Amazon researchers have more than 50 papers at this year’s International Conference on Acoustics, Speech, and Signal Processing (ICASSP). A plurality of them are on automatic speech recognition and related topics, such as keyword spotting and speaker identification. But others range farther afield, to topics such as computer vision and federated learning.
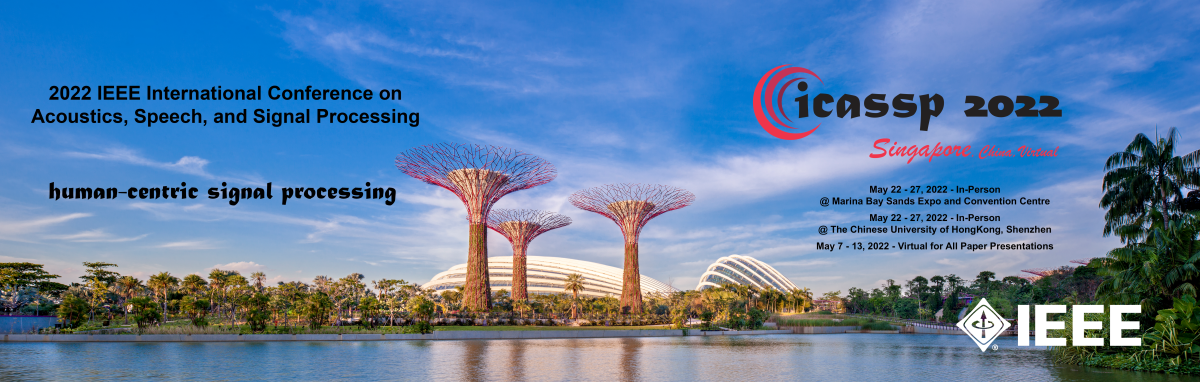
Acoustic-event detection
Federated self-supervised learning for acoustic event classification
Meng Feng, Chieh-Chi Kao, Qingming Tang, Ming Sun, Viktor Rozgic, Spyros Matsoukas, Chao Wang
Improved representation learning for acoustic event classification using tree-structured ontology
Arman Zharmagambetov, Qingming Tang, Chieh-Chi Kao, Qin Zhang, Ming Sun, Viktor Rozgic, Jasha Droppo, Chao Wang
WikiTAG: Wikipedia-based knowledge embeddings towards improved acoustic event classification
Qin Zhang, Qingming Tang, Chieh-Chi Kao, Ming Sun, Yang Liu, Chao Wang
Automatic speech recognition
A likelihood ratio-based domain adaptation method for end-to-end models
Chhavi Choudhury, Ankur Gandhe, Xiaohan Ding, Ivan Bulyko
Being greedy does not hurt: Sampling strategies for end-to-end speech recognition
Jahn Heymann, Egor Lakomkin, Leif RādellJahn Heymann, Egor Lakomkin, Leif RādelJahn Heymann, Egor Lakomkin, Leif RādelJahn Heymann, Egor Lakomkin, Leif Rādel
Caching networks: Capitalizing on common speech for ASR
Anastasios Alexandridis, Grant P. Strimel, Ariya Rastrow, Pavel Kveton, Jon Webb, Maurizio Omologo, Siegfried Kunzmann, Athanasios Mouchtaris
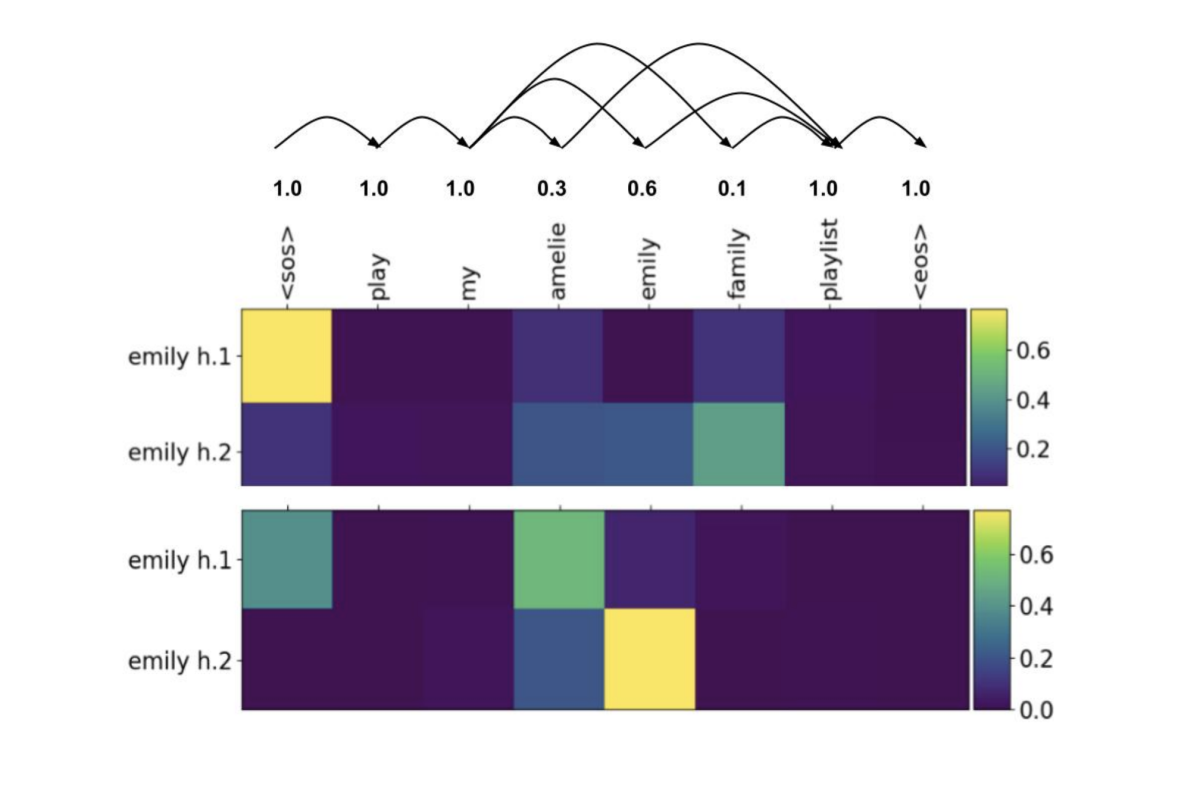
Contextual adapters for personalized speech recognition in neural transducers
Kanthashree Mysore Sathyendra, Thejaswi Muniyappa, Feng-Ju Chang, Jing Liu, Jinru Su, Grant P. Strimel, Athanasios Mouchtaris, Siegfried Kunzmann
LATTENTION: Lattice attention in ASR rescoring
Prabhat Pandey, Sergio Duarte Torres, Ali Orkan Bayer, Ankur Gandhe, Volker Leutnant
Listen, know and spell: Knowledge-infused subword modeling for improving ASR performance of out-of-vocabulary (OOV) named entities
Nilaksh Das, Monica Sunkara, Dhanush Bekal, Duen Horng Chau, Sravan Bodapati, Katrin Kirchhoff
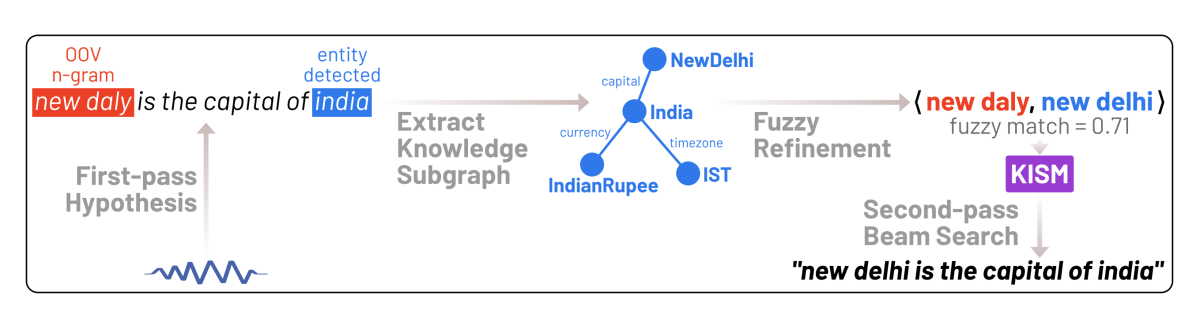
Mitigating closed-model adversarial examples with Bayesian neural modeling for enhanced end-to-end speech recognition
Chao-Han Huck Yang, Zeeshan Ahmed, Yile Gu, Joseph Szurley, Roger Ren, Linda Liu, Andreas Stolcke, Ivan Bulyko
Multi-modal pre-training for automated speech recognition
David M. Chan, Shalini Ghosh, Debmalya Chakrabarty, Björn Hoffmeister
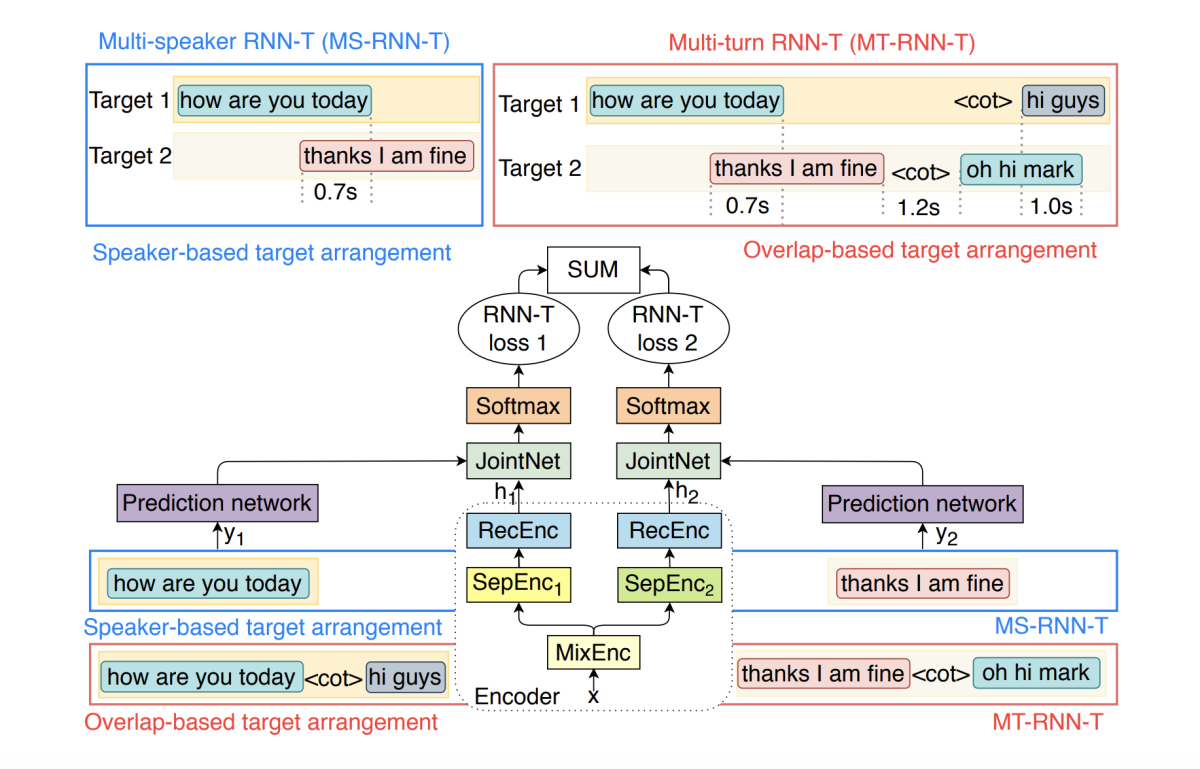
Multi-turn RNN-T for streaming recognition of multi-party speech
Ilya Sklyar, Anna Piunova, Xianrui Zheng, Yulan Liu
RescoreBERT: Discriminative speech recognition rescoring with BERT
Liyan Xu, Yile Gu, Jari Kolehmainen, Haidar Khan, Ankur Gandhe, Ariya Rastrow, Andreas Stolcke, Ivan Bulyko
USTED: Improving ASR with a unified speech and text encoder-decoder
Bolaji Yusuf, Ankur Gandhe, Alex Sokolov
VADOI: Voice-activity-detection overlapping inference for end-to-end long-form speech recognition
Jinhan Wang, Xiaosu Tong, Jinxi Guo, Di He, Roland Maas
Computer vision
ASD-transformer: Efficient active speaker detection using self and multimodal transformers
Gourav Datta, Tyler Etchart, Vivek Yadav, Varsha Hedau, Pradeep Natarajan, Shih-Fu Chang
Dynamically pruning SegFormer for efficient semantic segmentation
Haoli Bai, Hongda Mao, Dinesh Nair
Enhancing contrastive learning with temporal cognizance for audio-visual representation generation
Chandrashekhar Lavania, Shiva Sundaram, Sundararajan Srinivasan, Katrin Kirchhoff
Few-shot gaze estimation with model offset predictors
Jiawei Ma, Xu Zhang, Yue Wu, Varsha Hedau, Shih-Fu Chang
Visual representation learning with self-supervised attention for low-label high-data regime
Prarthana Bhattacharyya, Chenge Li, Xiaonan Zhao, István Fehérvári, Jason Sun
Federated learning
Federated learning challenges and opportunities: An outlook
Jie Ding, Eric Tramel, Anit Kumar Sahu, Shuang Wu, Salman Avestimehr, Tao Zhang
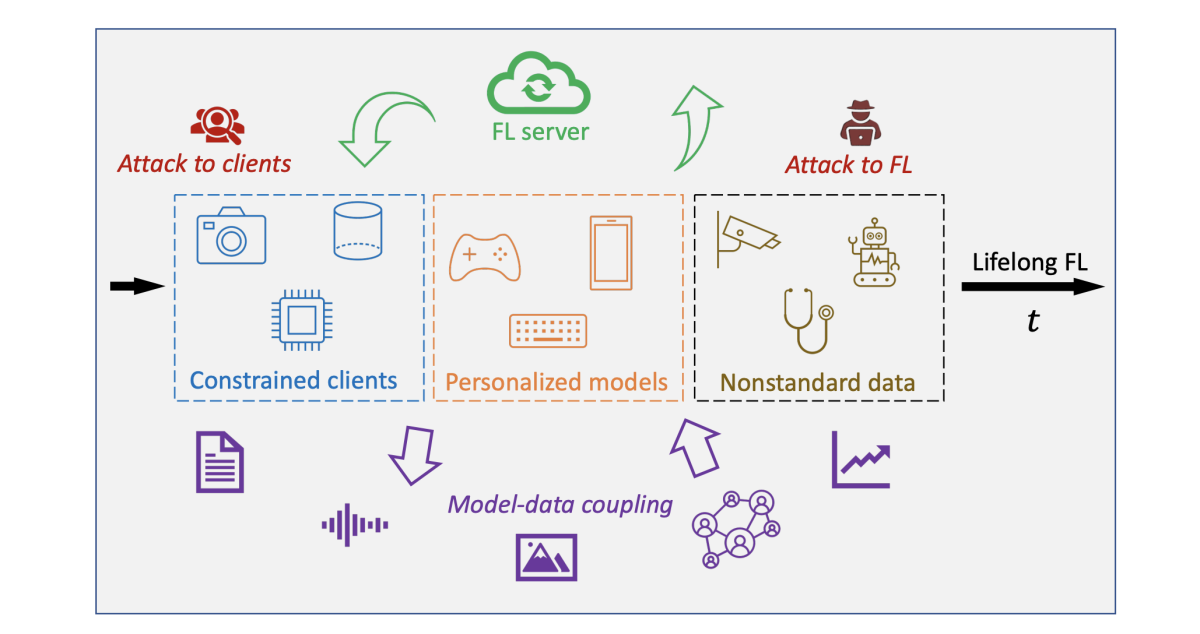
Learnings from federated learning in the real world
Christophe Dupuy, Tanya G. Roosta, Leo Long, Clement Chung, Rahul Gupta, Salman Avestimehr
Information retrieval
Contrastive knowledge graph attention network for request-based recipe recommendation
Xiyao Ma, Zheng Gao, Qian Hu, Mohamed Abdelhady
Keyword spotting
Unified speculation, detection, and verification keyword spotting
Geng-shen Fu, Thibaud Senechal, Aaron Challenner, Tao Zhang
Machine translation
Isometric MT: Neural machine translation for automatic dubbing
Surafel Melaku Lakew, Yogesh Virkar, Prashant Mathur, Marcello Federico
Natural-language understanding
ADVIN: Automatically discovering novel domains and intents from user text utterances
Nikhita Vedula, Rahul Gupta, Aman Alok, Mukund Sridhar, Shankar Ananthakrishnan
An efficient DP-SGD mechanism for large scale NLU models
Christophe Dupuy, Radhika Arava, Rahul Gupta, Anna Rumshisky
Paralinguistics
Confidence estimation for speech emotion recognition based on the relationship between emotion categories and primitives
Yang Li, Constantinos Papayiannis, Viktor Rozgic, Elizabeth Shriberg, Chao Wang
Multi-lingual multi-task speech emotion recognition using wav2vec 2.0
Mayank Sharma
Representation learning through cross-modal conditional teacher-student training for speech emotion recognition
Sundararajan Srinivasan, Zhaocheng Huang, Katrin Kirchhoff
Sentiment-aware automatic speech recognition pre-training for enhanced speech emotion recognition
Ayoub Ghriss, Bo Yang, Viktor Rozgic, Elizabeth Shriberg, Chao Wang
Personalization
Incremental user embedding modeling for personalized text classification
Ruixue Lian, Che-Wei Huang, Yuqing Tang, Qilong Gu, Chengyuan Ma, Chenlei (Edward) Guo
Signal processing
Deep adaptive AEC: Hybrid of deep learning and adaptive acoustic echo cancellation
Hao Zhang, Srivatsan Kandadai, Harsha Rao, Minje Kim, Tarun Pruthi, Trausti Kristjansson
Improved singing voice separation with chromagram-based pitch-aware remixing
Siyuan Yuan, Zhepei Wang, Umut Isik, Ritwik Giri, Jean-Marc Valin, Michael M. Goodwin, Arvindh Krishnaswamy
Sparse recovery of acoustic waves
Mohamed Mansour
Upmixing via style transfer: A variational autoencoder for disentangling spatial images and musical content
Haici Yang, Sanna Wager, Spencer Russell, Mike Luo, Minje Kim, Wontak Kim
Sound source localization
End-to-end Alexa device arbitration
Jarred Barber, Yifeng Fan, Tao Zhang
Speaker diarization/identification/verification
ASR-aware end-to-end neural diarization
Aparna Khare, Eunjung Han, Yuguang Yang, Andreas Stolcke
Improving fairness in speaker verification via group-adapted fusion network
Hua Shen, Yuguang Yang, Guoli Sun, Ryan Langman, Eunjung Han, Jasha Droppo, Andreas Stolcke
OpenFEAT: Improving speaker identification by open-set few-shot embedding adaptation with Transformer
Kishan K C, Zhenning Tan, Long Chen, Minho Jin, Eunjung Han, Andreas Stolcke, Chul Lee
Self-supervised speaker recognition training using human-machine dialogues
Metehan Cekic, Ruirui Li, Zeya Chen, Yuguang Yang, Andreas Stolcke, Upamanyu Madhow
Self-supervised speaker verification with simple Siamese network and self-supervised regularization
Mufan Sang, Haoqi Li, Fang Liu, Andrew O. Arnold, Li Wan
Spoken-language understanding
A neural prosody encoder for end-to-end dialogue act classification
Kai Wei, Dillon Knox, Martin Radfar, Thanh Tran, Markus Mueller, Grant P. Strimel, Nathan Susanj, Athanasios Mouchtaris, Maurizio Omologo
Multi-task RNN-T with semantic decoder for streamable spoken language understanding
Xuandi Fu, Feng-Ju Chang, Martin Radfar, Kai Wei, Jing Liu, Grant P. Strimel, Kanthashree Mysore Sathyendra
Tie your embeddings down: Cross-modal latent spaces for end-to-end spoken language understanding
Bhuvan Agrawal, Markus Mueller, Samridhi Choudhary, Martin Radfar, Athanasios Mouchtaris, Ross McGowan, Nathan Susanj, Siegfried Kunzmann
TINYS2I: A small-footprint utterance classification model with contextual support for on-device SLU
Anastasios Alexandridis, Kanthashree Mysore Sathyendra, Grant P. Strimel, Pavel Kveton, Jon Webb, Athanasios Mouchtaris
Text-to-speech
Cross-speaker style transfer for text-to-speech using data augmentation
Manuel Sam Ribeiro, Julian Roth, Giulia Comini, Goeric Huybrechts, Adam Gabrys, Jaime Lorenzo-Trueba
Distribution augmentation for low-resource expressive text-to-speech
Mateusz Lajszczak, Animesh Prasad, Arent van Korlaar, Bajibabu Bollepalli, Antonio Bonafonte, Arnaud Joly, Marco Nicolis, Alexis Moinet, Thomas Drugman, Trevor Wood, Elena Sokolova
Duration modeling of neural TTS for automatic dubbing
Johanes Effendi, Yogesh Virkar, Roberto Barra-Chicote, Marcello Federico
Neural speech synthesis on a shoestring: Improving the efficiency of LPCNET
Jean-Marc Valin, Umut Isik, Paris Smaragdis, Arvindh Krishnaswamy
Text-free non-parallel many-to-many voice conversion using normalising flows
Thomas Merritt, Abdelhamid Ezzerg, Piotr Biliński, Magdalena Proszewska, Kamil Pokora, Roberto Barra-Chicote, Daniel Korzekwa
VoiceFilter: Few-shot text-to-speech speaker adaptation using voice conversion as a post-processing module
Adam Gabrys, Goeric Huybrechts, Manuel Sam Ribeiro, Chung-Ming Chien, Julian Roth, Giulia Comini, Roberto Barra-Chicote, Bartek Perz, Jaime Lorenzo-Trueba
Time series forecasting
Robust nonparametric distribution forecast with backtest-based bootstrap and adaptive residual selection
Longshaokan Marshall Wang, Lingda Wang, Mina Georgieva, Paulo Machado, Abinaya Ulagappa, Safwan Ahmed, Yan Lu, Arjun Bakshi, Farhad Ghassemi