Through smart-home devices and systems, customers can already instruct Alexa to do things like open garage doors, turn lights on and off, or start the dishwasher. But we envision a future in which AI assistants can help with a broader range of more-complex tasks, including performing day-to-day chores, such as preparing breakfast.
To accomplish such tasks, AI assistants will need to interact with objects in the world, understand natural-language instructions to complete tasks, and engage in conversations with users to clarify ambiguous instructions.
Amazon launches new Alexa Prize SimBot Challenge
Today, Amazon also announced the Alexa Prize SimBot Challenge, a competition focused on helping develop next-generation virtual assistants that will assist humans in completing real-world tasks. One of the TEACh benchmarks will be the basis of the challenge's public-benchmark phase.
To aid in the development of such AI assistants, we have publicly released a new dataset called TEACh, for Task-driven Embodied Agents that Chat. TEACh contains over 3,000 simulated dialogues, in which a human instructs a robot in the completion of household tasks, and associated visual data from a simulated environment.
For each dialogue, the roles of human and robot were played by paid crowd workers. The worker playing the robot did not know what task needed to be completed but depended entirely on the other worker’s instructions. Each worker received a visual feed that reflected a first-person point of view on the simulated environment. Both workers could move freely through the environment, but only the robot could interact with objects. The workers needed to collaborate and communicate to successfully complete tasks.
The simulated home environment is based on the AI2-THOR simulator, which includes 30 variations on each of four types of rooms: kitchens, living rooms, bedrooms, and bathrooms. Each gameplay session in the dataset consists of the initial and final states of the simulated environment, a task defined in terms of object properties to be satisfied, and a sequence of actions taken by the crowd workers.
Those actions could include movement through the environment, interactions with objects (the robot can pick and place objects, open and close cabinets, drawers, and appliances, toggle lights on and off, operate appliances and faucets, slice objects, and pour liquid out of one object into another).
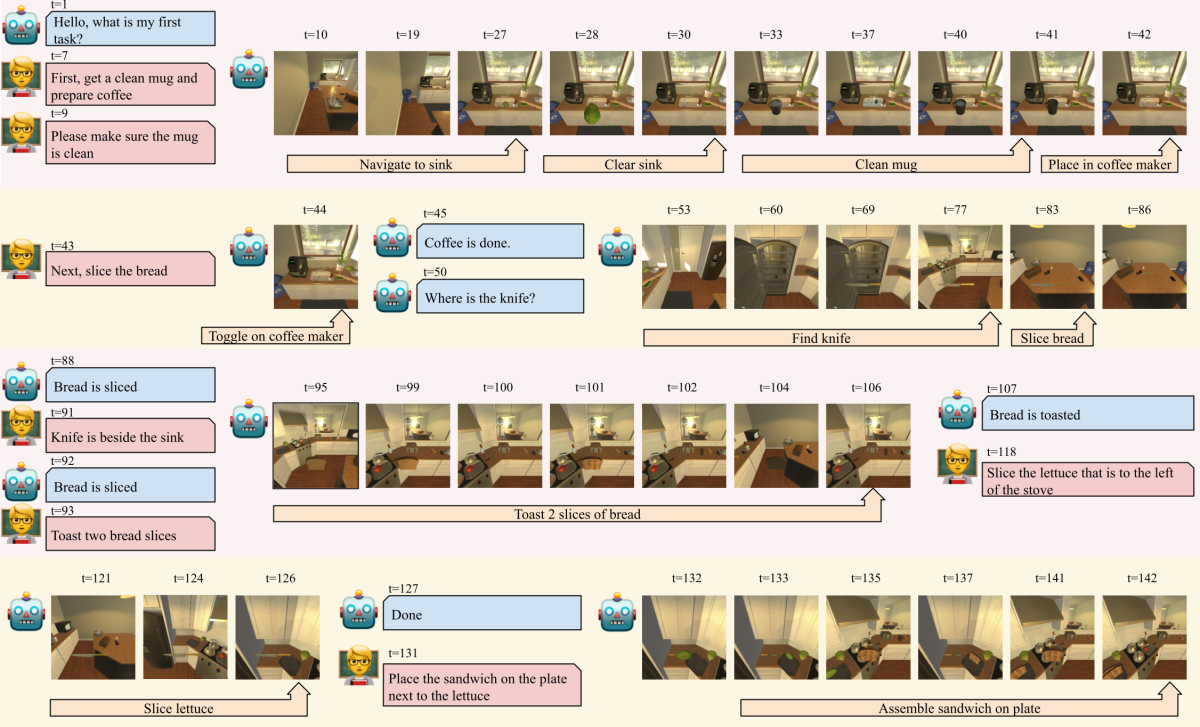
Data collection
To collect the dataset, we first developed a task definition language that let us specify what properties needed to be satisfied in the environment for a task to be considered complete. For example, to check that coffee is made, we confirm that there exists a clean mug in the environment that is filled with coffee. We implement a framework to check the AI2-THOR simulator for the status of different tasks, and we provide natural-language prompts for the steps remaining to complete a task.
We then pair two crowd workers using a web interface and place them in the same simulated room. The user can see the prompts describing what steps need to be completed and uses chat to communicate them to the robot. Additionally, the user can determine where important objects are by either clicking on the steps or searching the virtual space, so that, for example, the robot does not have to open every drawer in the kitchen to find a knife hidden in one of them.
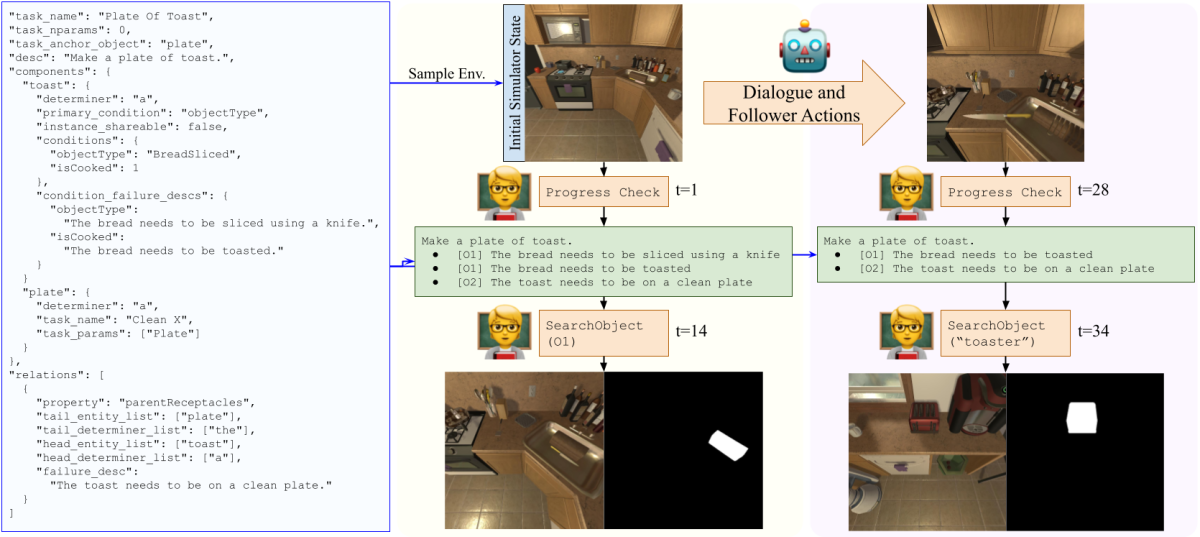
We place no constraints on the chat interface used by the annotators, and as a result, users provide instructions with different levels of granularity. One might say, “First get a clean mug and prepare coffee,” while another might break this up into several steps — “Grab the dirty mug out of the fridge”, “go wash it in the sink”, “place mug in coffee maker” — waiting for the robot to complete each step before providing the next one.
A user might provide instructions too early — for example, asking the robot to slice bread before it has finished preparing coffee — or too late — telling the robot where the knife is only after it has found it and sliced the bread with it. The user might also help the robot correct mistakes or get unstuck — for example, asking the robot to clear out the sink before placing a new object in it.
In total, we collected 4,365 sessions, of which 3,320 were successful. Of those, we were able to successfully replay 3,047 on the AI2-THOR simulator, meaning that providing the same sequence of actions resulted in the same simulator state. TEACh sessions span all 30 kitchens in the simulator and most of the living rooms, bedrooms, and bathrooms. The successful TEACh sessions span 12 task types and consist of more than 45,000 utterances, with an average of 8.40 user and 5.25 robot utterances per session.
Benchmarks
We propose three benchmark tasks that machine learning models can be trained to perform using our dataset: execution from dialogue history (EDH), trajectory from dialogue (TfD), and two-agent task completion (TATC).
In the EDH benchmark, the model receives some dialogue history, previous actions taken by the robot, and the corresponding first-person observations from a collected gameplay session. The model is expected to predict the next few actions the robot will take, receiving a first-person observation after each action. The model is judged on whether its actions yield the same result that the player’s actions did in the original gameplay session.
The EDH benchmark will also be the basis for the public-benchmark phase of the Alexa Prize SimBot Challenge, which we also announced today. The SimBot Challenge is focused on helping advance development of next-generation virtual assistants that will assist humans in completing real-world tasks by continuously learning and gaining the ability to perform commonsense reasoning.
In the TfD benchmark, a model receives the complete dialogue history and has to predict all the actions taken by the robot, receiving a first-person observation after each action.
In the TATC benchmark, the designer needs to build two models, one for the user and one for the robot. The user model receives the same task information that the human worker did, as well as the state of the environment. It has to communicate with the robot model, which takes actions in the environment to complete tasks.
We include baseline model performance on these benchmarks in a paper we’ve published to the arXiv, which we hope will be used as a reference for future work by other research groups.
For the EDH and TfD benchmarks, we created “validation-seen” and “test-seen” splits, which evaluate the ability of models to generalize to new dialogues and execution paths in the rooms used for training, and “validation-unseen” and “test-unseen” splits, which evaluate the ability of models to generalize to dialogues and execution paths in rooms never previously seen. These splits are designed to enable easy model transfer to and from a related dataset, ALFRED, which also uses floorplans from AI2-THOR and splits the data similarly.
Acknowledgements: This project came together through the efforts and support of several people on the Alexa AI team. We would like to thank Jesse Thomason, Ayush Shrivastava, Patrick Lange, Anjali Narayan-Chen, Spandana Gella, Robinson Piramuthu, Gokhan Tur, Dilek Hakkani-Tür, Ron Rezac, Shui Hu, Lucy Hu, Hangjie Shi, Nicole Chartier, Savanna Stiff, Ana Sanchez, Ben Kelk, Joel Sachar, Govind Thattai, Gaurav Sukhatme, Joel Chengottusseriyil, Tony Bissell, Qiaozi Gao, Kaixiang Lin, Karthik Gopalakrishnan, Alexandros Papangelis, Yang Liu, Mahdi Namazifar, Behnam Hedayatnia, Di Jin, and Seokhwan Kim for their contributions to the project.