This week, the IEEE International Conference on Acoustics, Speech and Signal Processing (ICASSP) got under way in virtual form, to be followed by an in-person meeting two weeks later (May 22-27) in Singapore. ICASSP is the flagship conference of the IEEE Signal Processing Society and, as such, one of the premier venues for publishing the latest advances in automatic speech recognition (ASR) and other speech-processing and speech-related fields, with strong participation from both industry and academia.
More ICASSP coverage on Amazon Science
- Andrew Breen, the senior manager of text-to-speech research in the Amazon Text-to-Speech group, discusses the group's four ICASSP papers.
- The 50-plus Amazon papers at ICASSP, sorted by research topic.
This year, the Alexa AI ASR organization is represented by 21 papers, more than in any prior year, reflecting the growth of speech-related science in Alexa AI. Here we highlight a few of these papers, to give an idea of their breadth.
Multimodal pretraining for end-to-end ASR
Deep-learning methods have taken over as the method of choice in speech-based recognition and classification tasks, and increasingly, self-supervised representation learning is used to pretrain models on large unlabeled datasets, followed by “fine-tuning” on task-labeled data.
In their paper “Multi-modal Pretraining for Automated Speech Recognition”, David Chan and colleagues give a new twist to this approach by pretraining speech representations on audiovisual data. As the self-supervision task for both modalities, they adapt the masked language model, in which words of training sentences are randomly masked out, and the model learns to predict them. In their case, however, the masks are applied to features extracted from the video and audio stream.
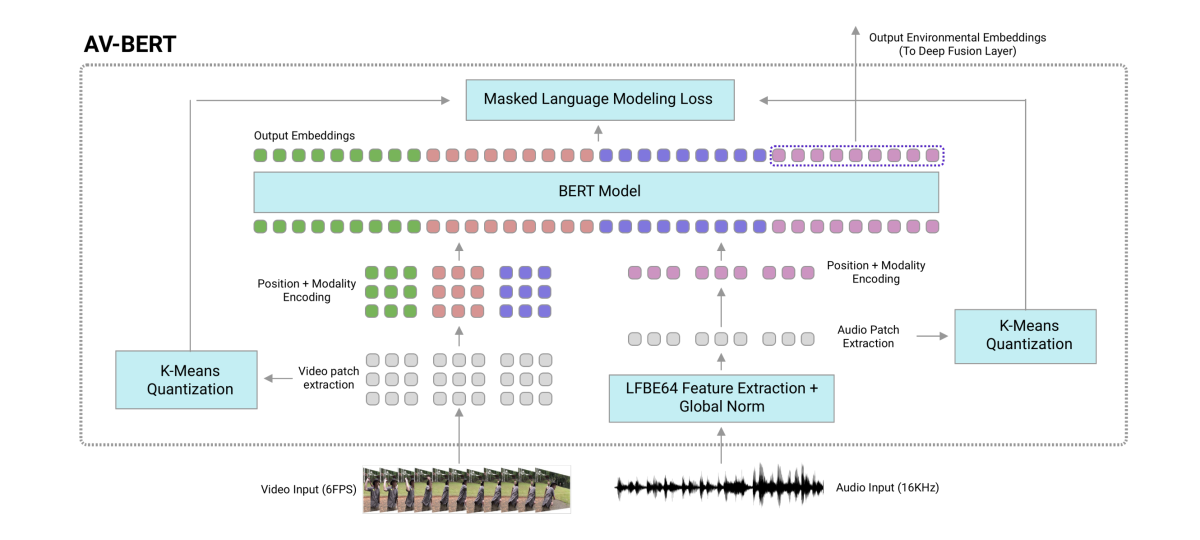
Once pretrained, the audio-only portion of the learned representation is fused with a more standard front-end representation to feed into an end-to-end speech recognition system. The researchers show that this approach yields more accurate ASR results than pretraining with only audio-based self-supervision, suggesting that the correlations between acoustic and visual signals are helpful in extracting higher-level structures relevant to the encoding of speech.
Signal-to-interpretation with multimodal embeddings
The advantages of multimodality are not limited to unsupervised-learning settings. In “Tie your embeddings down: Cross-modal latent spaces for end-to-end spoken language understanding”, Bhuvan Agrawal and coauthors study signal-to-interpretation (S2I) recognizers that map a sequential acoustic input to an embedding, from which the intent of an utterance is directly inferred.
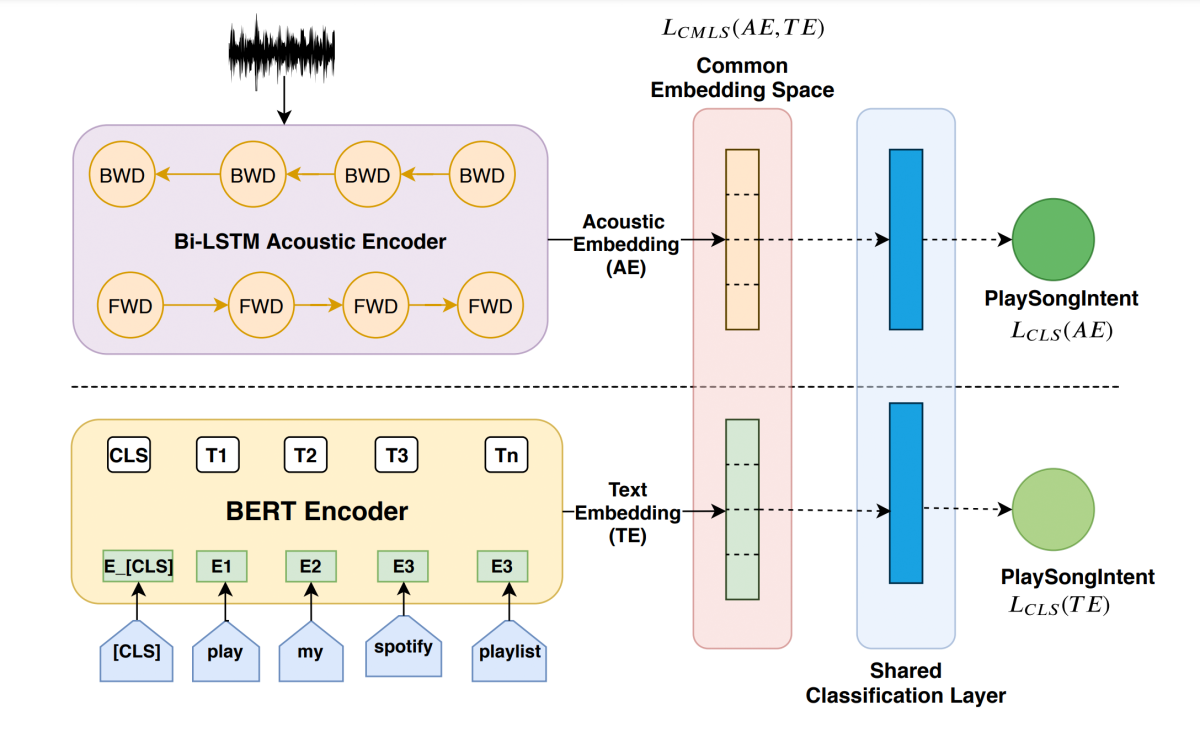
This bypasses the need for explicit speech transcription but still uses supervision for utterance intents. Due to their compactness, S2I models are attractive for on-device deployment, which has multiple benefits. For example, Alexa AI has used on-device speech processing to make Alexa faster and lower-bandwidth.
Agrawal and colleagues show that S2I recognizers give better results when their acoustic embeddings are constrained to be close to embeddings of the corresponding textual input produced by a pretrained language model (BERT). As in the earlier paper, this cross-modal signal is used during learning only and not required for inference (i.e., at runtime). It is a clever way to sneak linguistic structure back into the S2I system while also infusing it with knowledge gleaned from the vastly larger language model training data.
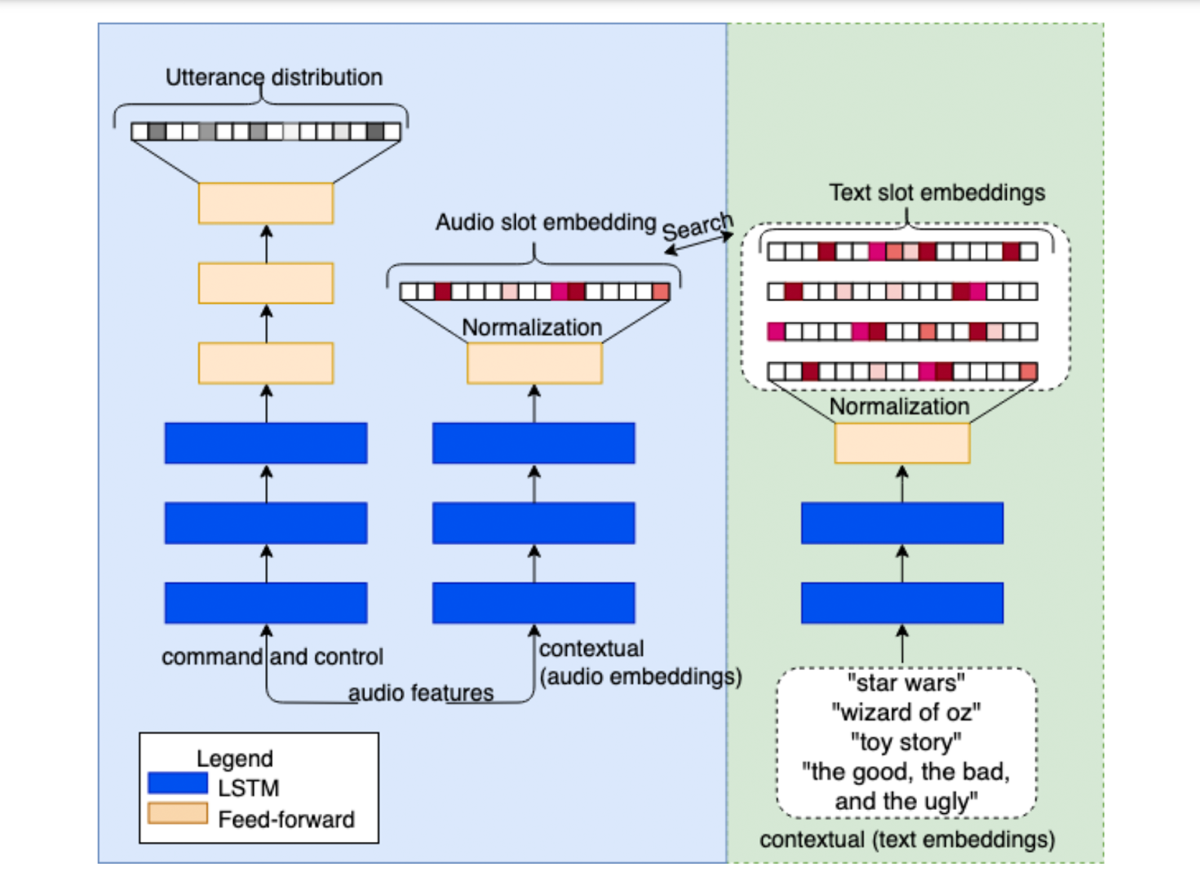
The idea of matching embeddings derived from audio to those for corresponding text strings (i.e., transcripts) also has other applications. In their paper “TinyS2I: A small-footprint utterance classification model with contextual support for on-device SLU”, Anastasios Alexandridis et al. show that extremely compact, low-latency speech-understanding models can be obtained for the utterances most frequently used to control certain applications, such as media playback.
The most frequent control commands (“pause”, “volume up”, and the like) can be classified directly from an acoustic embedding. For commands involving an item from a contextual menu (“play [title]”), the acoustic embedding is matched to the media title’s textual embedding. In this paper, unlike the previous one, the textual embeddings are trained jointly with the acoustic ones. But the same triplet loss function can be used to align the cross-modal embeddings in a shared space.
ASR rescoring with BERT
Deep encoders of text trained using the masked-language-model (MLM) paradigm, such as BERT, have been widely used as the basis for all sorts of natural-language tasks. As mentioned earlier, they can incorporate vast amounts of language data through self-supervised pretraining, followed by task-specific supervised fine-tuning.
So far, however, the practical impact of MLMs on ASR proper has been limited, in part because of unsatisfactory tradeoffs between computational overhead (latency) and achievable accuracy gains. This is now changing with the work of Liyan Xu et al., as described in “RescoreBERT: Discriminative speech recognition rescoring with BERT”.
The researchers show how BERT-generated sentence encodings can be incorporated into a model that rescores the text strings output by an ASR model. Because BERT is trained on large corpora of (text-only) public data, it understands the relative probabilities of different ASR hypotheses better than the ASR model can.
The researchers achieved their best results with a combined loss function that is based on both sentence pseudo-likelihood — a more computationally tractable estimate of sentence likelihood — and word error prediction. The resulting rescoring model is so effective compared to standard LSTM (long short-term memory) language models, while also exhibiting lower latency, that the RescoreBERT method has gone from internship project to Alexa production in less than a year.
Ontological biasing for acoustic-event detection
We round out this short selection of papers with one from an ASR-adjacent field. In “Improved representation learning for acoustic event classification using tree-structured ontology”, Arman Zharmagambetov and coauthors look at an alternative to self-supervised training for the task of acoustic-event detection (AED). (AED is the technology behind Alexa’s ability to detect breaking glass, smoke alarms, and other noteworthy events around the house.)
They show that AED classifier training can be enhanced by forcing the resulting representations to identify not only the target event label (such as “dog barking”) but also supercategories (such as “domestic animal” and “animal sound”) drawn from an ontology, a hierarchical representation of relationships between concepts. The method can be further enhanced by forcing the classification to stay the same under distortions of the inputs. The researchers found that their method is more effective than purely self-supervised pretraining and comes close to fully supervised training with only a fraction of the labeled data.
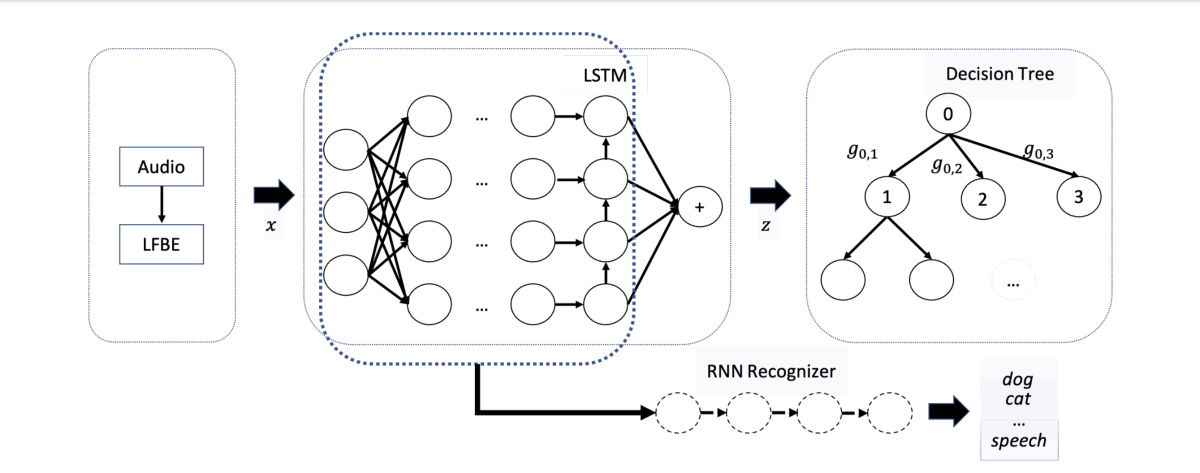
Conclusion and outlook
As we have seen, Alexa relies on a range of audio-based technologies that use deep-learning architectures. The need to train these models robustly, fairly, and with limited supervision, as well as computational constraints at runtime, continues to drive research in Alexa Science. We have highlighted some of the results from that work as they are about to be presented to the wider science community, and we are excited to see the field as a whole come up with creative solutions and push toward ever more capable applications of speech-based AI.