Voice agents like Alexa often have a variety of different speech synthesizers, which differ in attributes such as expressivity, personality, language, and speaking style. The machine learning models underlying these different applications can have completely different architectures, and integrating those architectures in a single voice service can be a time-consuming and challenging process.
To make that process easier and faster, Amazon’s Text-to-Speech group has developed a universal model integration framework that allows us to customize production voice models in a quick and scalable way.
Model variety
State-of-the-art voice models typically use two large neural networks to synthesize speech from text inputs.
The first network, called an acoustic model, takes text as input and generates a mel-spectrogram, an image that represents acoustic parameters such as pitch and energy of speech over time. The second network, called a vocoder, takes the mel-spectrogram as an input and produces an audio waveform of speech as the final output.
While we have released a universal architecture for the vocoder model that supports a wide variety of speaking styles, we still use different acoustic-model architectures to generate this diversity of speaking styles.
The most common architecture for the acoustic model relies on an attention mechanism, which learns which elements of the input text are most relevant to the current time slice — or “frame” — of the output spectrogram. With this mechanism, the network implicitly models the speech duration of different chunks of the text.
The same model also uses the technique of “teacher-forcing”, where the previously generated frame of speech is used as an input to produce the next one. While such an architecture can generate expressive and natural-sounding speech, it is prone to intelligibility errors such as mumbling or dropping or repeating words, and errors easily compound from one frame to the next.
More-modern architectures address these issues by explicitly modeling the durations of text chunks and generating speech frames in parallel, which is more efficient and stable than relying on previously generated frames as input. To align the text and speech sequences, the model simply “upsamples”, or repeats its encoding of a chunk of text (its representation vector), for as many speech frames as are dictated by the external duration model.
The continuous evolution of complex TTS models employed in different contexts — such as Alexa Q&A, storytelling for children, and smart-home automation — creates the need for a scalable framework that can handle them all.
The challenge of integration
To integrate acoustic models into production, we need a component that takes an input text utterance and returns a mel-spectrogram. The first difficulty is that speech is usually generated in sequential chunks, rather than being synthesized all at once. To minimize latency, our framework should return data as quickly as possible. A naive solution that wraps the whole model in code and processes everything with a single function call will be unacceptably slow.
Another challenge is adjusting the model to work with various hardware accelerators. As an example, to benefit from the high-performance AWS Inferentia runtime, we need to ensure that all tensors have fixed sizes (set once, during the model compilation phase). This means that we need to
- add logic that splits longer utterances into smaller chunks that fit specific input sizes (depending on the model);
- add logic that ensures proper padding; and
- decide which functionality should be handled directly by the model and which by the integration layer.
When we want to run the same model on general-purpose GPUs, we probably don’t need these changes, and it would be useful if the framework could switch back and forth between contexts in an easy way. We therefore decouple the TTS model into a set of more specialized integration components, capable of doing all the required logic.
Integration components
The integration layer encapsulates the model in a set of components capable of transforming an input utterance into a mel-spectrogram. As the model usually operates in two stages — preprocessing data and generating data on demand — it is convenient to use two types of components:
- a SequenceBlock, which takes an input tensor and returns a transformed tensor (the input can be the result of applying another SequenceBlock), and
- a StreamableBlock, which generates data (e.g., frames) on demand. As an input it takes the results of another StreamableBlock (blocks can form a pipeline) and/or data generated by a SequenceBlock.
These simple abstractions offer great flexibility in creating variants of acoustic models. Here’s an example:
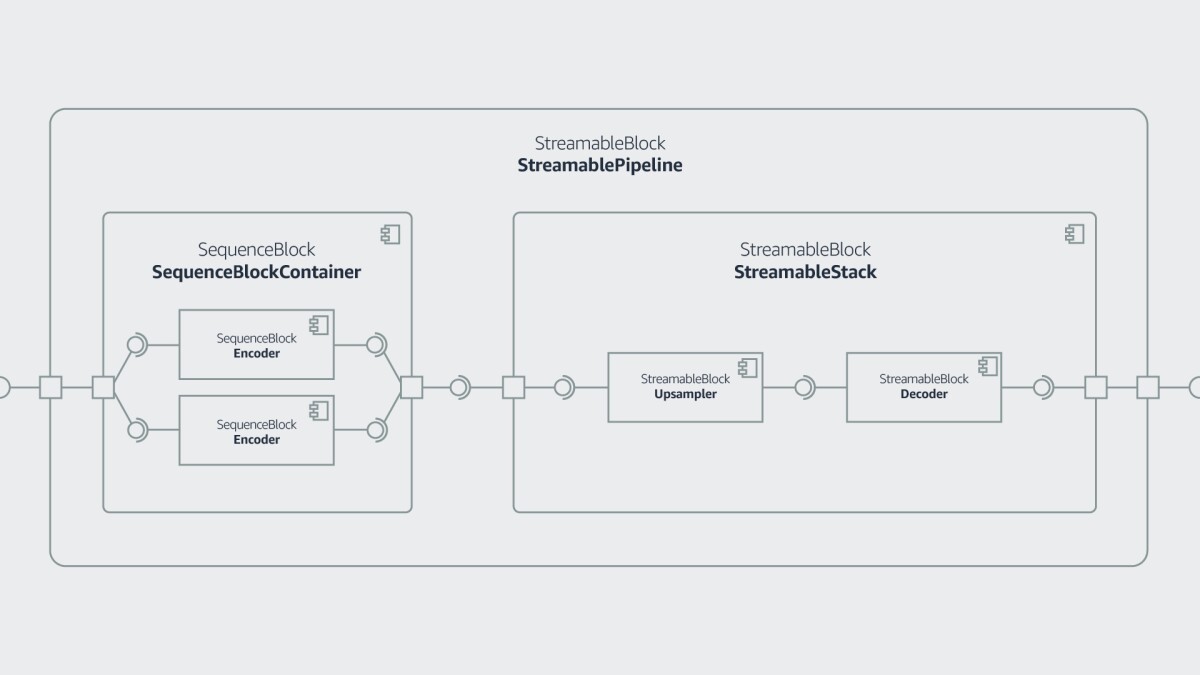
The acoustic model consists of
- two encoders (SequenceBlocks), which convert the input text embedding into one-dimensional representation tensors, one for encoded text and one for predicted durations;
- an upsampler (a StreamableBlock, which takes the encoders’ results as an input), which creates intermediary, speech-length sequences, according to the data returned by the encoders; and
- a decoder (a StreamableBlock), which generates mel-spectrogram frames.
The whole model is encapsulated in a specialized StreamableBlock called StreamablePipeline, which contains exactly one SequenceBlock and one StreamableBlock:
- the SequenceBlockContainer is a specialized SequenceBlock that consists of a set of nested SequenceBlocks capable of running neural-network encoders;
- the StreamableStack is specialized StreamableBlock that decodes outputs from the upsampler and creates mel-spectrogram frames.
The integration framework ensures that all components are run in the correct order, and depending on the specific versions of components, it allows for the use of various hardware accelerators.
The integration layer
The acoustic model is provided as a plugin, which we call an “addon”. An addon consists of exported neural networks, each represented as a named set of symbols and parameters (encoder, decoder, etc.), along with configuration data. One of the configuration attributes, called “stack”, specifies how integration components should be connected together to build a working integration layer. Here’s the code for the stack attribute that describes the architecture above:
'stack'=[ {'type' : 'StreamablePipeline, 'sequence_block' : {'type' : 'Encoders'}, 'streamable_block' : {'type': 'StreamableStack', 'stack' : [ {'type' : 'Upsampler'}, {'type' : 'Decoder'} ]} } ]
This definition will create an integration layer consisting of a StreamablePipeline with
- All encoders specified in the addon (the framework will automatically create all required components);
- An upsampler, which generates intermediate data for the decoder; and
- the decoder specified in the addon, which generates the final frames.
The JSON format allows us to make easy changes. For example, we can create a specialized component that runs all sequence blocks in parallel on a specific hardware accelerator and name it CustomizedEncoders. In this case, the only change in the configuration specification is to replace the name “Encoders” with “CustomizedEncoders”.
Running experiments using components with additional diagnostic or digital-signal-processing effects is also trivial. A new component’s only requirement is to extend one of two generic abstractions; other than that, there are no other restrictions. Even replacing one StreamableBlock with the whole nested sequence-to-sequence stack is perfectly fine, according to the framework design.
This framework is already used in production. It is a vital pillar of our recent, successful integration of state-of-the-art TTS architectures (without attention) and legacy models.
Acknowledgments: Daniel Korzekwa