Like many other machine learning applications, neural machine translation (NMT) benefits from overparameterized deep neural models — models so large that they would seem to risk overfitting but whose performance, for some reason, keeps scaling with the number of parameters.
Recently, larger models have mounted impressive improvements in translation quality, but like the models used in other applications, the NMT models are brittle: predictions are sensitive to small input changes, and there can be significant changes in model predictions when models are retrained. Users can be negatively affected, especially if they come to expect certain outputs for downstream tasks.
Especially jarring are cases where the model suddenly produces worse outputs on identical input segments. While these effects have been studied earlier in classification tasks, where an input is sorted into one of many existing categories, they haven’t been as well explored for generation tasks, where the output is a novel data item or sequence.
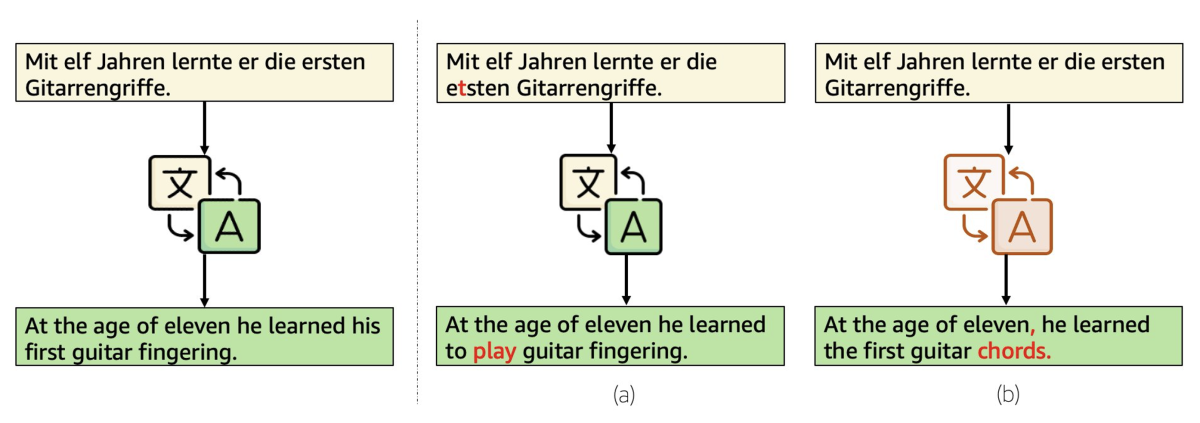
In a paper we recently presented at the International Conference on Learning Representations (ICLR), we investigated the issue of model robustness, consistency, and stability to updates — a set of properties we call model inertia. We found that the technique of using pseudo-labeled data in model training — i.e., pseudo-label training (PLT) — has the underreported side effect of improving model inertia.
In particular, we looked at bidirectional arcs between low- and high-resourced languages (en ↔ de, en ↔ ru, and en ↔ ja), and PLT improved model inertia across all of them. We also introduced a means of measuring regression — in which an updated model backslides on particular tasks — in generation models and show that it is also reduced by PLT. Having observed these effects, we hypothesize that a distribution simplification effect is at play and may hold more generally for other generation tasks.
Experiments
In our experiments, we examined several different flavors of PLT common in machine translation. In certain applications (e.g., non-autoregressive machine translation), unlabeled data or monolingual data is made into parallel data by translating (pseudo-labeling) the monolingual data. This is typically known as self-training or forward translation. In other contexts (e.g., knowledge distillation), it is common to use a larger model (a teacher model) to pseudo-label the training data and train a smaller model (a student model) on the combination of pseudo-labeled and parallel training data.
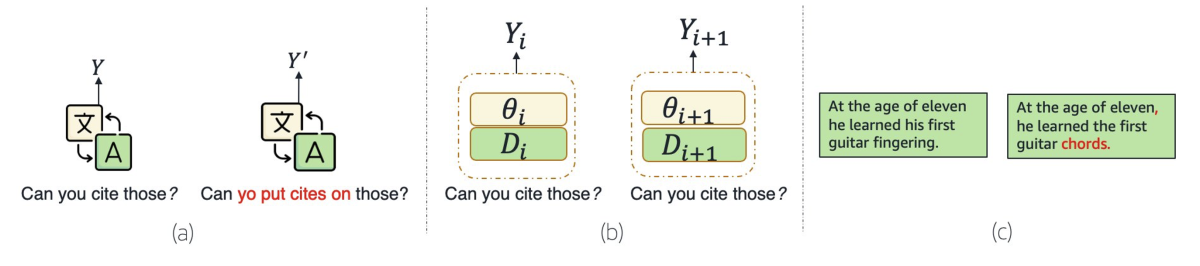
First, we tested the impact that adding pseudo-labeled data has on model robustness to minor variations in the inputs. We looked at synthetically generated misspellings where one character is randomly replaced by another and also at naturally occurring grammatical errors. We then compared the outputs of the machine translation models with and without these variations and measured how consistent (i.e., similar) the outputs are and the robustness of the models (i.e., how much quality degrades). We found that training on pseudo-labeled data makes models more consistent and that this wasn’t a function of the amount of training data or the size of the teacher model.
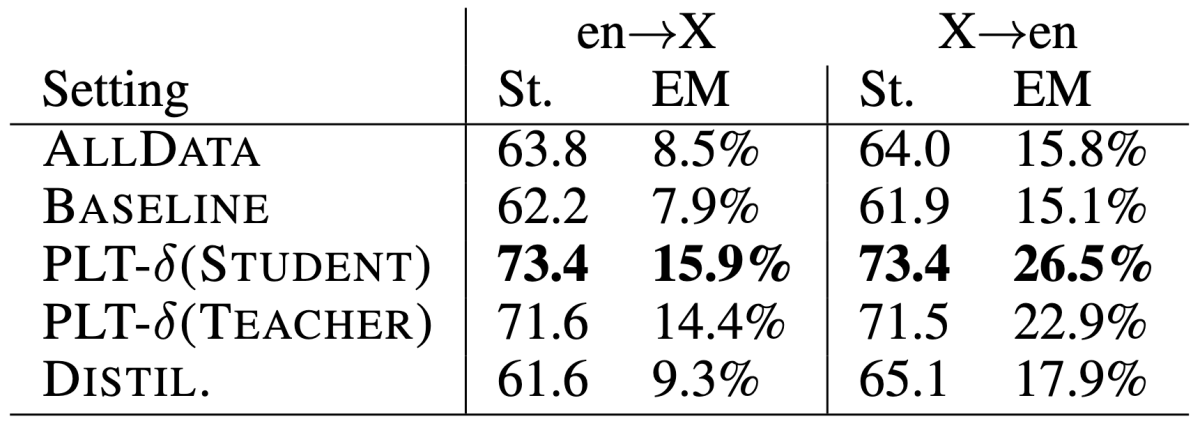
We also considered the scenario in which models are incrementally updated (i.e., no changes to the model architecture, no major changes to the data, etc.) and tested whether models were more stable when we changed random seeds in student models or teacher models. We looked at the number of segments that were exact matches (EM) of each other and the stability (St.) of the models, which we defined as the lexical similarity of the outputs under changes in random seed. Surprisingly, we found that upwards of 90% of outputs are changed, just by changes in random seeds. We found that with pseudo-label data, the models are more stable by 20%, and close to double the number of segments are similar.
Given the large number of output changes, we naturally asked if the model makes worse translations for specific inputs, i.e., negative flips. Previously, negative flips have been studied in the context of classification, but in machine translation, the concept is more nebulous, since metrics can be noisy on the level of sentence segments. Consequently, we used human evaluations of our models to see if models had regressed.
Given the limitations in human evaluations, we also looked at a targeted error category that allowed us to measure segment-level regression automatically. In this work, we adopted gender translation accuracy as the targeted error and tested on the WinoMT dataset. We found that PLT methods reduce the number of negative flips in terms of regressions on the targeted and generic quality metric.
A hypothesis
Having observed an improvement in the model inertia of models trained on pseudo-labeled data, we set out to investigate the reasons behind it. We hypothesized that the improvement comes from a distribution-simplification effect similar to one seen in non-autoregressive MT. To test this idea, we conducted experiments comparing pseudo-label training with several other techniques well-known in MT for producing more robust models: BPE-Dropout, Back-translation, and n-best sampling.
We then looked at how each of these methods reduced the complexity of the training data, using a metric called the conditional entropy. Across the methods we experimented with, we found that model stability is correlated with simpler training distributions as measured by the conditional entropy.
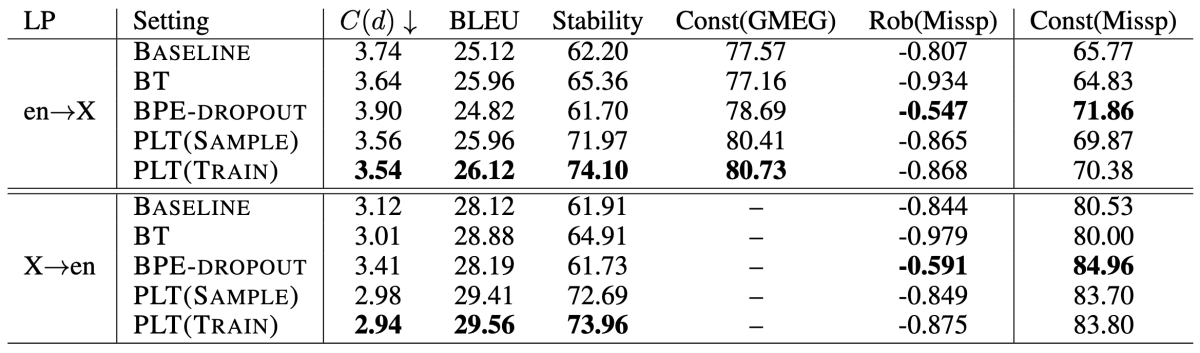
As we enter an era where ever larger neural networks come into wider use to solve a variety of generation tasks, with the potential to shape the user experience in unimaginable ways, controlling these models to produce more robust, consistent, and stable outputs becomes paramount. We hope that by sharing our results, we can help make progress toward a world where AI evolves gracefully over time.