen images fall into, or the ability to determine in advance which unlabeled training examples fall into unseen categories. The researchers’ novel progressive approach avoids such assumptions, selecting at each iteration unlabeled samples that are highly homogenous but belong to classes that are distant from the current set of known classes. High-quality pseudo-labels generated via clustering over these selected samples then improve the feature generalization iteratively.
Rayleigh EigenDirections (REDs): Nonlinear GAN latent space traversals for multidimensional features
Guha Balakrishnan, Raghudeep Gadde, Aleix Martinez, Pietro Perona
Generative adversarial networks (GANs) can map points in a latent space to images, producing extremely realistic synthetic data. Past attempts to control GANs’ outputs have looked for linear trajectories through the space that correspond, approximately, to continuous variation of a particular image feature. The researchers propose a new method for finding nonlinear trajectories through the space, providing unprecedented control over GANs’ outputs, including the ability to hold specified image features fixed while varying others.
Rethinking few-shot object detection on a multi-domain benchmark
Kibok Lee, Hao Yang, Satyaki Chakraborty, Zhaowei Cai, Gurumurthy Swaminathan, Avinash Ravichandran, Onkar Dabeer
Most existing work on few-shot object detection (FSOD) focuses on settings where both the pretraining and few-shot learning datasets are from similar domains. The researchers propose a Multi-dOmain Few-Shot Object Detection (MoFSOD) benchmark consisting of 10 datasets from a wide range of domains to evaluate FSOD algorithms across a greater variety of applications. They comprehensively analyze the effects of freezing layers, different architectures, and different pretraining datasets on FSOD performance, drawing several surprising conclusions. One of these is that, contrary to prior belief, on a multidomain benchmark, fine-tuning (FT) is a strong baseline for FSOD.
SPot-the-Difference: Self-supervised pre-training for anomaly detection and segmentation
Yang Zou, Jongheon Jeong, Latha Pemula, Dongqing Zhang, Onkar Dabeer
Visual anomaly detection is commonly used in industrial quality inspection. This paper presents a new dataset and a new self-supervised learning method for ImageNet pretraining to improve anomaly detection and segmentation in 1-class and 2-class 5/10/high-shot training setups. The Visual Anomaly (VisA) Dataset consists of 10,821 high-resolution color images (9,621 normal and 1,200 anomalous samples) covering 12 objects in three domains, making it one of the largest industrial anomaly detection datasets to date. The paper also proposes a new self-supervised framework — SPot-the-Difference (SPD) — that can regularize contrastive self-supervised and also supervised pretraining to better handle anomaly detection tasks.
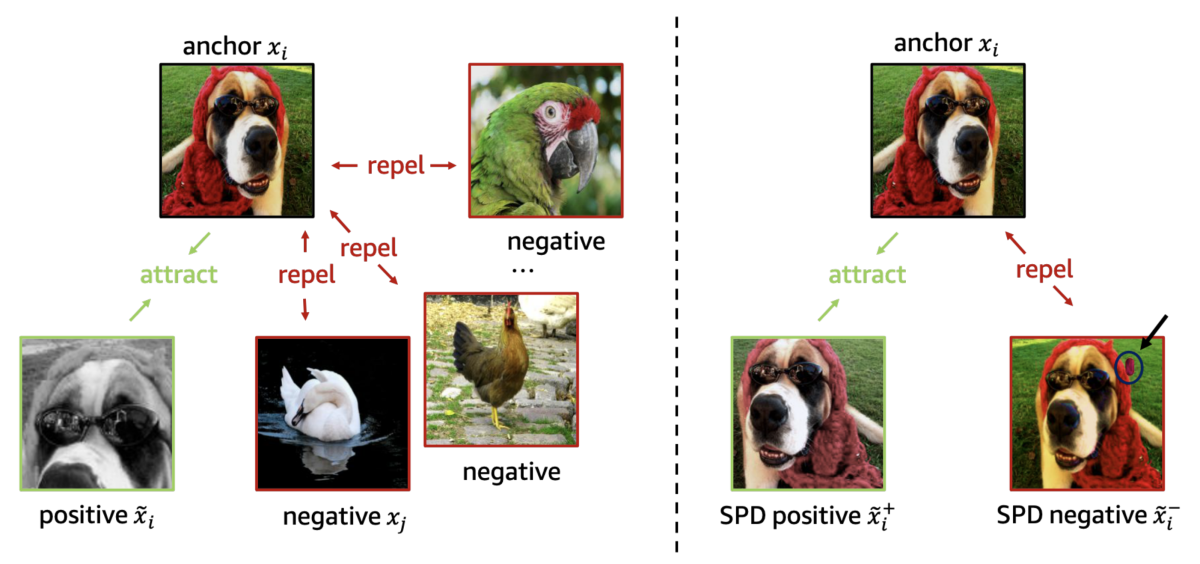
TD-Road: Top-down road network extraction with holistic graph construction
Yang He, Ravi Garg, Amber Roy Chowdhury
Road network extraction from satellite imagery is essential for constructing rich maps and enabling numerous applications in route planning and navigation. Previous graph-based methods used a bottom-up approach, estimating local information and extending a graph iteratively. This paper, by contrast, proposes a top-down approach that decomposes the problem into two subtasks: key point prediction and connectedness prediction. Unlike previous approaches, the proposed method applies graph structures (i.e., locations of nodes and connections between them) as training supervisions for deep neural networks and directly generates road graph outputs through inference.
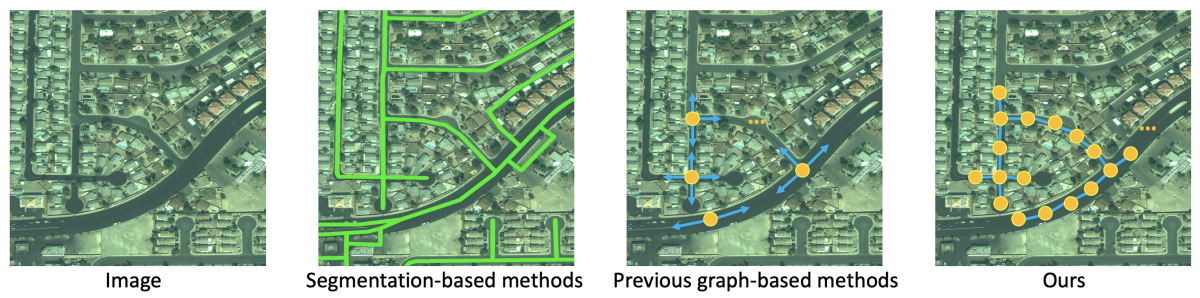
Towards regression-free neural networks for diverse compute platforms
Rahul Duggal, Hao Zhou, Shuo Yang, Jun Fang, Yuanjun Xiong, Wei Xia
Commercial machine learning models are constantly being updated, and while an updated model may improve performance on average, it can still regress — i.e., suffer “negative flips” — on particular inputs it used to handle correctly. This paper introduces regression-constrained neural-architecture search (REG-NAS), which consists of two components: (1) a novel architecture constraint that enables a larger model to contain all the weights of a smaller one, thus maximizing weight sharing, and (2) a novel search reward that incorporates both top-1 accuracy and negative flips in the architecture search metric. Relative to the existing state-of-the-art approach, REG-NAS enables 33 – 48% reduction of negative flips.
Unsupervised and semi-supervised bias benchmarking in face recognition
Alexandra Chouldechova, Siqi Deng, Yongxin Wang, Wei Xia, Pietro Perona
This paper introduces semi-supervised performance evaluation for face recognition (SPE-FR), a statistical method for evaluating the performance and algorithmic bias of face verification systems when identity labels are unavailable or incomplete. The method is based on parametric Bayesian modeling of face embedding similarity scores, and it produces point estimates, performance curves, and confidence bands that reflect uncertainty in the estimation procedure. Experiments show that SPE-FR can accurately assess performance on data with no identity labels and confidently reveal demographic biases in system performance.
X-DETR: A versatile architecture for instance-wise vision-language tasks
Zhaowei Cai, Gukyeong Kwon, Avinash Ravichandran, Erhan Bas, Zhuowen Tu, Rahul Bhotika, Stefano Soatto
This paper addresses the challenge of instance-wise vision-language tasks, which require free-form language to align with objects inside an image, rather than the image itself. The paper presents the X-DETR model, whose architecture has three major components: an object detector, a language encoder, and a vision-language alignment module. The vision and language streams are independent until the end, and they are aligned using an efficient dot-product operation. This simple architecture shows good accuracy and fast speeds for multiple instance-wise vision-language tasks, such as open-vocabulary object detection.
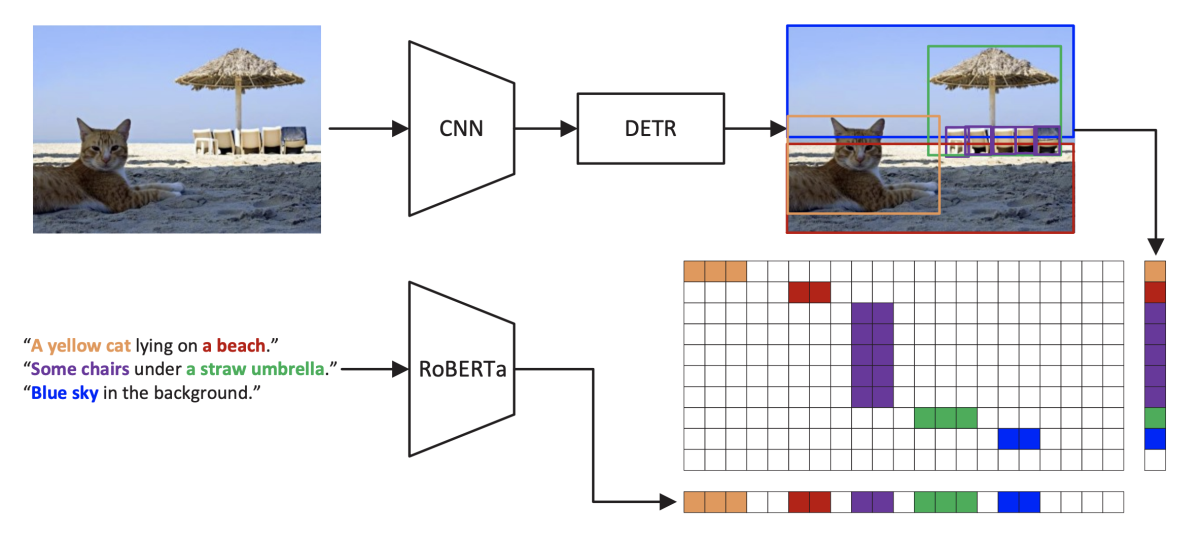