In April, our research team at Amazon open-sourced our PECOS framework for extreme multilabel ranking (XMR), which is the general problem of classifying an input when you have an enormous space of candidate classes. PECOS presents a way to solve XMR problems that is both accurate and efficient enough for real-time use.
At this year’s Knowledge Discovery and Data Mining Conference (KDD), members of our team presented two papers that demonstrate both the power and flexibility of the PECOS framework.
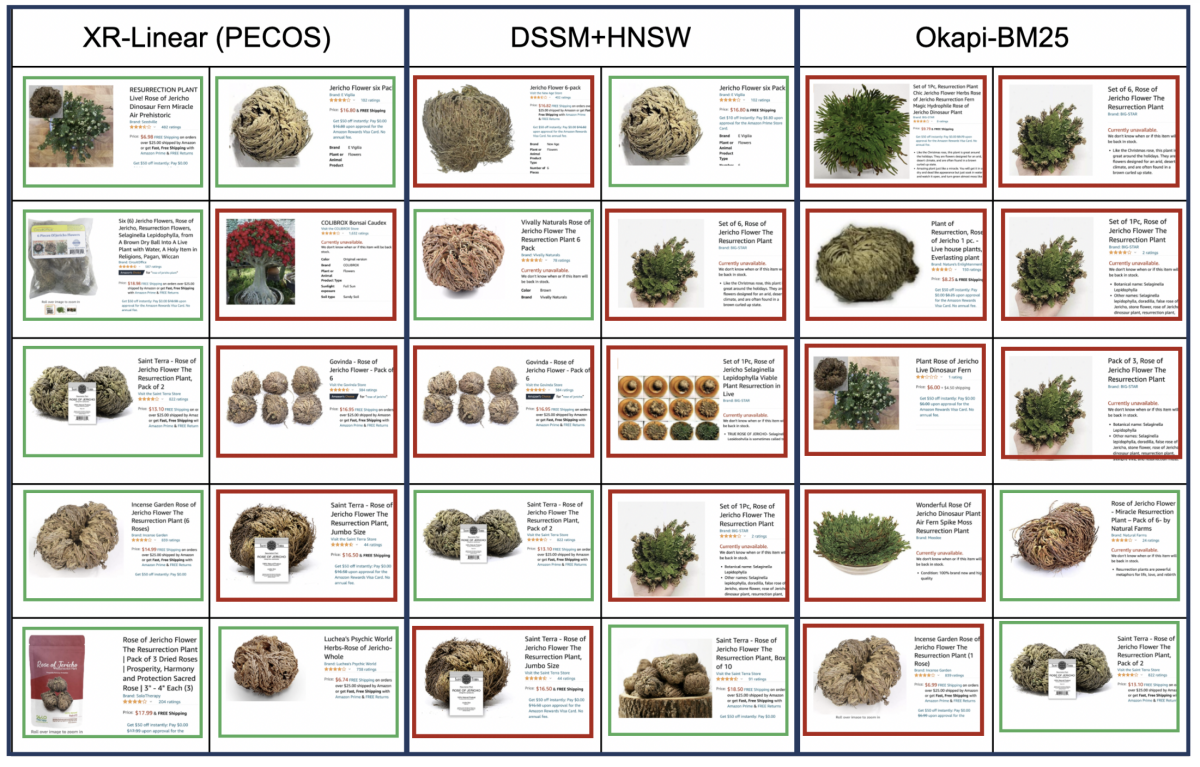
One applies PECOS to the problem of product retrieval, a use case very familiar to customers at the Amazon Store. The other is a less obvious application: session-aware query autocompletion, in which an autocompletion model — which predicts what a customer is going to type — bases its predictions on the customer’s last few text inputs, as well as on statistics for customers at large.
In both cases, we tailor PECOS’s default models to the tasks at hand and, in comparisons with several strong benchmarks, show that PECOS offers the best combination of accuracy and speed.
The PECOS model
The classic case of XMR would be the classification of a document according to a handful of topics, where there are hundreds of thousands of topics to choose from.
We generalize the idea, however, to any problem that, for a given input, finds a few matches from among a large set of candidates. In product retrieval, for instance, the names of products would be “labels” we apply to a query: “Echo Dot”, “Echo Studio”, and other such names would be labels applied to the query “Smart speaker”.
PECOS adopts a three-step solution to the XMR problem. First is the indexing step, in which PECOS groups labels according to topic. Next is the matching step, which matches an input to a topic (which significantly shrinks the space of candidates). Last comes the ranking step, which reranks the labels in the matched topic, based on features of the input.
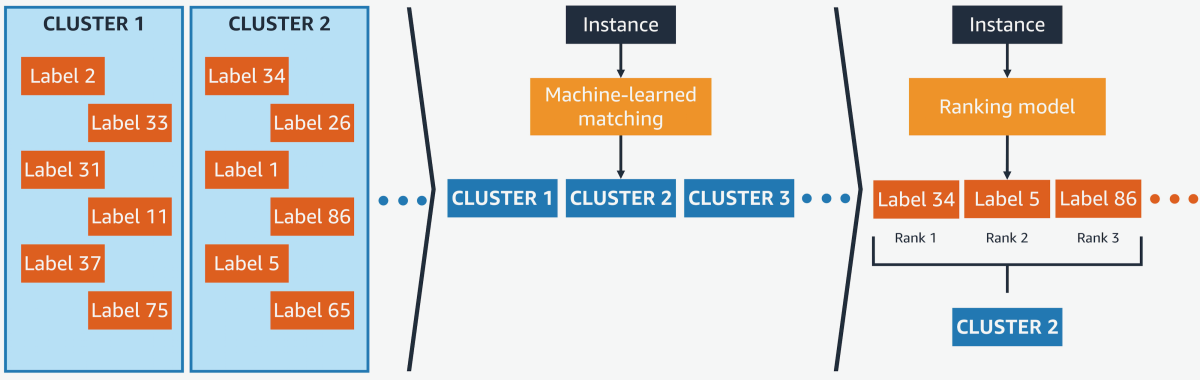
PECOS comes with default models for each of these steps, which we described in a blog post about the April code release. But users can modify those models as necessary, or create their own and integrate them into the PECOS framework.
Product retrieval
For the product retrieval problem, we adapt one of the matching models that comes standard with PECOS: XR-Linear. Details are in the earlier blog post (and in our KDD paper), but XR-Linear reduces computation time by using B-ary trees — a generalization of binary trees to trees whose nodes have B descendants each. The top node of the tree represents the full label set; the next layer down represents B partitions of the full set; the next layer represents B partitions of each partition in the previous layer, and so on.
Connections between nodes of the trees have associated weights, which are multiplied by features of the input query to produce a probability score. Matching is the process of tracing the most-probable routes through the tree and retrieving the topics at the most-probable leaf nodes. To make this process efficient, we use beam search: i.e., at each layer, we limit the number of nodes whose descendants we consider, a limit known as the beam width.
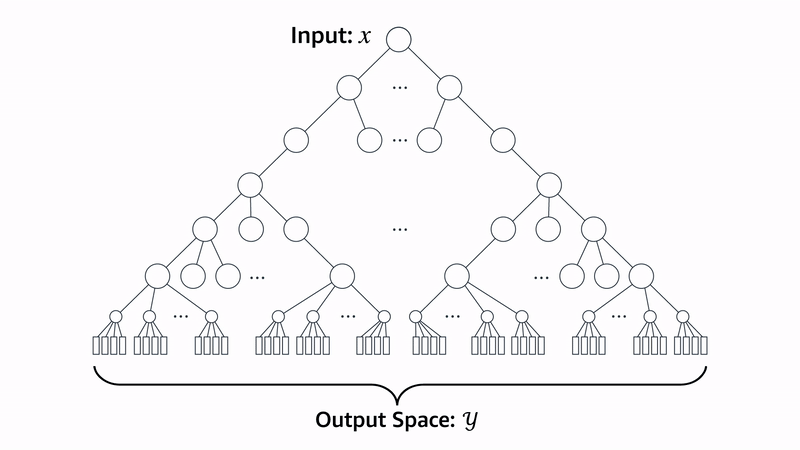
In our KDD paper on product retrieval, we vary this general model through weight pruning; i.e., we delete edges whose weights fall below some threshold, reducing the number of options the matching algorithm has to consider as it explores the tree. In the paper, we report experiments with several different weight thresholds and beam widths.
We also experimented with several different sets of input features. One was n-grams of query words. For instance, the query “Echo with screen” would produce the 1-grams “Echo”, “with”, “screen”, the 2-grams “Echo with” and “with screen”, and the 3-gram “Echo with screen”. This sensitizes the matching model to phrases that may carry more information than their constituent words.
Similarly, we used n-grams of input characters. If we use the token “#” to denote the end of a word, the same query would produce the character trigrams “Ech”, “cho”, “ho#”, “with”, “ith”, and so on. Character n-grams helps the model deal with typos or word variants.
Finally, we also used TF-IDF (term frequency–inverse document frequency) features, which normalize the frequency of a word in a given text by its frequency across all texts (which filters out common words like “the”). We found that our model performed best when we used all three sets of features.
As benchmarks in our experiments, we used the state-of-the-art linear model and the state-of-the-art neural model and found that our linear approach outperformed both, with a recall@10 — that is, the number of correct labels among the top ten — that was more than double the neural model’s and almost quadruple the linear model’s. At the same time, our model took about one-sixth as long to train as the neural model.
We also found that our model took an average of only 1.25 milliseconds to complete each query, which is fast enough for deployment in a real-time system like the Amazon Store.
Session-aware query autocompletion
Session-aware query autocompletion uses the history of a customer’s recent queries — not just general statistics for the customer base — to complete new queries. The added contextual information means that it can often complete queries accurately after the customer has typed only one or two letters.
To frame this task as an XMR problem, we consider the case in which the input is a combination of the customer’s previous query and the beginning — perhaps just a few characters — of a new query. The labels are queries that an information retrieval system has seen before.
In this case, PECOS didn’t work well out of the box, and we deduced that the problem was the indexing scheme used to cluster labels by topic. PECOS’s default indexing model embeds inputs, or converts them into vectors, then clusters labels according to proximity in the vector space.
We suspected that this was ineffective when the inputs to the autocompletion model were partial phrases — fragments of words that a user is typing in. So we experimented with an indexing model that instead used data structures known as tries(a variation on “tree” that borrows part of the word “retrieve”).
A trie is a tree whose nodes represent strings of letters, where each descendant node extends its parent node’s string by one letter. So if the top node of the trie represents the letter “P”, its descendants might represent the strings “PA” and “PE”; their descendants might represent the strings “PAN”, “PAD”, “PEN”, “PET”, and so on. With a trie, all the nodes that descend from a common parent constitute a cluster.
Clustering using tries dramatically improved the performance of our model, but it also slowed it down: the strings encoded by tries can get very long, which means that tracing a path through the trie can get very time consuming.
So we adopted a hybrid clustering technique that combines tries with embeddings. The top few layers of the hybrid tree constitute a trie, but the nodes that descend from the lowest of these layers represent strings whose embeddings are near that of the parent node in the vector space.
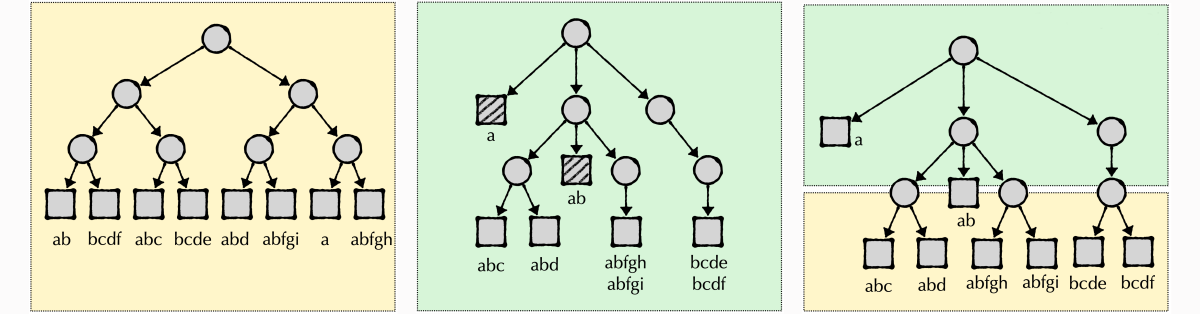
To ensure that the embeddings in the hybrid tree preserve some of the sequential information encoded by tries, we varied the standard TF-IDF approach. First we applied it at the character level, rather than at the word level, so that it measured the relative frequency of particular strings of letters, not just words.
Then we weighted the frequency statistics, overcounting character strings that occurred at the beginning of words, relative to those that occurred later. This forced the embedding to mimic the string extension logic of the tries.
Once we’d adopted this indexing scheme, we found that the PECOS model outperformed both the state-of-the-art linear model and the state-of-the art neural model, when measured by both mean reciprocal rank and the BLEU metric used to evaluate machine translation models.
The use of tries still came with a performance penalty: our model took significantly longer to process inputs than the earlier linear model did. But its execution time was still below the threshold for real-time application and significantly lower than the neural model’s.