Dr. George Karypis, Distinguished McKnight University Professor at the University of Minnesota Twin Cities and an Amazon Scholar, has been awarded the 10-Year-Highest-Impact award at the 2020 IEEE Conference on Data Mining (ICDM). The award-winning paper was selected by the ICDM award committee, and announced at the conference on November 19, 2020.
Congratulations to alumna Xia Ning & Professor George Karypis for winning the prestigious 10-Year Highest-Impact award at @icdm2020. The paper “SLIM: Sparse Linear Methods for Top-N Recommender Systems” was originally presented at ICDM in 2011.
— UMNComputerScience (@UMNComputerSci) December 7, 2020
➡️ https://t.co/tjM328mV3Q pic.twitter.com/Ah2NBPbsKL
The honor, awarded every year to the authors of an ICDM publication published ten years earlier, recognizes research that has had a significant impact on the field of data mining. Karypis co-authored the paper with Xia Ning, now an associate professor at Ohio State in biomedical informatics and computer science and engineering. Karypis was Ning’s PhD advisor at the time the paper was published.
The paper, SLIM: Sparse Linear Methods for Top-N Recommender Systems, proposes a novel approach for an algorithm that generates high-quality recommendations for e-commerce products at high speeds.
The approach outlined in the paper enables an algorithm to identify a subset of items that an e-commerce customer will most likely find interesting given the user’s interaction history. To recommend an ideal set of products in a computationally scalable way, the paper recommends an entirely novel class of methods that rely on learning a sparse item-to-item similarity matrix (SLIM) from the data. These methods build upon the traditional item-item based neighborhood schemes, but employ statistical learning techniques to estimate the similarity matrix directly from the data.
“Our research that was conducted ten years ago is particularly relevant in 2020, where consumers shop for billions upon billions of products online,” said Karypis. “The results, initially presented in ICDM 2011, showed that SLIM outperforms all previously published methods and does so in a computationally efficient manner. In fact, if you look at 2019’s ACM Recommender Systems Conference Best Paper Awardee, 'Are we Really Making Much Progress? A Worrying Analysis of Recent Neural Recommendation Approaches', you’ll find that the performance achieved by SLIM is extremely competitive to this date.”
In the course of his career, Karypis has conducted research in the areas of data mining, recommender systems, learning analytics, and high-performance computing. Across all of these areas, Karypis has focused on developing novel algorithms and software to solve problems that have practical, real-world applications.
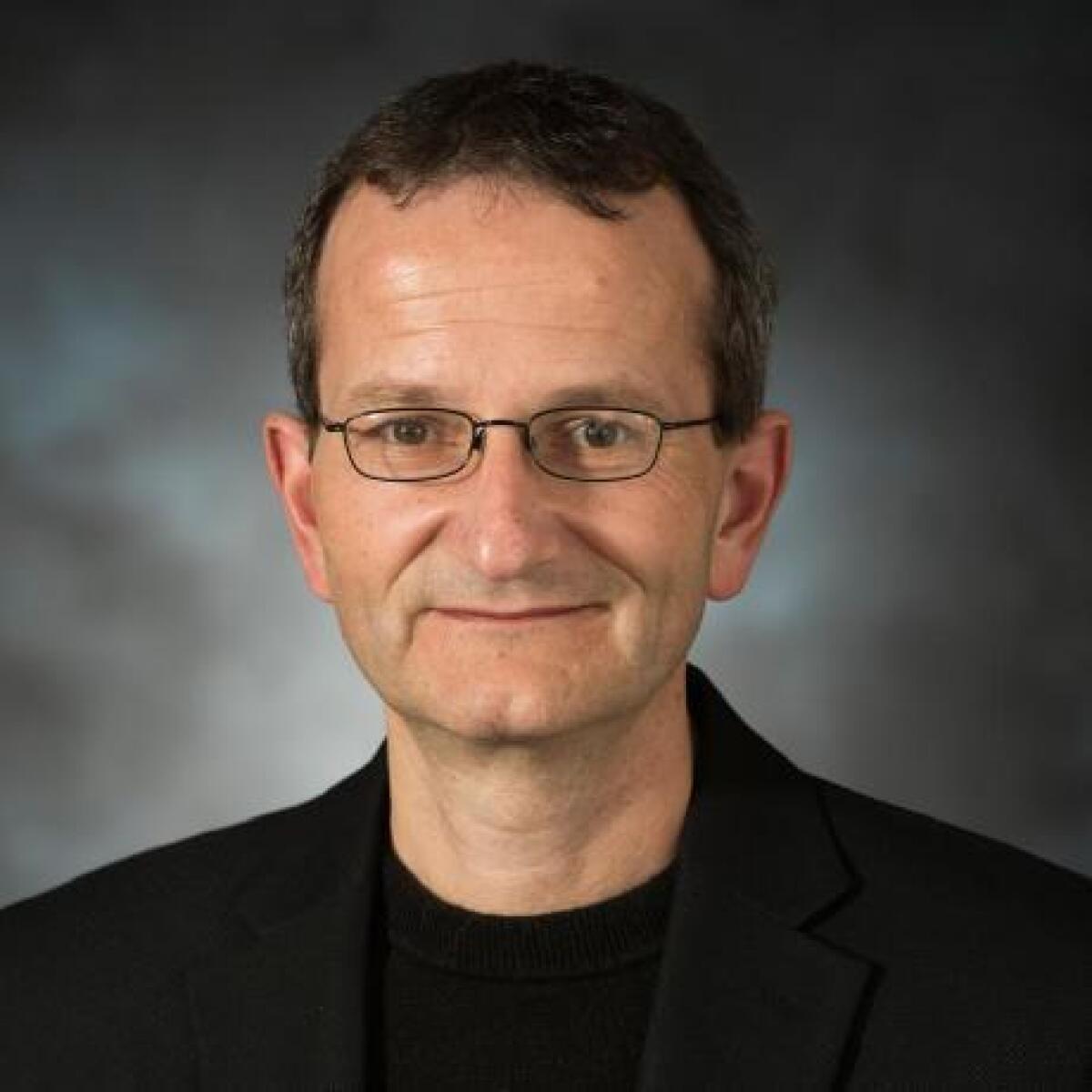
An outcome of Karypis’ research was the development, distribution, and ongoing enhancement of different software tools for graph and hypergraph partitioning on serial and parallel computers. These tools, which make up the METIS Family of Graph and Hypergraph Partitioning Software, are used extensively to partition the graphs and hypergraphs that arise in diverse application areas.
At Amazon, Karypis’ team is working on the Deep Graph Library (DGL), an easy-to-use, high-performance and scalable Python package for deep learning on graphs. DGL is a framework that allows developers to program a class of machine learning models called graph neural networks (GNN). DGL supplements existing tensor-based frameworks such as Tensorflow, PyTorch, and MXNet to support the growing area of deep graph learning.
“I am honored to have worked with collaborators like Xia Ning in the course of my career and I thank the ICDM award committee for recognizing our work,” said Karypis.
To learn more about the role of academics at Amazon, visit the Amazon Scholars page.