The International Conference on Robotics and Automation (ICRA), the major conference in the field of robotics, takes place this week, and Amazon is one of its silver sponsors. To mark the occasion, Amazon Science sat down with three of Amazon’s leading roboticists to discuss the challenges of building robotic systems that interact with human beings in real-world settings.
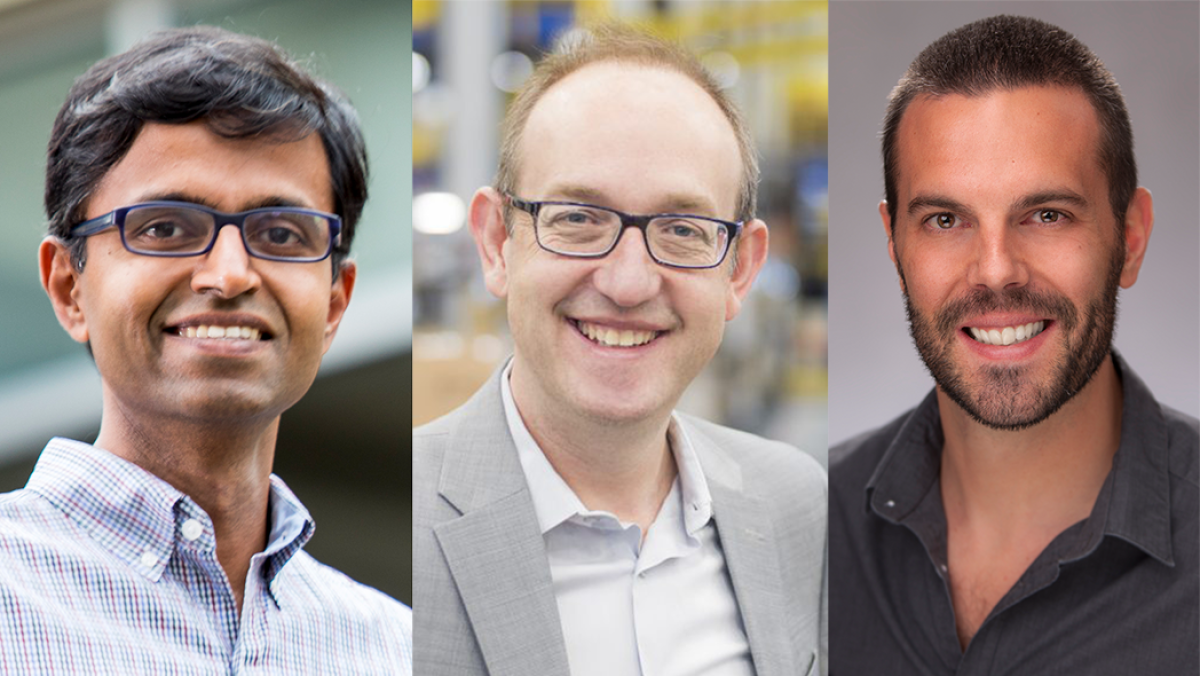
As the director of Amazon Robotics AI, Siddhartha (Sidd) Srinivasa is responsible for the algorithms that govern the autonomous robots that assist employees in Amazon fulfillment centers, including robots that can pick up and package products and the autonomous carts that carry products from the shelves to the packaging stations.
More about robotics at Amazon
Learn more about robotics at Amazon — including job opportunities — and about Amazon's participation at ICRA.
Tye Brady, the chief technologist for global Amazon Robotics, helps shape Amazon’s robotics strategy and oversees university outreach for robotics.
Philipp Michel is the senior manager of applied science for Amazon Scout, an autonomous delivery robot that moves along public sidewalks at a walking pace and is currently being field-tested in four U.S. states.
Amazon Science: There are a lot of differences between the problems you’re addressing, but I wondered what the commonalities are.
Sidd Srinivasa: The thing that makes our problem incredibly hard is that we live in an open world. We don't even know what the inputs that we might face are. In our fulfillment centers, I need to manipulate over 20 million items, and that increases by several hundreds of thousands every day. Oftentimes, our robots have absolutely no idea what they're picking up, but they need to be able to pick it up carefully without damaging it and package it effortlessly.
Philipp Michel: For Scout, it's the objects we encounter on the sidewalk, as well as the environment. We operate personal delivery devices in four different U.S. states. The weather conditions, lighting conditions — there’s a huge amount of variability that we explicitly wanted to tackle from the get-go to expose ourselves to all of those difficult, difficult problems.
Tye Brady: For the development of our fulfillment robotics, we have a significant advantage in that we operate in a semi-structured environment. We get to set the rules of the road. Knowing the environment really helps our scientists and engineers contextualize and understand the objects we have to move, manipulate, sort, and identify to fulfill any order. This is a significant advantage in that it gives us real-world project context to pursue our plans for technology development
Philipp Michel: Another commonality, if it isn't obvious, is that we rely very heavily on learning from data to solve our problems. For Scout, that is all of the real-world data that the robot receives on its missions, which we continuously try to iterate on to develop machine learning solutions for perception, for localization to a degree, and eventually for navigation as well.
Sidd Srinivasa: Yeah, I completely agree with that. I think that machine learning and adaptive control are critical for superlinear scaling. If we have tens, hundreds, thousands of robots deployed, we can't have tens, hundreds, thousands of scientists and engineers working on them. We need to scale superlinearly with respect to that.
And I think the open world compels us to think about continual learning. Our machine learning models are trained on some input data distribution. But because of an open world, we have what's called covariate shift, which is that the data that you see doesn't match the distribution, and that causes your machine learning model often to be unreasonably overconfident.
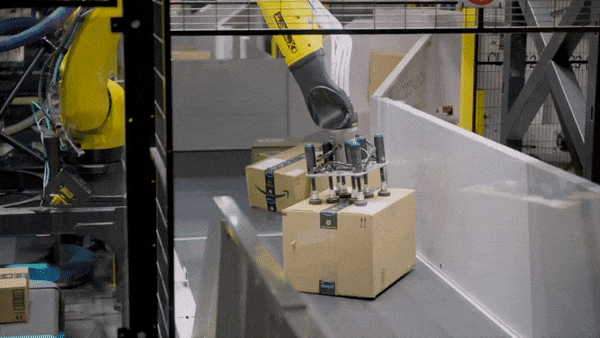
So a lot of work that we do is on creating watchdogs that can identify when the input data distribution has deviated from the distribution that it was trained on. Secondly, we do what we call importance sampling such that we can actually pick out the pieces that have changed and retrain our machine learning models.
Philipp Michel: This is again one of the reasons why we want to have this forcing function of being in a wide variety of different places, so we get exposed to those things as quickly as possible and so that it forces us to develop solutions that handle all of that novel data.
Sidd Srinivasa: That's a great point that I want to continue to highlight. One of the advantages of having multiple robots is the ability for one system to identify that something has changed, to retrain, and then to share that knowledge to the rest of the robots.
We have an anecdote of that in one of our picking robots. There was a robot in one part of the world that noticed a new package type that came by. It struggled mightily at the beginning because it had never seen that and identified that it was struggling. The solution was rectified, and then it was able to transmit the model to all the other robots in the world such that even before this new package type arrived in some of those locations, those robots were prepared to address it. So there was a blip, but that blip occurred only in one location, and all the other locations were prepared to address that because this system was able to retrain itself and share that information.
Philipp Michel: Our bots do similar things. If there are new types of obstacles that we haven't encountered before, we try to adjust our models to recognize those and handle those, and then that gets deployed to all of the bots.
One of the things that keeps me up at night is that we encounter things on the sidewalk that we may not see again for three years. Specific kinds of stone gargoyles used as Halloween decorations on people’s lawns. Or somebody deconstructed a picnic table that had an umbrella, so it is not recognizable as a picnic table to any ML [machine learning] algorithm.
One of the advantages of having multiple robots is the ability to identify that something has changed, to retrain, and then to share that knowledge to the rest of the robots.
So some of our scientific work is on how we balance between generic things that detect that there is something you should not be driving over and things that are quite specific. If it's an open manhole cover, we need to get very good at recognizing that. Whereas if it's just some random box, we might not need a specific hierarchy of boxes — just that it is something that we should not be traversing.
Sidd Srinivasa: Another challenge is that when you do change your model, it can have unforeseen consequences. Your model might change in some way that perhaps doesn't affect your perception but maybe changes the way your robot brakes, and that leads to the wearing of your ball bearings two months from now. We work with these end-to-end systems, where a lot of interesting future research is in being able to understand the consequences of changing parts of the system on the entire system performance.
Philipp Michel: We spent a lot of time thinking about to what degree we should compartmentalize the different parts of the robot stack. There are lots of benefits to trying to be more integrative across them. But there's a limit to that. One extreme is the cameras-to-motor-torques kind of learning that is very challenging in any real-world robotics application. And then there is the traditional robotics stack, which is well separated into localization, perception, planning, and controls.
We also spend a lot of time thinking about how the stack should evolve over time. What performance gains can we get when we more tightly couple some of these parts? At the same time, we want to have a system that remains as explainable as possible. A lot of thought goes into how we can leverage more integration of the learned components across the stack while at the same time retaining the amounts of explainability and safety functionality that we need.
Sidd Srinivasa: That's a great point. I completely agree with Philipp that one model to rule them all may not necessarily be the right answer. But oftentimes we end up building machine learning models that share a common backbone but have multiple heads for multiple applications. What an object is, what it means to segment an object, might be similar for picking or stowing or for packaging, but then each of those might require specialized heads that sit on top of a backbone for those specialized tasks.
Philipp Michel: Some factors we consider are battery, range, temperature, space, and compute limitations. So we need to be very efficient in the models that we use and how we optimize them and how we try to leverage as much shared backbone across them as possible with, as Sidd mentioned, different heads for different tasks.
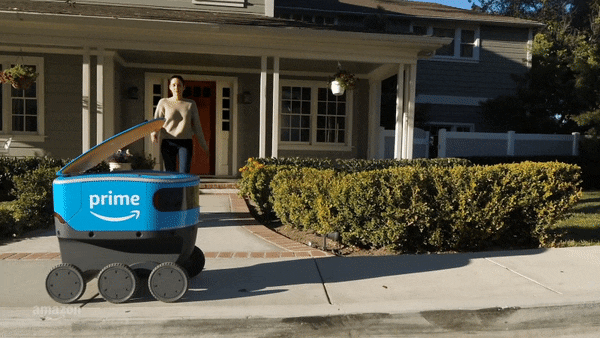
Tye Brady: The nice thing about what Sidd and Philipp describe is that there is always a person to help. The robot can ask another robot through AWS for a different sample or perspective, but the true power comes from asking one of our employees for help in how to perceive or problem-solve. This is super important because the robot can learn from this interaction, allowing our employees to focus on higher-level tasks, things you and I would call common sense. That is not so easy in the robotics world, but we are working to design our machines to understand intent and redirection to reinforce systemic models our robots have of the world. All three of us have that in common.
Amazon Science: When I asked about the commonalities between your projects, one of the things I was thinking about is that you all have robots that are operating in the same environments as humans. How does that complicate the problem?
Tye Brady: When we design our machines right, humans never complicate the problem; they only make it easier. It is up to us to make machines that enhance our human environment by providing a safety benefit and a convenience to our employees. A well-designed machine may fill a deficit for employees that’s not possible without a machine. Either way, our robotics should make us more intelligent, more capable, and freer to do the things that matter most to us.
Philipp Michel: Our direct interactions with our customers and the community are of utmost importance for us. So there's a lot of work that we do on the CX [customer experience] side in trying to make that as delightful as possible.
Another thing that's important for us is that the robot has delightful and safe and understandable interactions with people who might not be customers but whom the robot encounters on its way. People haven't really been exposed to autonomous delivery devices before. So we think a lot about what those interactions should look like on the sidewalk.
A big part of our identity is not just the appearance but how it manifests it through its motion and its yielding behaviors
On the one hand, you should try to act as much as a normal traffic participant would as possible, because that's what people are used to. But on the other hand, people are not used to this new device, so they don't necessarily assume it's going to act like a pedestrian. It's something that we constantly think about. And that's not just at the product level; it really flows down to the bot behavior, which ultimately is controlled by the entire stack. A big part of our identity is not just the appearance but how it manifests it through its motion and its yielding behaviors and all of those kinds of things.
Sidd Srinivasa: Our robots are entering people's worlds. And so we have to be respectful of all the complicated interactions that happen inside our human worlds. When we walk, when we drive, there is this complex social dance that we do in addition to the tasks that we are performing. And it's important for our robots, first of all, to have awareness of it and, secondly, to participate in it.
And it's really hard, I must say. When you're driving, it's sometimes hard to tell what other people are thinking about. And then it's hard to decide how you want to act based on what they're thinking about. So just the inference problem is hard, and then closing the loop is even harder.
If you're playing chess or go against a human, then it's easier to predict what they're going to do, because the rules are well laid out. If you play assuming that your opponent is optimal, then you're going to do well, even if they are suboptimal. That's a guarantee in certain two-player games.
But that's not the case here. We're playing this sort of cooperative game of making sure everybody wins. And when you're playing these sorts of cooperative games, then it's actually very, very hard to predict even the good intentions of the other agents that you're working with.
Philipp Michel: And behavior varies widely. We have times when pets completely ignore the robot, could not care at all, and we have times when the dog goes straight towards the bot. And it's similar with pedestrians. Some just ignore the bot, while others come right up to it. Particularly kids: they’re super curious and interact very closely. We need to be able to handle all of those types of scenarios safely. All of that variability makes the problem super exciting.
Tye Brady: It is an exciting time to be in robotics at Amazon! If any roboticists are out there listening, come join us. It's wicked awesome.