George Karypis, a senior principal scientist at Amazon Web Services, is one of the keynote speakers at this year’s Conference on Web Search and Data Mining (WSDM), and his topic will be graph neural networks, his chief area of research at Amazon.
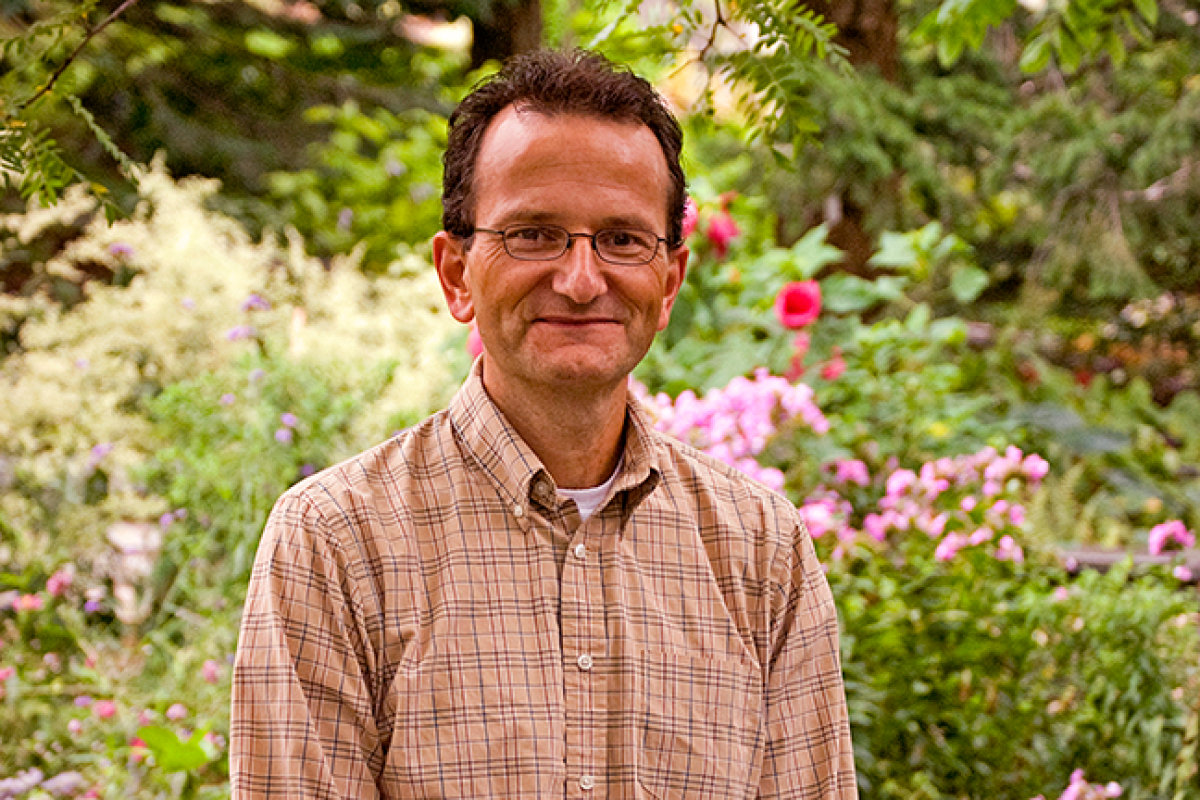
“A lot of the WSDM crowd are looking at relations between entities, especially if you think in terms of the web and social networks,” Karypis says. “If I'm going to develop deep-learning techniques to compute a representation of a graph, then a graph neural network is the right formalism to do that.”
A graph consists of nodes, often depicted as circles, and edges, often depicted as line segments connecting nodes. Graphs are infinitely expressive: the nodes might represent atoms in a molecule and the edges the bonds between them; or, as in a knowledge graph, the nodes could represent entities and the edges relationships between them; or, as in a recommendation engine, the nodes could represent both customers and products, and edges could indicate both similarity between products and which customers have bought which products.
Graph neural networks (GNNs) represent information contained in graphs as vectors, so other machine learning models can make use of that information.
“In the standard machine learning workflow nowadays, we compute a representation of a piece of text,” Karypis says. “I then use that representation as input to a downstream model. I either do an end-to-end fine tuning of my language model or just use it the way it is, as a kind of a static representation.
“We do exactly the same thing for graphs using graph neural networks. For example, in many drug discovery use cases, I can pretrain a graph neural network so that it learns how to compute a representation of small molecules. Then I can take that representation as input to another model that predicts various physicochemical properties of the molecules.”
In addition to providing inputs to downstream models, GNNs can also be used to predict properties of the graphs themselves — deducing missing edges, for instance.
“In that case, you still compute representations of the two nodes that potentially are connected, and then you learn a model that answers the question, ‘Given the representations, are these nodes connected?’” Karypis says. “So you do pretty much the same thing there as well.”
Scope of representation
Graphs are so useful because their structure encodes information beyond the information encoded in individual nodes — the characteristics of particular atoms, products, or customers, for instance. One outstanding research question in the field is how much of that structural information a GNN representation can capture.
Computing node representations is an iterative process. The first step is to compute a representation of each node. The next step is to update each node’s representation, taking into account both its previous representation and the representations of its immediate neighbors. Every repetition of this process extends the scope of the representation by one hop.
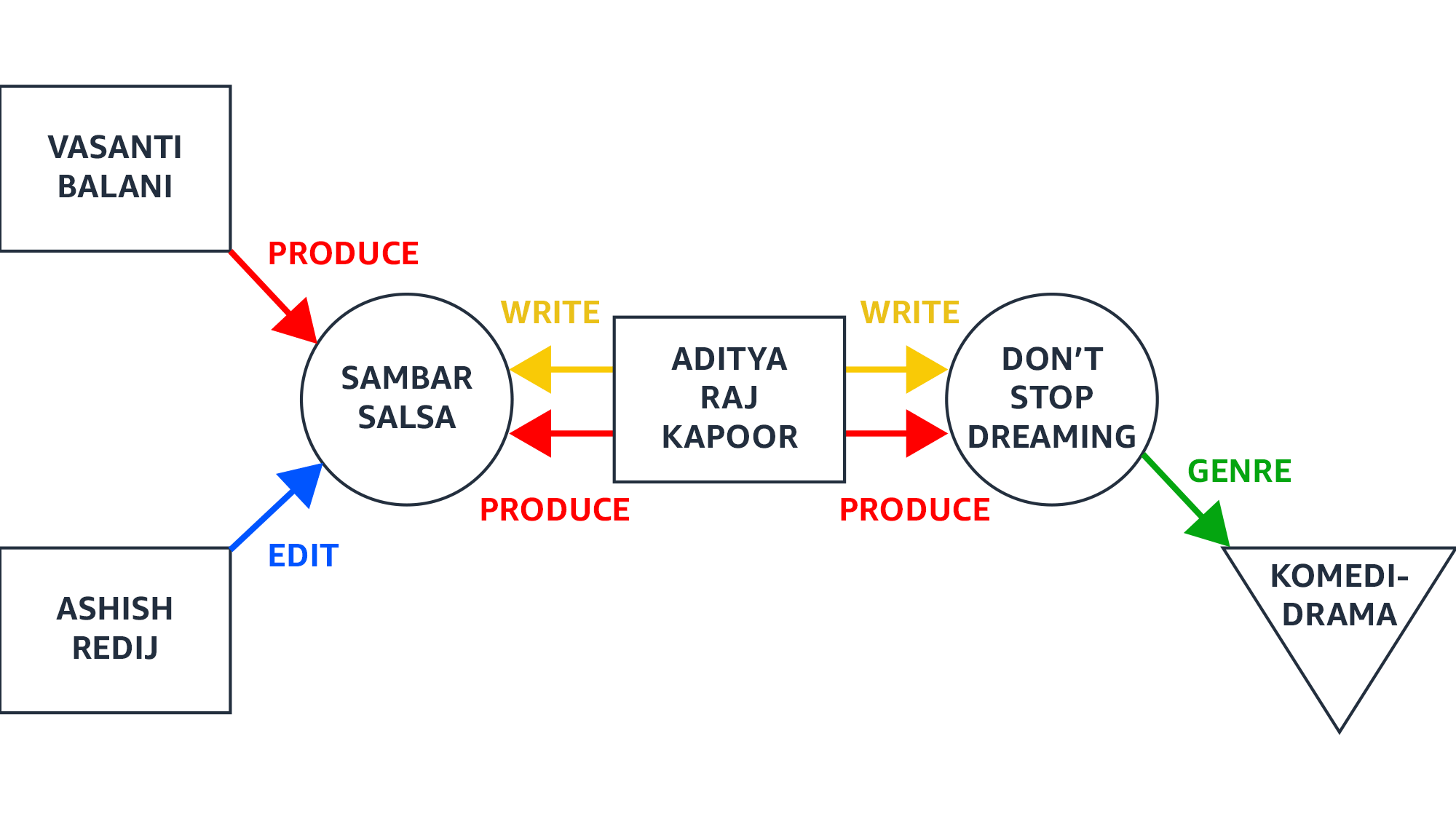
“The problem is that if you keep on doing that, then pretty much every node will end up becoming the same,” Karypis says. “On GNNs we call that oversmoothing. For some networks, like those coming from natural graphs, this often happens after a very small number of steps. Think of social networks and the Kevin Bacon game. It does not take many hops before you hit a large fraction of the nodes.
“In the past year or two, there has been a lot of research work in terms of people trying to see how I can still get information from faraway neighbors but not get to the point that every node becomes pretty much the same because I have oversmoothed all the information?”
Questions of translation
Another outstanding research question, Karypis says, is how to represent data in graph form in the first place, because this has a significant effect on GNN performance.
“There are certain application domains where we've been very successful in developing accurate GNN-based models,” Karypis says. “For example, for domains in which the underlying data is already a graph, such as small and large molecules or knowledge graphs, we have very good GNN models.
“For domains for which there are multiple ways to model the underlying data via a graph, it often takes a lot of trial and error to develop successful GNN-based approaches because we need to consider the interplay between graph and GNN models.
GNN models that can tolerate variations in how the underlying data is modeled will go a long way toward reducing the effort required to develop successful GNN-based approaches.
“If I look at a relational database, let's say I have information about you, like your address. I can choose to create a table for the street name, a table for the zip code, and a table for the city. Then I can create a table for the address. Its rows will have a foreign key to the zip code table, a foreign key to the street name, and a foreign key to the city table. Then, in the table that stores information about you, I can have a foreign key to that address table.
“Alternatively, I can choose to create three different columns in the main table, with street number, city, and zip code. Now If I'm going to view those things as a graph, in one case, everything will be pretty much directly connected. If I have a node for a particular row, that node will be connected to another node that has the street number and street name and so forth. As opposed to the other case, where I'm going to have a pointer to another table that will have the pointers to the other three tables that contain information about the other stuff.
“All of a sudden, something will go from being one hop away to potentially being three hops away or even more. That creates a very different topology when I'm trying to aggregate information within the context of a GNN. Developing GNN models that can tolerate variations on how the underlying data is modeled will go a long way toward reducing the effort required to develop successful GNN-based approaches.
“GNNs are one of the hottest areas of deep-learning research and are being used in an ever-growing set of domains and applications. I think that in the field of GNN research, there are many things that we still do not know. It's a field that is very much in the early days.”