Most secure transactions online are protected by public-key encryption schemes like RSA, whose security depends on the difficulty of factoring large numbers. Public-key encryption improves security because it enables the encrypted exchange of private keys. But because it depends on operations like modular exponentiation of large integers, it introduces significant computational overhead.
Researchers and engineers have introduced all kinds of optimizations to make public-key encryption more efficient, but the resulting complexity makes it difficult to verify that the encryption algorithms are behaving properly. And a bug in an encryption algorithm can be disastrous.
This post explains how Amazon’s Automated Reasoning group improved the throughput of RSA signatures on Amazon’s Graviton2 chip by 33% to 94%, depending on the key size, while also proving the functional correctness of our optimizations using formal verification.
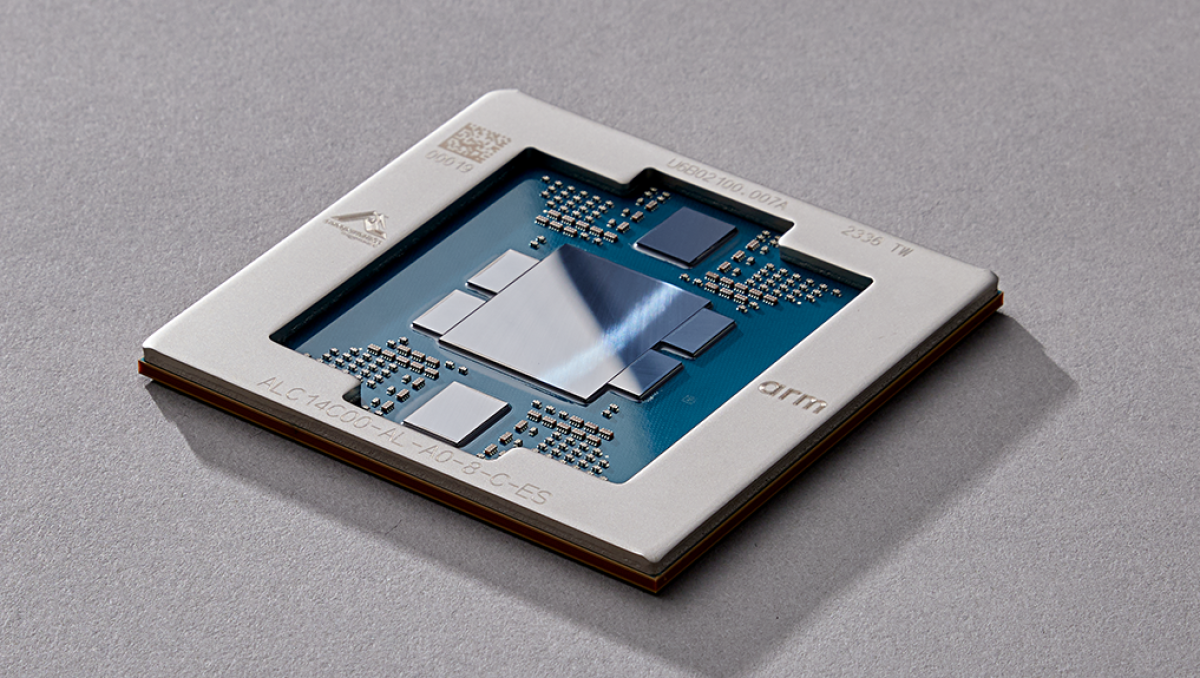
Graviton2 is a server-class CPU developed by Amazon Annapurna Labs, based on Arm Neoverse N1 cores. To improve the throughput of RSA signatures on Graviton2, we combined various techniques for fast modular arithmetic with assembly-level optimizations specific to Graviton2. To show that the optimized code is functionally correct, we formally verified it using the HOL Light interactive theorem prover, which was developed by a member of our team (John Harrison).
Our code is written in a constant-time style (for example, no secret-dependent branches or memory access patterns) to avoid side-channel attacks, which can learn secret information from operational statistics like function execution time. The optimized functions and their proofs are included in Amazon Web Services’ s2n-bignum library of formally verified big-number operations. The functions are also adopted by AWS-LC, the cryptographic library maintained by AWS, and by its bindings Amazon Corretto Crypto Provider (ACCP) and AWS Libcrypto for Rust (AWS-LC-RS).
Key size (bits) | Baseline throughput (ops/sec) | Improved throughput (ops/sec) | Speedup (%) |
2048 | 299 | 541 | 81.00% |
3072 | 95 | 127 | 33.50% |
4096 | 42 | 81 | 94.20% |
Improvements in the throughput times of RSA signatures in AWS-LC on Graviton2.
Step 1. Making RSA fast on Graviton2
Optimizing the execution of RSA algorithms on Graviton2 requires the careful placement and use of multiplication instructions. On 64-bit Arm CPUs, the multiplication of two 64-bit numbers, with a product of up to 128 bits (conventionally designated 64×64→128), are accomplished by two instructions: MUL, producing the lower 64 bits, and UMULH, producing the upper 64 bits. On Graviton2, MUL has a latency of four cycles and stalls the multiplier pipeline for two cycles after issue, while UMULH has a latency of five cycles and stalls the multiplier pipeline for three cycles after issue. Since Neoverse N1 has a single multiplier pipeline but three addition pipelines, multiplication throughput is around one-tenth the throughput of 64-bit addition.
To improve throughput, we (1) applied a different multiplication algorithm, trading multiplication for addition instructions, and (2) used single-instruction/multiple-data (SIMD) instructions to offload a portion of multiplication work to the vector units of the CPU.
Algorithmic optimization
For fast and secure modular arithmetic, Montgomery modular multiplication is a widely used technique. Montgomery multiplication represents numbers in a special form called Montgomery form, and when a sequence of modular operations needs to be executed — as is the case with the RSA algorithm — keeping intermediary products in Montgomery form makes computation more efficient.
We implement Montgomery multiplication as the combination of big-integer multiplication and a separate Montgomery reduction, which is one of its two standard implementations.
On Graviton2, the benefit of this approach is that we can use the well-known Karatsuba algorithm to trade costly multiplications for addition operations. The Karatsuba algorithm decomposes a multiplication into three smaller multiplications, together with some register shifts. It can be performed recursively, and for large numbers, it’s more efficient than the standard multiplication algorithm.
We used Karatsuba’s algorithm for power-of-two bit sizes, such as 2,048 bits and 4,096 bits. For other sizes (e.g., 3072 bits), we still use a quadratic multiplication. The Karatsuba multiplication can be further optimized when the two operands are equal, and we wrote functions specialized for squaring as well.
With these optimizations we achieved a 31–49% speedup in 2,048- and 4,096-bit RSA signatures compared with our original code.
Microarchitectural optimization
Many Arm CPUs implement the Neon single-instruction/multiple-data (SIMD) architecture extension. It adds a file of 128-bit registers, which are viewed as vectors of various sizes (8/16/32/64 bit), and SIMD instructions that can operate on some or all of those vectors in parallel. Furthermore, SIMD instructions use different pipelines than scalar instructions, so both types of instructions can be executed in parallel.
Vectorization strategy. Vectorization is a process that replaces sequential executions of the same operation with a single operation over multiple values; it usually increases efficiency. Using SIMD instructions, we vectorized scalar 64-bit multiplications.
For big-integer multiplication, vectorized 64-bit multiply-low code nicely overlapped with scalar 64-bit multiply-high instructions (UMULH). For squaring, vectorizing two 64×64→128-bit squaring operations worked well. For multiplications occurring in Montgomery reduction, vectorizing 64×64→128-bit multiplications and 64×64→64 multiply-lows worked. To choose which scalar multiplications to vectorize, we wrote a script that enumerated differently vectorized codes and timed their execution. For short code fragments, exhaustive enumeration was possible, but for larger code fragments, we had to rely on experience. The overall solution was chosen only after extensive experiments with other alternatives, such as those described by Seo et. al. at ICISC’14.
Although the scalar and SIMD units are able to operate in parallel, it is sometimes necessary to move inputs and intermediate results between integer and SIMD registers, and this brings significant complications. The FMOV instruction copies data from a 64-bit scalar register to a SIMD register, but it uses the same pipeline as the scalar multiplier, so its use would reduce scalar-multiplier throughput.
The alternative of loading into a vector register first and then using MOV to copy it to a scalar register has lower latency, but it occupies the SIMD pipeline and hence lowers the throughput of SIMD arithmetic operations. Somewhat counterintuitively, the best solution was to make two separate memory loads into the integer and SIMD registers, with care for their relative placement. We did still use MOV instructions to copy certain SIMD results into integer registers when the SIMD results were already placed at SIMD registers because it was faster than a round trip via store-load instructions.
Fast constant-time table lookup code. Another independent improvement was the reimplementation of a vectorized constant-time lookup table for a fast modular-exponentiation algorithm. Combining this with our earlier optimization further raises our speedup to 80–94% when compared to the throughput of 2,048-/4,096-bit RSA signatures from our initial code, as well as a 33% speedup for 3,072-bit signatures.
Instruction scheduling. Even though Graviton2 is an out-of-order CPU, carefully scheduling instructions is important for performance, due to the finite capacity of components like reorder buffers and issue queues. The implementations discussed here were obtained by manual instruction scheduling, which led to good results but was time consuming.
We also investigated automating the process using the SLOTHY superoptimizer, which is based on constraint solving and a (simplified) microarchitecture model. With additional tweaks to Montgomery reduction to precalculate some numbers used in Karatsuba, SLOTHY optimization enabled a 95–120% improvement on 2,048-/4,096-bit throughputs and 46% on 3,072-bit! However, this method is not yet incorporated into AWS-LC since verifying the automated scheduling proved to be challenging. Studying the potential for automatically proving correctness of scheduling optimizations is a work in progress.
Step 2. Formally verifying the code
To deploy the optimized code in production we need to ensure that it works correctly. Random testing is a cheap approach for quickly checking simple and known cases, but to deliver a higher level of assurance, we rely on formal verification. In this section we explain how we apply formal verification to prove functional correctness of cryptographic primitives.
Introduction to s2n-bignum
AWS’s s2n-bignum is both (1) a framework for formally verifying assembly code in x86-64 and Arm and (2) a collection of fast assembly functions for cryptography, verified using the framework itself.
Specification in s2n-bignum. Every assembly function in s2n-bignum — including the new assembly functions used in RSA — has a specification stating its functional correctness. A specification states that for any program state satisfying some precondition, the output state of the program must satisfy some postcondition. For example, bignum_mul_4_8(uint64_t *z, uint64_t *x, uint64_t *y) is intended to multiply two 256-bit (four-word) numbers producing a 512-bit (eight-word) result. Its (abbreviated) precondition over an input state s is
aligned_bytes_loaded s (word pc) bignum_mul_4_8_mc ∧ read PC s = word pc ∧ C_ARGUMENTS [z, x, y] s ∧ bignum_from_memory (x,4) s = a ∧ bignum_from_memory (y,4) s = b
This means that the machine code of bignum_mul_4_8 is loaded at the address currently contained in the program counter PC (aligned_bytes_loaded), symbolic values are assigned to the function arguments according to C’s application binary interface (C_ARGUMENTS ...), and big integers logically represented by the symbols a and b are stored in the memory location pointed to by x and y for four words (bignum_from_memory ...).
The (abbreviated) postcondition over an output state s is
bignum_from_memory (z,8) s = a * b
This means that the multiplied result a * b is stored in the eight-word buffer starting at location z.
One more component is a relation between the input and output states that must be satisfied:
(MAYCHANGE_REGS_AND_FLAGS_PERMITTED_BY_ABI; MAYCHANGE [memory :> bytes(z,8 * 8)]) (s_in,s_out)
This means that executing the code may change registers/flags permitted by the application binary interface (ABI) and the eight-word buffer starting at z, but all other state components must remain unchanged.
Verifying assembly using HOL Light. To prove that the implementation is correct with respect to the specification, we use the HOL Light interactive theorem prover. In contrast to “black-box” automated theorem provers, tools like HOL Light emphasize a balance between automating routine proof steps and allowing explicit, and programmable, user guidance. When a proof exists on paper or inside someone’s head, a proficient user can effectively rewrite the proof in an interactive theorem prover. S2n-bignum uses a combination of two strategies to verify a program:
Symbolic execution. Given a representation of the input program state using symbolic variables in place of specific values, symbolic execution infers a symbolic output state at the end of some code snippet, in effect doing a more rigorous and generalized form of program execution. While this still leaves the postcondition to be proved, it strips away artifacts of program execution and leaves a purely mathematical problem.
Intermediate annotations in the style of Floyd-Hoare logic. Each intermediate assertion serves as a postcondition for the preceding code and a precondition for the subsequent code. The assertion need contain only the details that are necessary to prove its corresponding postcondition. This abstraction helps make symbolic simulation more tractable, in terms of both automated-reasoning capacity and the ease with which humans can understand the result.
We assume that the Arm hardware behaves in conformance with the model of s2n-bignum, but the model was developed with care, and it was validated by extensively cross-checking its interpretations against hardware.
Future formal-verification improvements. The formal verification for s2n-bignum does not yet cover nonfunctional properties of the implementation, including whether it may leak information through side channels such as the running time of the code. Rather, we handle this through a disciplined general style of implementation: never using instructions having variable timing, such as division, and no conditional branching/memory access patterns that depend on secret data. Also, we sanity-check some of these properties using simple static checks, and we execute the code on inputs with widely differing bit densities to analyze the corresponding run times and investigate any unexpected correlations.
These disciplines and sanity checks are standard practice with us, and we apply them to all the new implementations described here. In ongoing work, we are exploring the possibility of formally verifying the absence of information leakage.