The prices of products in the Amazon Store reflect a range of factors, such as demand, seasonality, and general economic trends. Pricing policies typically involve formulas that take such factors into account; newer pricing policies usually rely on machine learning models.
With the Amazon Pricing Labs, we can conduct a range of online A/B experiments to evaluate new pricing policies. Because we practice nondiscriminatory pricing — all visitors to the Amazon Store at the same time see the same prices for all products — we need to apply experimental treatments to product prices over time, rather than testing different price points simultaneously on different customers. This complicates the experimental design.
In a paper we published in the Journal of Business Economics in March and presented at the American Economics Association’s annual conference in January (AEA), we described some of the experiments we can conduct to prevent spillovers, improve precision, and control for demand trends and differences in treatment groups when evaluating new pricing policies.
The simplest type of experiment we can perform is a time-bound experiment, in which we apply a treatment to some products in a particular class, while leaving other products in the class untreated, as controls.
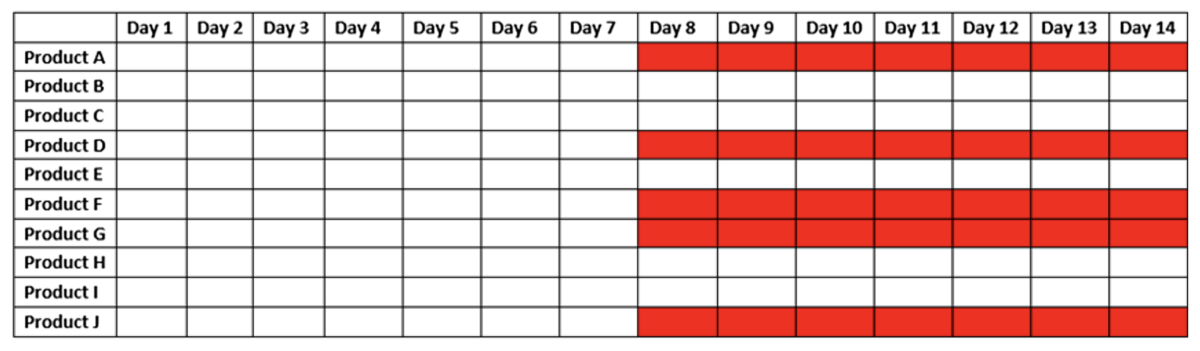
One potential source of noise in this type of experiment is that an external event — say, a temporary discount on the same product at a different store — can influence treatment effects. If we can define these types of events in advance, we can conduct triggered interventions, in which we time the starts of our treatment and control periods to the occurrence of the events. This can result in staggered start times for experiments on different products.
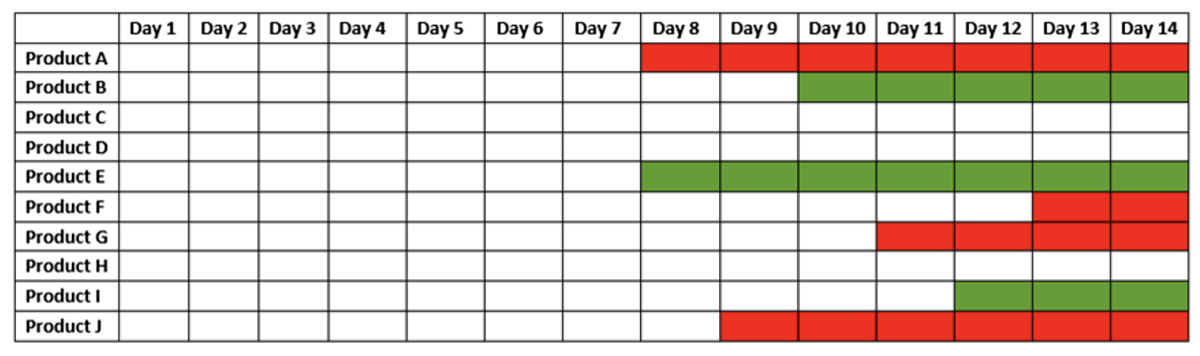
If the demand curves for the products are similar enough, and the difference in results between the treatment group and the control group are dramatic enough, time-bound and triggered experiments may be adequate. But for more precise evaluation of a pricing policy, it may be necessary to run treatment and control experiments on the same product, as would be the case with typical A/B testing. That requires a switchback experiment.
The most straightforward switchback experiment is the random-days experiments, in which, each day, each product is randomly assigned to either the control group or the treatment group. Our analyses indicate that random days can reduce the standard error of our experimental results — that is, the extent to which the statistics of our observations differ, on average, from the true statistics of the intervention — by 60%.
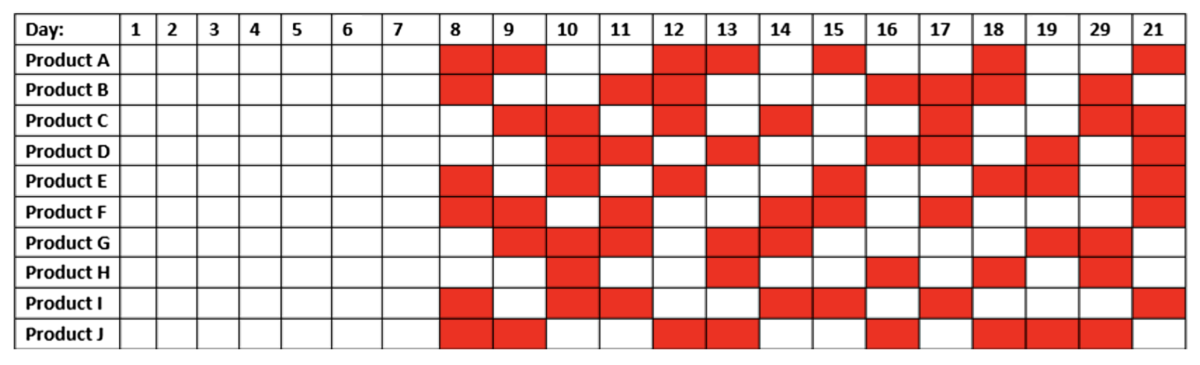
One of the drawbacks with any switchback experiment, however, is the risk of carryover, in which the effects of a treatment carry over from the treatment phase of the experiment to the control phase. For instance, if treatment increases a product’s sales, recommendation algorithms may recommend that product more often. That could artificially boost the product’s sales even during control periods.
We can combat carryover by instituting blackout periods during transitions to treatment and control phases. In a crossover experiment, for instance, we might apply a treatment to some products in a group, leaving the others as controls, but toss out the first week’s data for both groups. Then, after collecting enough data — say, two weeks’ worth — we remove the treatment from the former treatment group and apply it to the former control group. Once again, we throw out the first week’s data, to let the carryover effect die down.
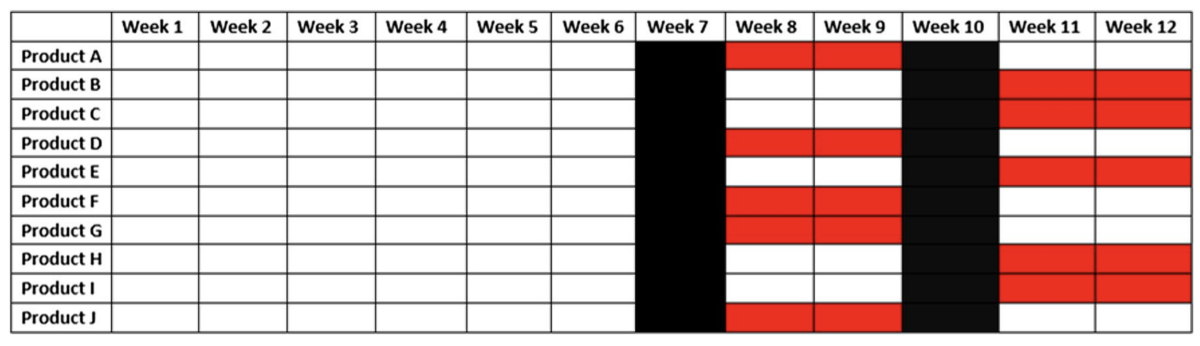
Crossover experiments can reduce the standard error of our results measurements by 40% to 50%. That’s not quite as good as random days, but carryover effects are mitigated.
Heterogeneous panel treatment effect
The Amazon Pricing Labs also offers two more sophisticated means of evaluating pricing policies. The first of these is the heterogeneous panel treatment effect, or HPTE.
HPTE is a four-step process:
- Estimate product-level first difference from detrended data.
- Filter outliers.
- Estimate second difference from grouped products using causal forest.
- Bootstrap data to estimate noise.
Estimate product-level first difference from detrended data. In a standard difference-in-difference (DID) analysis, the first difference is the difference between the results for a single product before and after the experiment begins.
Rather than simply subtracting the results before treatment from the results after treatment, however, we analyze historical trends to predict what would have happened if products were left untreated during the treatment period. We then subtract that prediction from the observed results.
Filter outliers. In pricing experiments, there are frequently unobserved factors that can cause extreme swings in our outcome measurements. We define a cutoff point for outliers as a percentage (quantile) of the results distribution that is inversely proportional to the number of products in the data. This approach has been used previously, but we validated it in simulations.
Estimate second difference from grouped products using causal forest. In DID analysis, the second difference is the difference between the treatment and control groups’ first differences. Because we’re considering groups of heterogeneous products, we calculate the second difference only for products that have strong enough affinities with each other to make the comparison informative. Then we average the second difference across products.
To compute affinity scores, we use a variation on decision trees called causal forests. A typical decision tree is a connected acyclic graph — a tree — each of whose nodes represents a question. In our case, those questions regard product characteristics — say, “Does it require replaceable batteries?”, or “Is its width greater than three inches?”. The answer to the question determines which branch of the tree to follow.
A causal forest consists of many such trees. The questions are learned from the data, and they define the axes along which the data shows the greatest variance. Consequently, the data used to train the trees requires no labeling.
After training our causal forest, we use it to evaluate the products in our experiment. Products from the treatment and control groups that end up at the same terminal node, or leaf, of a tree are deemed similar enough that their second difference should be calculated.
Bootstrap data to estimate noise. To compute the standard error, we randomly sample products from our dataset and calculate their average treatment effect, then return them to the dataset and randomly sample again. Multiple resampling allows us to compute the variance in our outcome measures.
Spillover effect
At the Amazon Pricing Labs, we have also investigated ways to gauge the spillover effect, which occurs when treatment of one product causes a change in demand for another, similar product. This can throw off our measurements of treatment effect.
For instance, if a new pricing policy increases demand for, say, a particular kitchen chair, more customers will view that chair’s product page. Some fraction of those customers, however, may buy a different chair listed on the page’s “Discover similar items” section.
If the second chair is in the control group, its sales may be artificially inflated by the treatment of the first chair, leading to an underestimation of the treatment effect. If the second chair is in the treatment group, the inflation of its sales may lead to an overestimation of the treatment effect.
To correct for the spillover effect, we need to measure it. The first step in that process is to build a graph of products with correlated demand.
We begin with a list of products that are related to each other according to criteria such as their fine-grained classifications in the Amazon Store catalogue. For each pair of related items, we then look at a year’s worth of data to determine whether a change in the price of one affects demand for another. If those connections are strong enough, we join the products by an edge in our substitutable-items graph.
From the graph, we compute the probability that any given pair of substitutable products will find themselves included in the same experiment and which group, treatment or control, they’ll be assigned to. From those probabilities, we can use an inverse probability-weighting schema to estimate the effect of spillover on our observed outcomes.
Estimating spillover effect, however, is not as good as eliminating it. One way to do that is to treat substitutable products as a single product class and assign them to treatment or control groups en masse. This does reduce the power of our experiments, but it gives our business partners confidence that the results aren’t tainted by spillover.
To determine which products to include in each of our product classes, we use a clustering algorithm that searches the substitutable-product graph for regions of dense interconnection and severs those regions connections to the rest of the graph. In an iterative process, this partitions the graph into clusters of closely related products.
In simulations, we found that this clustering process can reduce spillover bias by 37%.