This post is an adaptation of a keynote address that Leo de Moura delivered at the International Conference on Computer Aided Verification (CAV), in July 2024.
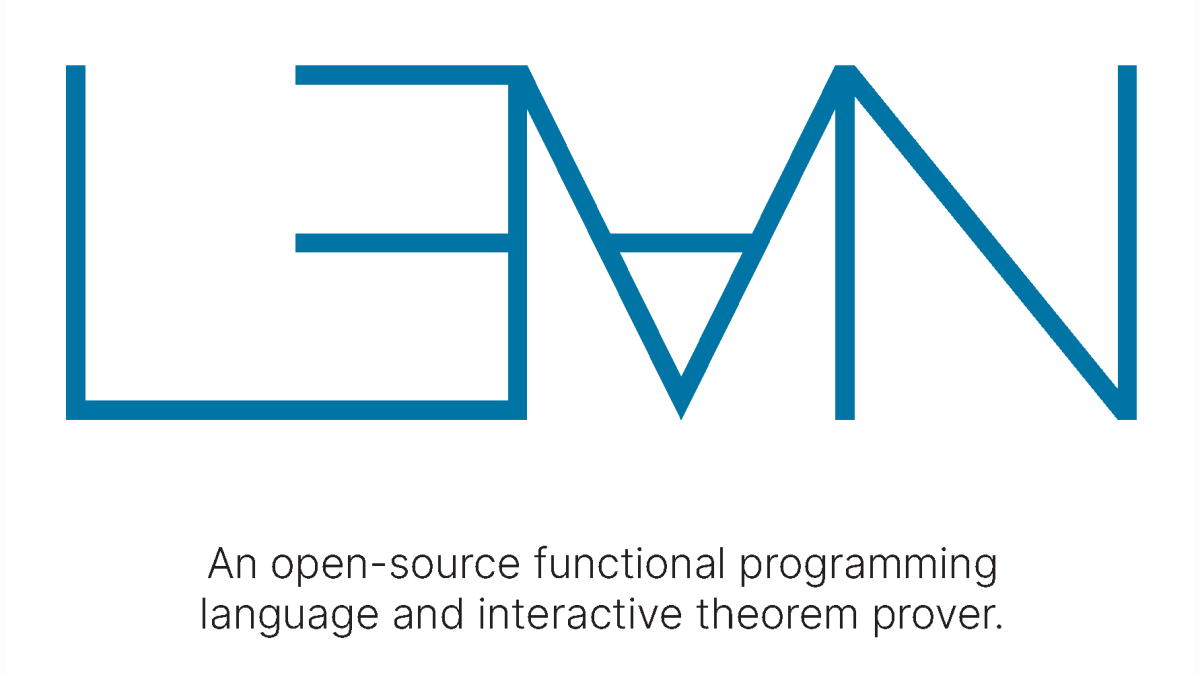
In 2013, I launched the Lean project with the goal of bridging the gap between automated and interactive theorem provers. Since its inception, Lean has seen unparalleled adoption in the mathematical community, surpassing previous efforts in formalized mathematics. Lean 4, the latest version, is implemented in Lean itself and is also a fully fledged, extensible programming language with robust IDE support, package management, and a thriving ecosystem.
In 2023, Sebasian Ullrich and I founded the Lean Focused Research Organization (FRO), a nonprofit dedicated to advancing Lean and supporting its community. The Lean project embraces a philosophy that promotes decentralized innovation, empowering a diverse community of researchers, developers, and enthusiasts to collaboratively push the boundaries of mathematical practice and software development. In this blog post, we will provide a brief introduction to the project and describe how it is used at AWS.
A brief introduction to Lean
Lean is an open-source, extensible, functional programming language and interactive theorem prover that makes writing correct and maintainable code easy. Lean programming primarily involves defining types and functions, allowing users to focus on the problem domain and its data rather than on coding details. Lean has four primary use cases: formal mathematics, software and hardware verification, AI for math and code synthesis, and math and computer science education.
Formal mathematics
Lean allows mathematicians to work with advanced mathematical structures using syntax that feels natural to them. The math community recognizes its usefulness: for instance, Fields medalists Peter Scholze and Terence Tao used Lean to confirm their new results; Quanta Magazine has lauded Lean as one of the biggest breakthroughs in mathematics, and it has been featured in numerous popular scientific and academic publications, including the Wired magazine article “The effort to build the mathematical library of the future”. Recently, DeepMind used Lean to build an AI engine that met the silver-medal standard at the International Math Olympiad.
As of July 2024, the Lean Mathematical Library has received contributions from over 300 mathematicians and contains 1.58 million lines of code, surpassing other formal-mathematics systems in use. This remarkable growth has come despite Lean’s concision and youth: it’s at least a decade younger than comparable libraries.
Software and hardware verification
Lean’s combination of formal verification, user interaction, and mathematical rigor makes it invaluable for both software and hardware verification. Lean is a system for programming your proofs and proving your programs. An additional benefit is that Lean produces efficient code, and its extensibility features, originally designed for mathematicians, are also highly convenient for creating abstractions when writing clean and maintainable code. Its benefits extend to any system requiring exceptional accuracy and security, including industries such as aerospace, cryptography, web services, autonomous vehicles, biomedical systems, and medical devices. Later on, we will provide several examples of Lean's applications at AWS.
AI for math and code synthesis
Lean is popular with groups developing AI for mathematics and code synthesis. One of the key reasons is that Lean formal proofs are machine checkable and can be independently audited by external proof checkers. Additionally, Lean's extensibility allows users to peer into the system internals, including data structures for representing proofs and code. This capability is also used to automatically generate animations from Lean proofs.
AI researchers are leveraging large language models (LLMs) to create Lean formal proofs and automatically translate prose into formalized mathematics. OpenAI has released lean-gym, a reinforcement learning environment based on Lean. Harmonic used Lean in the development of its Mathematical Superintelligence Platform (MSI), an AI model designed to guarantee accuracy and avoid hallucinations. Meta AI created an AI model that has solved 10 International Mathematical Olympiad problems, and DeepMind has formalized a theoretical result related to AI safety in Lean. Additionally, LeanDojo is an open-source project using LLMs to automate proof construction in Lean.
Lean's unique combination of machine-checkable proofs, system introspection, and extensibility makes it an ideal tool for advancing AI research in mathematics and code synthesis. The synergy between LLMs and Lean formal proofs is emphasized in Terence Tao's colloquium lecture at the American Mathematical Society, “Machine Assisted Proof”; in the Scientific American article “AI will become mathematicians' co-pilot”; and in the New York Times article “A.I. Is coming for mathematics, too.”
Math and CS education
Millions of people learn mathematics as students and use it throughout their careers. Since its inception, the Lean project has supported students' mathematical-reasoning needs and enabled a more diverse population to contribute to the fields of math and computer science. Numerous educational resources are available for learning Lean, including interactive computer games such as the Natural Number Game, computer science and mathematics textbooks, university courses, and on-demand tutorials. The Lean FRO is committed to expanding Lean’s educational content and envisions a future where children use Lean as a playground for learning mathematics, progressing at their own paces and receiving instantaneous feedback, similar to how many have learned to code.
A quick tour of Lean
Lean combines programming and formal verification. Let's take a quick tour through a small example to see how we write code in Lean and prove properties about that code.
Writing code in Lean
First, let's define a simple function that appends two lists:
def append (xs ys : List a) : List a := match xs with | [] => ys | x :: xs => x :: append xs ys
This function is defined using pattern matching. For the base case, appending an empty list [] to ys results in ys. The notation x :: xs represents a list with head x and tail xs. For the recursive case, appending x :: xs to ys results in x :: append xs ys. Additionally, the append function is polymorphic, meaning it works with lists of any type a.
Extensible syntax
The notation x :: xs used above is not built into Lean but is defined using the infixr command:
infixr:67 " :: " => List.cons
The infixr command defines a new infix operator x :: xs, denoting List.cons x xs. This command is actually a macro implemented using Lean's hygienic macro system. Lean's extensible syntax allows users to define their own domain-specific languages. For example, Verso, the Lean documentation-authoring system, is implemented in Lean using this mechanism. Verso defines alternative concrete syntaxes that closely resemble Markdown and HTML.
Proving properties about code
Next, we'll prove a property about our append function: that the length of the appended lists is the sum of their lengths.
theorem append_length (xs ys : List a) : (append xs ys).length = xs.length + ys.length := by induction xs with | nil => simp [append] | cons x xs ih => simp [append, ih]; omega
Here, theorem introduces a new theorem named append_length. The statement (append xs ys).length = xs.length + ys.length is what we want to prove. The by ... block contains the proof. In this proof,
- induction xs with initiates a proof by induction on xs;
- the nil case proves the base case using simp, the Lean simplifier. The parameter append instructs the simplifier to expand append’s definition; and
- the cons x xs ih case proves the inductive step where ih is the inductive hypothesis. It also uses simp and omega, which complete the proof using arithmetical reasoning.
In this proof, induction, simp, and omega are tactics. Tactics, which transform one state of the proof into another, are key to interactive theorem proving in Lean. Users can inspect the states of their proofs using the Lean InfoView, a panel in the IDE. The InfoView is an interactive object that can be inspected and browsed by the user. In the following picture, we see the state of our proof before the simp tactic at line 10. Note that the proof state contains all hypotheses and the goal (append (x :: xs) ys).length = (x :: xs).length + ys.length, which remains to be proved.
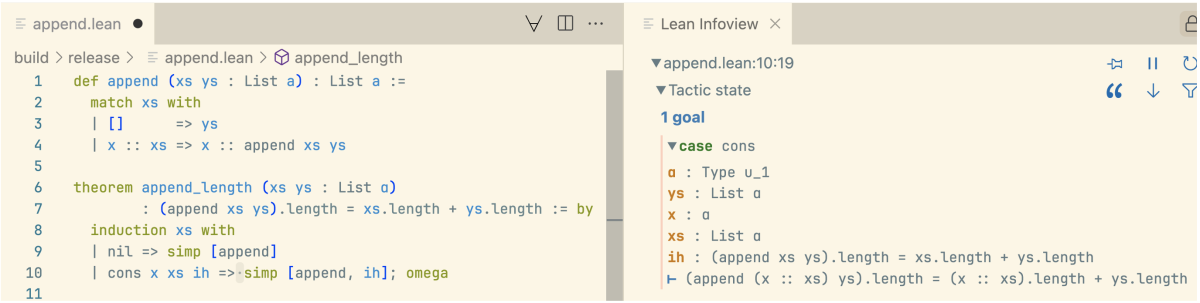
How Lean is used at AWS
At AWS, Lean is used in several open-source projects to address complex verification and modeling challenges. These projects not only highlight the practical applications of Lean in different domains but also emphasize AWS's commitment to open-source development and collaboration. We cover four key projects: Cedar, LNSym, and SampCert, whose Lean source code is already available on GitHub, and AILean, which is exploring the relationship between LLMs and formal mathematics and whose code is not open source yet.
Cedar: an open-source policy language and evaluation engine
Cedar is an open-source policy language and evaluation engine. Cedar enables developers to express fine-grained permissions as easy-to-understand policies enforced in their applications and to decouple access control from application logic. Cedar supports common authorization models such as role-based access control and attribute-based access control. It is the first policy language built from the ground up to be verified formally using automated reasoning and tested rigorously using differential random testing.
The Cedar project uses Lean to create an executable formal model of each core component of the Cedar runtime (such as the authorization engine) and static-analysis tools (such as the type checker). This model serves as a highly readable specification, allowing the team to prove key correctness properties using Lean.
Lean was chosen for modeling Cedar due to its fast runtime, extensive libraries, IDE support, and small trusted computing base (TCB). The fast runtime enables efficient differential testing of Cedar models. The libraries provide reusable verified data structures and tactics built by the open-source community. Lean’s small TCB allows Cedar to leverage these contributions confidently, as Lean checks their correctness, requiring trust only in Lean’s minimal proof-checking kernel.
LNSym: Symbolic simulation for cryptographic verification
LNSym is a symbolic simulator for Armv8 native-code programs. It’s currently under development, with a focus on enabling automated reasoning of cryptographic machine-code programs. Many cryptographic routines are written in assembly to optimize performance and security on the underlying processor. LNSym aims to reduce the cost of verifying cryptographic routines, particularly block ciphers and secure hashes, ultimately empowering cryptography developers to formally reason about their native-code programs.
LNSym uses Lean as a specification language to model the Arm instruction semantics and cryptographic protocols and as a theorem prover for reasoning about these artifacts. Since Lean programs are executable, the specifications achieve a high degree of trust through thorough conformance testing. Lean orchestrates proofs such that the heavy and often tedious lifting is done automatically, using decision procedures like SAT solvers or custom domain-specific tactics. When proof automation fails, users can employ Lean as an interactive theorem prover. This combination of interactive and automated theorem proving ensures that progress on verification tasks is not hindered by the limitations of proof automation.
SampCert: formally verified differential-privacy primitives
SampCert is an open-source library of formally verified differential-privacy primitives used by the AWS Clean Rooms Differential Privacy service for its fast and sound sampling algorithms. Using Lean, SampCert provides the only verified implementation of the discrete Gaussian sampler and the primitives of zero concentrated differential privacy.
Although SampCert focuses on software, its verification relies heavily on Mathlib, the Lean Mathematical Library. The verification of code addressing practical problems in data privacy depends on the formalization of mathematical concepts from Fourier analysis to number theory and topology.
AILean: AI for math and math for AI
AILean is exploring the relationship between LLMs and formal mathematics in collaboration with the Technology Innovation Institute (TII). This exploration works in both directions: AI for math and math for AI. In AILean, LLMs are used to enhance proof automation and user experience in formal mathematics. LLMs can analyze theorem statements and existing proof steps, suggesting relevant lemmas, definitions, or tactics to guide users in completing proofs. They can also identify common mistakes or inconsistencies, proposing corrections or alternative approaches that avoid dead ends and thereby improving the proof development process.
Takeaways
Lean is a complex system, but its correctness relies only on a small trusted kernel. Moreover, all proofs and definitions can be exported and independently audited and checked. This is a crucial feature for both the mathematical and software verification communities because it eliminates the trust bottleneck. It doesn't matter who you are; if Lean checked your proof, the whole world can build on top of it. This enables large groups of mathematicians who have never met to collaborate and work together. Additionally, it allows users to extend Lean without fearing the introduction of soundness bugs that could compromise the logical consistency of the system.
Lean's extensibility enables customization, which was particularly important during its first ten years, when resources were limited. Lean’s extensibility allowed the community to extend the system without needing to synchronize with its developers. Self-hosting, or implementing Lean in Lean, also ensured that users can access all parts of the system without having to learn a different programming language. This makes it easy and convenient to extend Lean. Packages such as ProofWidgets and SciLean are excellent examples of user-defined extensions that leverage these features.
The FRO model introduced by Convergent Research has been instrumental in supporting Lean and helping it transition to a self-sufficient foundation. The Lean project has grown significantly, and driving it forward would have been difficult without Convergent Research’s efforts to secure philanthropic support. Just as foundations like the Rust and Linux Foundations are vital for the success and sustainability of open-source projects, the support of Convergent Research has been critical for Lean's ongoing progress.
To learn more about Lean, visit the website.