The automatic conversion of text to speech is crucial to Alexa: it’s how Alexa communicates with customers. The models developed by the Amazon Text-to-Speech group are also available to Amazon Web Services (AWS) customers through Polly, the AWS text-to-speech service.
The Text-to-Speech (TTS) group has four papers at this year’s International Conference on Acoustics, Speech, and Signal Processing (ICASSP), all of which deal with either voice conversion (preserving prosodic features while converting one synthetic voice to another), data augmentation, or both.
More ICASSP coverage on Amazon Science
- Alexa AI senior principal scientist Andreas Stolcke highlights five of the 21 ICASSP papers from Alexa's automatic-speech-recognition team.
- The 50-plus Amazon papers at ICASSP, sorted by research topic.
In “Voice Filter: Few-shot text-to-speech speaker adaptation using voice conversion as a post-processing module”, the Amazon TTS group addresses the problem of few-shot speaker adaptation, or learning a new synthetic voice from just a handful of training examples. The paper reformulates the problem as learning a voice conversion model that’s applied to the output of a high-quality TTS model, a conceptual shift from the existing few-shot-TTS paradigm.
In “Cross-speaker style transfer for text-to-speech using data augmentation”, the team shows how to build a TTS model capable of expressive speech, even when the only available training data for the target voice consists of neutral speech. The idea is to first train a voice conversion model, which converts samples of expressive speech in other voices into the target voice, and then use the converted speech as additional training data for the TTS model.
In “Distribution augmentation for low-resource expressive text-to-speech”, the TTS group expands the range of texts used to train a TTS model by recombining excerpts from existing examples to produce new examples. The trick is to maintain the syntactic coherence of the synthetic examples, so that the TTS model won’t waste resources learning improbable sequences of phonemes. (This is the one data augmentation paper that doesn’t rely on voice conversion.)
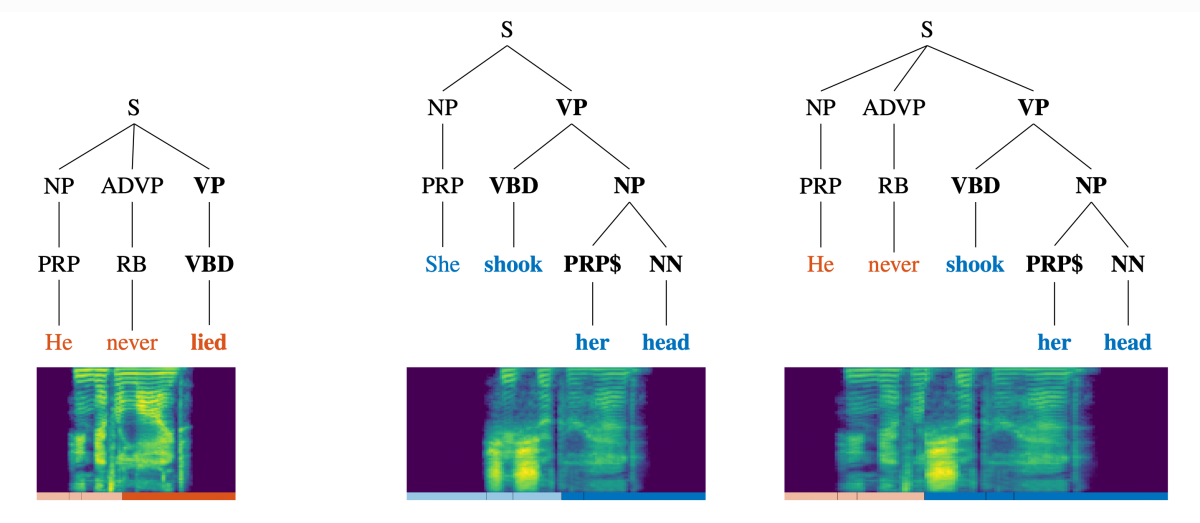
Finally, in “Text-free non-parallel many-to-many voice conversion using normalising flows”, the team adapts the concept of normalizing flows, which have been used widely for TTS, to the problem of voice conversion. Like most deep-learning models, normalizing flows learn functions that produce vector representations of input data. The difference is that the functions are invertible, so the inputs can be recovered from the representations. The team hypothesized that preserving more information from the input data would yield better voice conversion, and early experiments bear that hypothesis out.
Voice filter
The idea behind “Voice Filter: Few-shot text-to-speech speaker adaptation using voice conversion as a post-processing module” is that for few-shot learning, it’s easier to take the output of an existing, high-quality TTS model — a voice spectrogram — and adapt that to a new target voice than it is to adapt the model itself.
The key to the approach is that the voice filter, which converts the TTS model’s output to a new voice, is trained on synthetic data created by the TTS model itself.
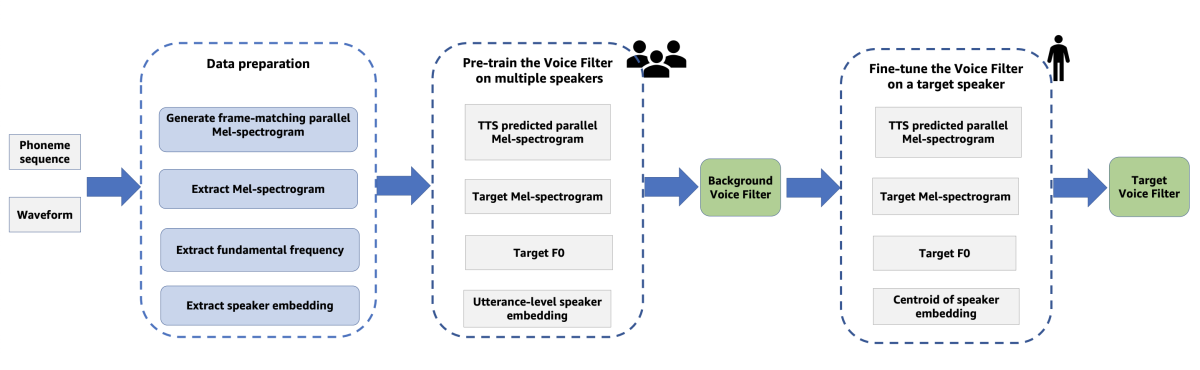
The TTS model is duration controllable, meaning that the input text is encoded to indicate the duration that each phoneme should have in the output speech. This enables the researchers to create two parallel corpora of training data. One corpus consists of real training examples, from 120 different speakers. The other corpus is synthetic speech generated by the TTS model, but with durations that match those of the multispeaker examples.
The voice filter is trained on the parallel corpora, and then, for few-shot learning, the researchers simply fine-tune it on a new speaker. In experiments, the researchers found that this approach produced speech whose quality was comparable to that produced by conventional models trained on 30 times as much data.
Cross-speaker style transfer
The voice conversion model that the researchers use in “Cross-speaker style transfer for text-to-speech using data augmentation” is based on the CopyCat model previously reported on the Amazon Science blog. The converted expressive data is added to the neutral data to produce the dataset used to train the TTS model
The TTS model takes two inputs: a text sequence and a style vector. During training, the text sequence passes to the TTS model, and the spectrogram of the target speech sample passes to a reference encoder, which produces the style embedding. At inference time, of course, there is no input spectrogram. But the researchers show that they can control the style of the TTS model’s output by feeding it a precomputed style embedding.
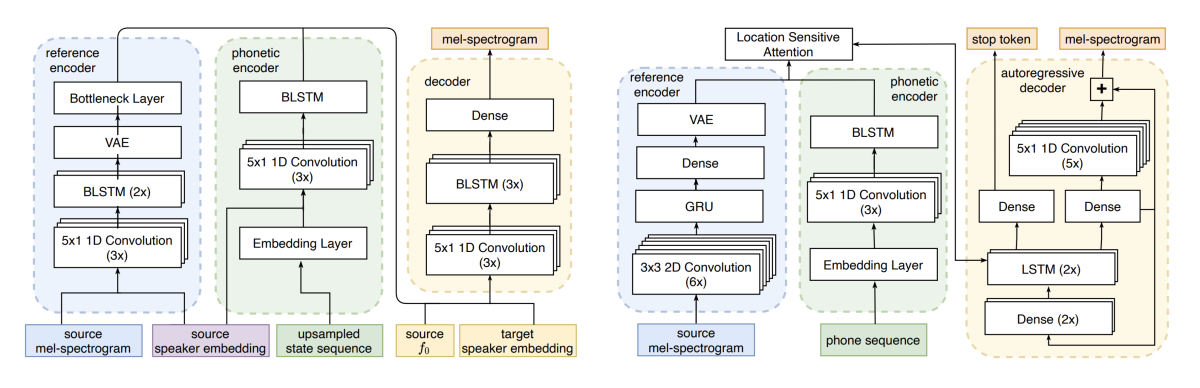
The researchers assessed the model based on human evaluation using the MUSHRA perception scale. Human evaluators reported that, relative to a benchmark model, the new model reduced the gap in perceived style similarity between synthesized and real speech by an average of 58% across 14 different speakers.
Distribution augmentation
“Distribution augmentation for low-resource expressive text-to-speech” considers the case in which training data for a new voice is lacking. The goal is to permute the texts of the existing examples, producing new examples, and recombine excerpts from the corresponding speech samples to produce new samples. This does not increase the acoustic diversity of the training targets, but it does increase the linguistic diversity of the training inputs.
To ensure that the synthetic training examples do not become too syntactically incoherent, the researchers construct parse trees for the input texts and then swap syntactically equivalent branches across trees (see figure, above). Swapping the corresponding sections of the acoustic signal requires good alignment between text and signal, which is accomplished by existing forced-alignment models.
During training, to ensure that the resulting TTS model doesn’t become overbiased toward the synthetic examples, the researchers also include a special input token to indicate points at which two existing samples have been fused together. The expectation is that the model will learn to privilege phonemic sequences internal to the real samples over phonemic sequences that cross boundaries between fused samples. At inference time, the value of the token is simply set to 0 across all inputs.
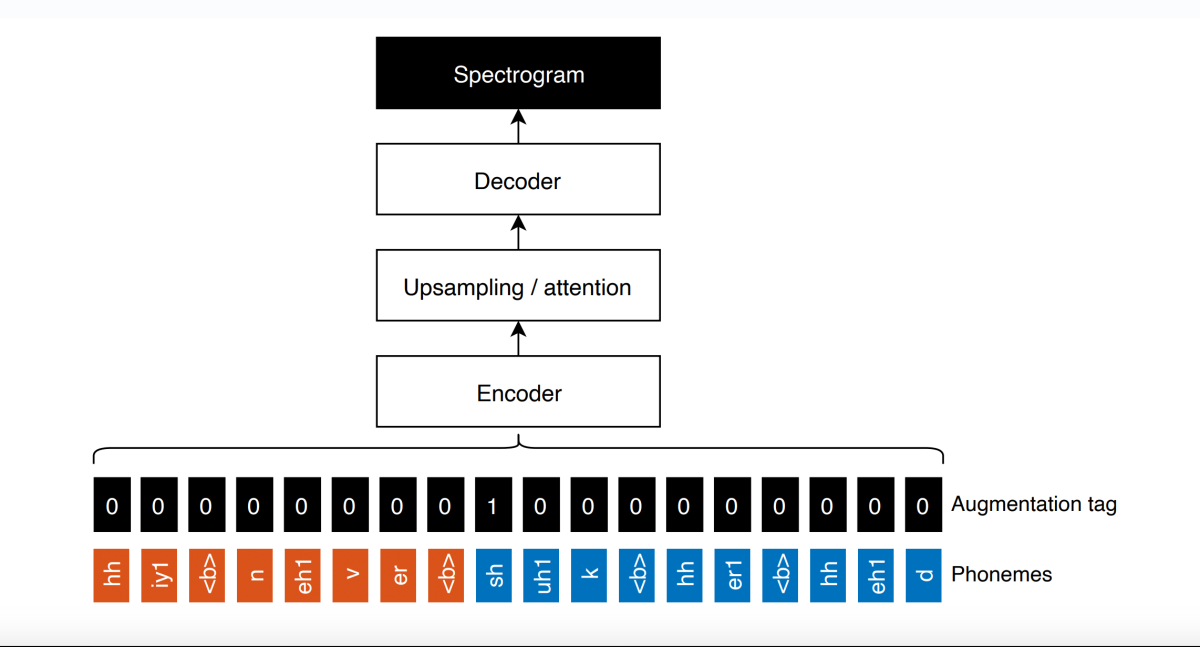
The quality of the model’s speech output was assessed by 60 human evaluators, who compared it to speech output by a baseline model, on five different datasets. Across the board, the output of the new model received better scores than the output of the benchmark model.
Normalizing flows
A normalizing flow learns to map input data to a representational space in a way that maximizes the approximation of some prior distribution. The word “flow” indicates that the mapping can be the result of passing the data through a series of invertible transformations, and the enforcement of the distribution imposes the normalization.
In “Text-free non-parallel many-to-many voice conversion using normalising flows”, Amazon TTS researchers consider a flow whose inputs are a source spectrogram, a phoneme embedding, a speaker identity embedding, the fundamental frequency of the acoustic signal, and a flag denoting whether a frame of input audio is voiced or unvoiced. The flow maps the inputs to a distribution of phoneme frequencies in a particular application domain.
Typically, a normalizing flow will learn both the distribution and the mapping from the training data. But here, the researchers pretrain the flow on a standard TTS task, for which training data is plentiful, to learn the distribution in advance.
Because the flow is reversible, a vector in the representational space can be mapped back to a set of source inputs, provided that the other model inputs (phoneme embedding, speaker ID, and so on) are available. To use normalizing flows to perform speech conversion, the researchers simply substitute one speaker for another during this reverse mapping.
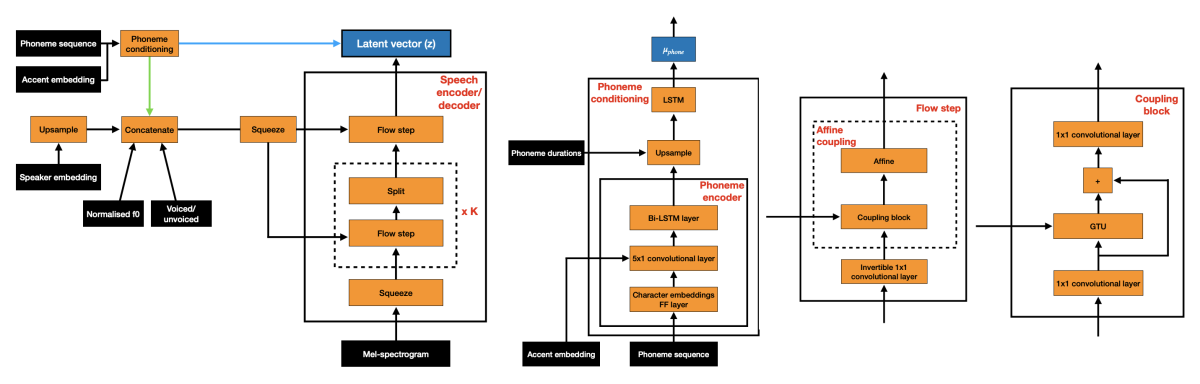
The researchers examine two different experimental setting, one in which the voice conversion model takes both text sequences and spectrograms as inputs and one in which it takes spectrograms only. In the second case, the pretrained normalizing-flow model significantly outperformed the benchmarks. A normalizing-flow model that learned the phoneme distribution directly from the training data didn’t fare as well, indicating the importance of the pretraining step.