Typically, when someone speaks to a voice agent like Alexa, an automatic speech recognition (ASR) model converts the speech to text. A natural-language-understanding (NLU) model then interprets the text, giving the agent structured data that it can act on.
Traditionally, ASR systems were pipelined, with separate acoustic models, dictionaries, and language models. The language models encoded word sequence probabilities, which could be used to decide between competing interpretations of the acoustic signal. Because their training data included public texts, the language models encoded probabilities for a large variety of words.
End-to-end ASR models, which take an acoustic signal as input and output word sequences, are far more compact, and overall, they perform as well as the older, pipelined systems did. But they are typically trained on limited data consisting of audio-and-text pairs, so they sometimes struggle with rare words.
The standard way to address this problem is to use a separate language model to rescore the output of the end-to-end model. If the end-to-end model is running on-device, for instance, the language model might rescore its output in the cloud.
At this year’s Automatic Speech Recognition and Understanding Workshop (ASRU), we presented a paper in which we propose training the rescoring model not only on the standard language model objective — computing word sequence probabilities — but also on tasks performed by the NLU model.
The idea is that adding NLU tasks, for which labeled training data are generally available, can help the language model ingest more knowledge, which will aid in the recognition of rare words. In experiments, we found that this approach could reduce the language model’s error rate on rare words by about 3% relative to a rescoring language model trained in the conventional way and by about 5% relative to a model with no rescoring at all.
Furthermore, we got our best results by pretraining the rescoring model on just the language model objective and then fine-tuning it on the combined objective using a smaller NLU dataset. This allows us to leverage large amounts of unannotated data while still getting the benefit of the multitask learning.
Multitask training
Our end-to-end ASR model is a recurrent neural network–transducer, a type of network that processes sequential inputs in order. Its output is a set of text hypotheses, ranked according to probability.
Ordinarily, an NLU model performs two principal functions: intent classification and slot tagging. If the customer says, for instance, “Play ‘Christmas’ by Darlene Love”, the intent might be PlayMusic, and the slots SongName and ArtistName would take the values “Christmas” and “Darlene Love”, respectively.
Language models are usually trained on the task of predicting the next word in a sequence, given the words that precede it. The model learns to represent the input words as fixed-length vectors — embeddings — that capture the information necessary to do accurate prediction.
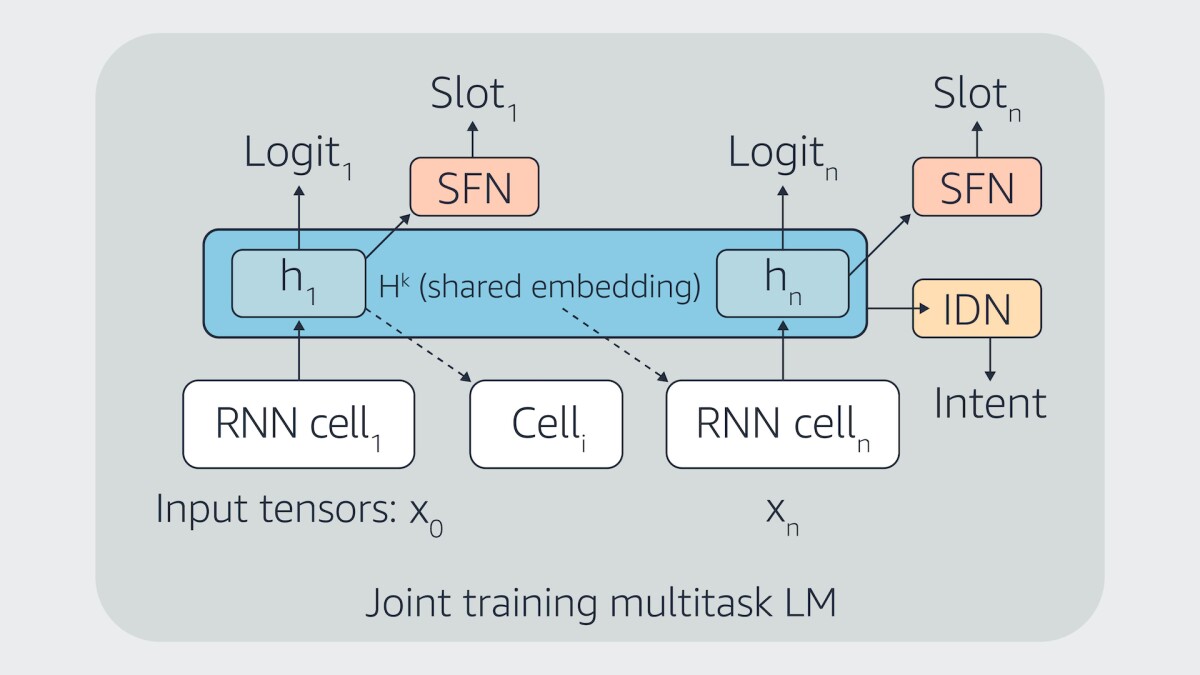
We feed the language model embeddings to two additional subnetworks, an intent detection network and a slot-filling network. During training, the model learns to produce embeddings optimized for all three tasks — word prediction, intent detection, and slot filling.
At run time, the additional subnetworks for intent detection and slot filling are not used. The rescoring of the ASR model’s text hypotheses is based on the sentence probability scores computed from the word prediction task (“LM scores” in the figure below).
During training, we had to optimize three objectives simultaneously, and that meant assigning each objective a weight, indicating how much to emphasize it relative to the others.
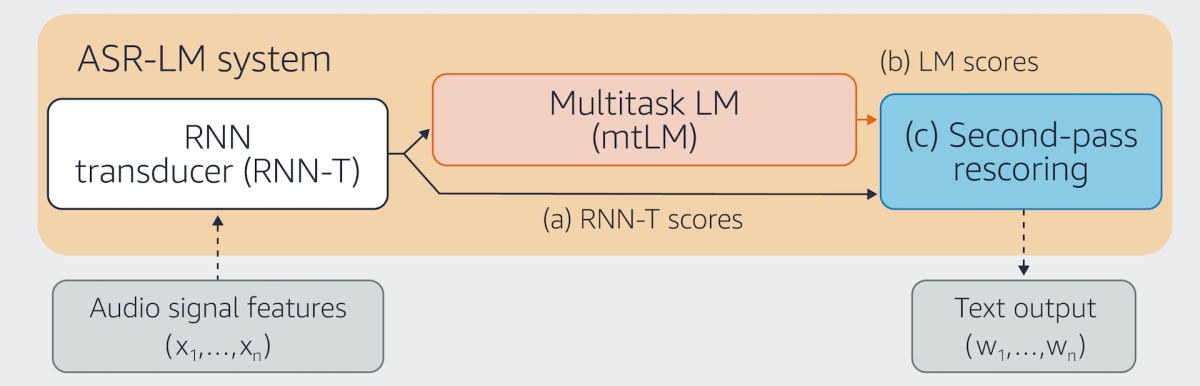
We experimented with two techniques for assigning weights. One was a linear method, in which we started the weights of the NLU objectives at zero and incrementally dialed them up. The other was the randomized-weight-majority algorithm, in which each objective’s weight is randomly assigned according to a particular probability distribution. The distributions are adjusted during training, depending on performance. In our experiments, this worked better than the linear method.
The gains our method shows — a 2.6% reduction in word error rate for rare words, relative to a rescoring model built atop an ordinary language model — are not huge, but they do demonstrate the merit of our approach. In ongoing work, we are exploring additional methods to drive the error rate down further.
For instance, we could use the NLU classifications as explicit inputs to the decoder, rather than just as objectives for training the encoder. Or we could use the intent classification to dynamically bias the rescoring results. We are also exploring semi-supervised training techniques, in which we augment the labeled data used to train the NLU subnetworks with larger corpora of automatically labeled data.