For as long as he can remember, Jonathan Toner has been drawn to the toughest problems he can find. Where did life originate? What can Earth’s most extreme environments tell us about the planet’s history? Was Mars always the desolate, lifeless place we imagined it to be?
Toner investigated these and other questions as a research assistant professor of Earth and space sciences at the University of Washington (UW), where he also earned a PhD in geophysics. After more than a decade in academia, he joined the Amazon Flex team as a research scientist in 2020. It was an eyebrow-raising move: The two careers have very little overlap in subject matter. But crucially, both require an explorer’s mindset.
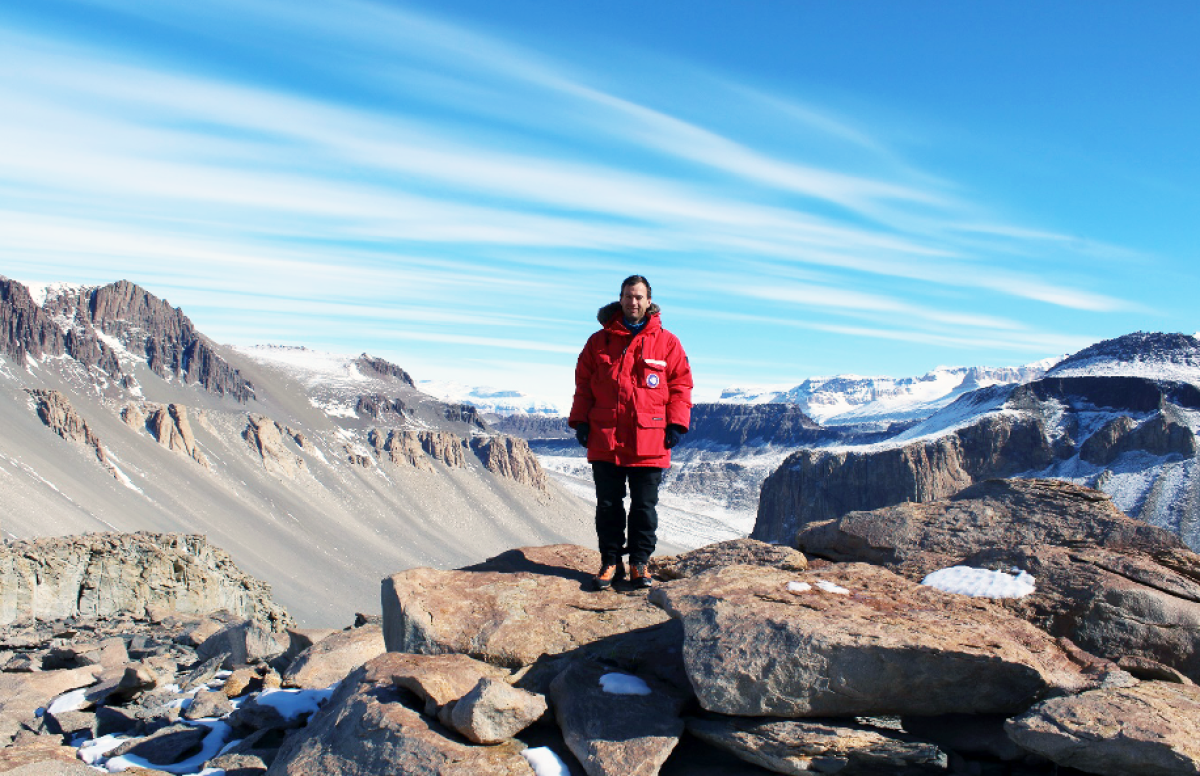
From a young age, Toner was different from his peers. Like other kids, he went to the library to find fun, entertaining reads, but young Jonathan also checked out books on relativity, number theory, and calculus.
“I was pretty curious — maybe a little weird,” he says. “Topics that other people tended to avoid as too difficult, I’d see as a challenge.” When it came to picking a major at the College of New Jersey, the choice was easy: “I’d heard that physics was the hardest, so I did that.”
Making the transition to tech
When grad school beckoned, he decided to combine his passions for science and the outdoors by studying at UW, which is close to the Cascade Range. A few months later, much to his delight, he was conducting field studies at the McMurdo Dry Valleys in Antarctica. It was the first of three expeditions to Antarctica, with the most recent in 2017, funded by NASA and the U.S. National Science Foundation.
I would write an application and say, ‘I’ve got a PhD in geophysics, and I studied planetary science. They’d look at that and be like, ‘Who are you?’
Antarctica is a fertile region for scientific study — particularly the effects of climate change, but also as an analogue for the extreme environments found on Mars or the icy moons of Jupiter or Saturn. In his pursuit of ever-harder questions, Toner moved from physics to geophysics to planetary chemistry to astrobiology. He even investigated the watery origins of life itself.
By 2019, Toner was at the top of his game: a strong publication record and research funding for his ongoing projects at one of the world’s leading institutions for planetary science. But looking into the far future, he didn’t like what he was seeing. He had a strong desire to remain in the Seattle area, but he felt his opportunities to progress in academia were limited, and he had a young family to support, so Toner decided to look for opportunities in the tech industry.
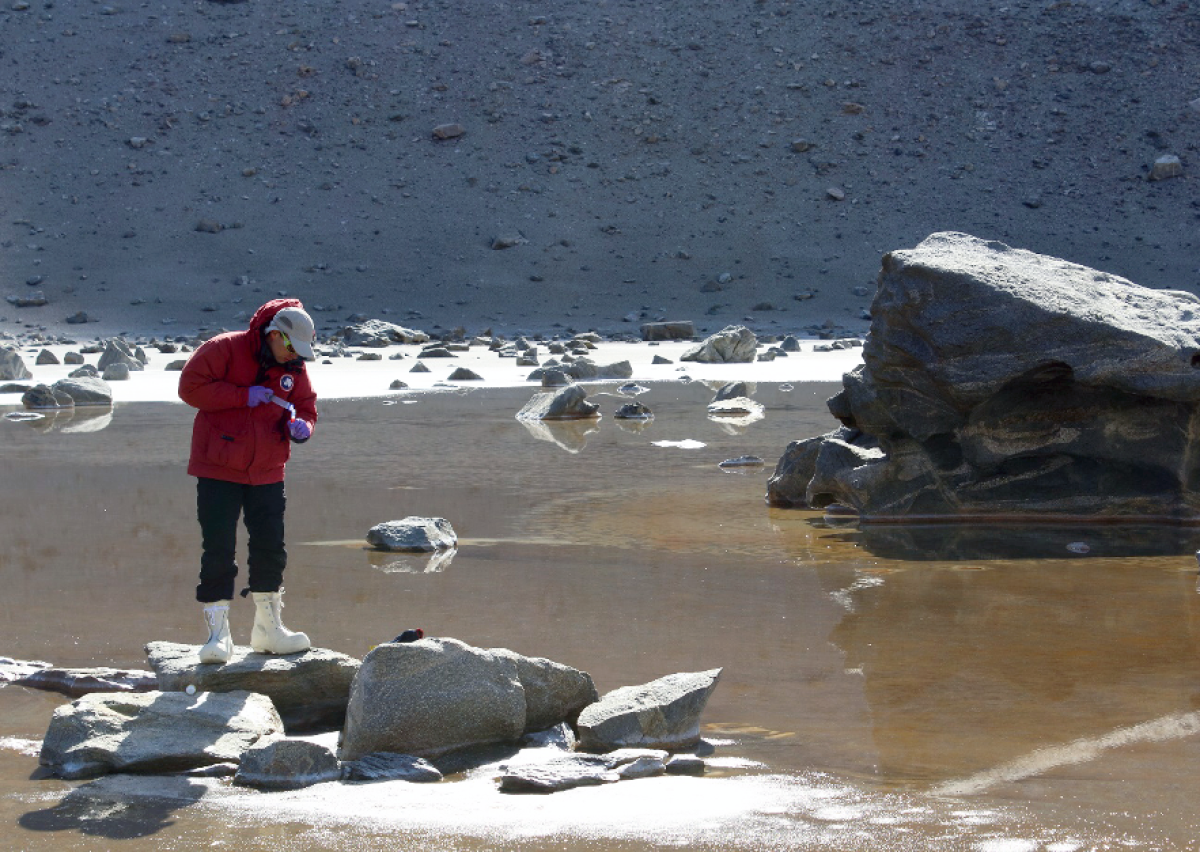
“I had friends in tech who were doing really well and could live wherever they wanted,” he said. “And I thought to myself, ‘I can do that.’”
He started applying for a few positions, but his situation was unusual, to say the least: “I would write an application and say, ‘I’ve got a PhD in geophysics, and I studied planetary science. They’d look at that and be like, ‘Who are you?’”
This is where Toner’s intellectual tenacity kicked in. He had very little need for machine learning in his field and limited programming experience. So, leveraging his strong academic fundamentals, he taught himself both.
“I bought a load of machine learning and stats textbooks. I read them. I did the problems. I took about 10 online courses,” he says. “I taught myself to build machine learning models and the Python programming language.” And he did all that in just three months.
And while Toner didn’t have specific experience in the tech industry, he had credentials from other technical fields. In particular, he had developed complex applied models, including thermal and physical diffusion in Martian soils, and thermodynamic models of multicomponent solutions.
“Machine learning is perhaps a step down in complexity from that,” says Toner, “but it’s like trying to gauge the difference in difficulty between playing the violin and the piano.”
That approach worked and, in May of 2020, Toner joined Amazon in the logistics space.
Satisfying two kinds of customers on Amazon Flex
Package delivery to Amazon customers occurs in a variety of ways, including via Amazon Flex. Launched in September 2015, Amazon Flex offers individuals the flexibility to use their own vehicles and set their own schedules while making deliveries as a way of earning extra money. Amazon Flex drivers pick up customer orders from a local Amazon pick-up location and deliver them directly to customers. The Amazon Flex business was built to support Amazon’s growth and meet customer expectations for fast deliveries.
Amazon Flex drivers are our customers too. So, we have this dynamic of multiple customers to satisfy, and that becomes a really intricate science problem. How do you keep everyone happy? It’s a fun problem to think about.
Toner’s overarching goal is to find cost-effective ways to keep Amazon Flex drivers happy and signing up for more blocks, which ensures the service has enough driver capacity to meet projected demand.
“Amazon is customer-obsessed, and you normally think of the customer as the person receiving a delivery. But Amazon Flex drivers are our customers too,” says Toner. “So, we have this dynamic of multiple customers to satisfy, and that becomes a really intricate science problem. How do you keep everyone happy? It’s a fun problem to think about. It occupies all my time.”
As independent contractors, Amazon Flex drivers have the flexibility to accept offers and schedule blocks when it suits them. This means Amazon needs to make sure the program is attractive to drivers, so they feel confident that their time is well-spent with Amazon Flex. This can be through a combination of things, such as block pricing, promotional activity, and desirable delivery windows.
“A lot of our work is around forecasting what Amazon Flex drivers will do, what they want, and ensuring that they have a good experience ,” Toner explains.
Amazon Flex has been active in the U.S. since 2017 — and it is growing quickly.
“Given that the number of Flex depots and working drivers on any given week is so large, manual adjustments are impractical,” Toner says. So he is working with Amazon product and business operations teams to create smart, autonomous systems that balance customer needs.
Since joining Amazon Flex, Toner has been promoted to senior research scientist and has created an entire ecosystem of machine learning models and optimization tools. This ecosystem is orchestrated to recommend recruiting actions for Amazon Flex in the US. It considers forecasted delivery demand, predicts capacity contributions from existing drivers and potential new recruits, and predicts how drivers will flow through the onboarding process.
“What intrigues me about this ecosystem of tools is that it is many different models all interacting with each other,” says Toner. “And it’s allowed me to work on so many approaches: Markov chain models powered by logistic regression, recurrent neural networks, dynamic programming to find optimal policies. It definitely keeps me interested.”
Intellectual freedom
Toner also appreciates the latitude he is given to create this smorgasbord of tools.
“Coming from academia, I had some reservations. I was worried about being micromanaged. But I was very pleasantly surprised,” says Toner. “My colleagues are lovely people — so smart and supportive. And, crucially, they allow me freedom to do my thing.”
There's rich science to be done with a company like Amazon that is not available elsewhere. I’ve grown enormously as a scientist because of it.
Right now, Toner’s thing is the ongoing expansion of his suite of models, which for now is focused on the US.
“I want to expand worldwide, and I’ve had interest from Amazon Flex in various countries,” he says.
He is also working on long-lead planning for Amazon Flex, which means modelling driver capacity many years in advance. “This is a very hard question. I mean, where do you even start? To me, that’s a gold mine.”
Does Toner have any advice for others in academia considering making the move into the tech industry?
“When you're in academia, it really is like an ivory tower, and going to the corporate world can be viewed with pessimism,” he says. “But I would strongly argue against that. There's rich science to be done with a company like Amazon that is not available elsewhere. I’ve grown enormously as a scientist because of it.”
Toner also counsels against limiting yourself based on what you think you can do:
“Science is really about the interesting questions, right? So, focus on the questions. The methods will come.”