As machine-learning-based decision systems improve rapidly, we are discovering that it is no longer enough for them to perform well on their own. They should also behave nicely toward their predecessors. When we replace an old trained classifier with a new one, we should expect a smooth transition and a peaceful transfer of decision powers.
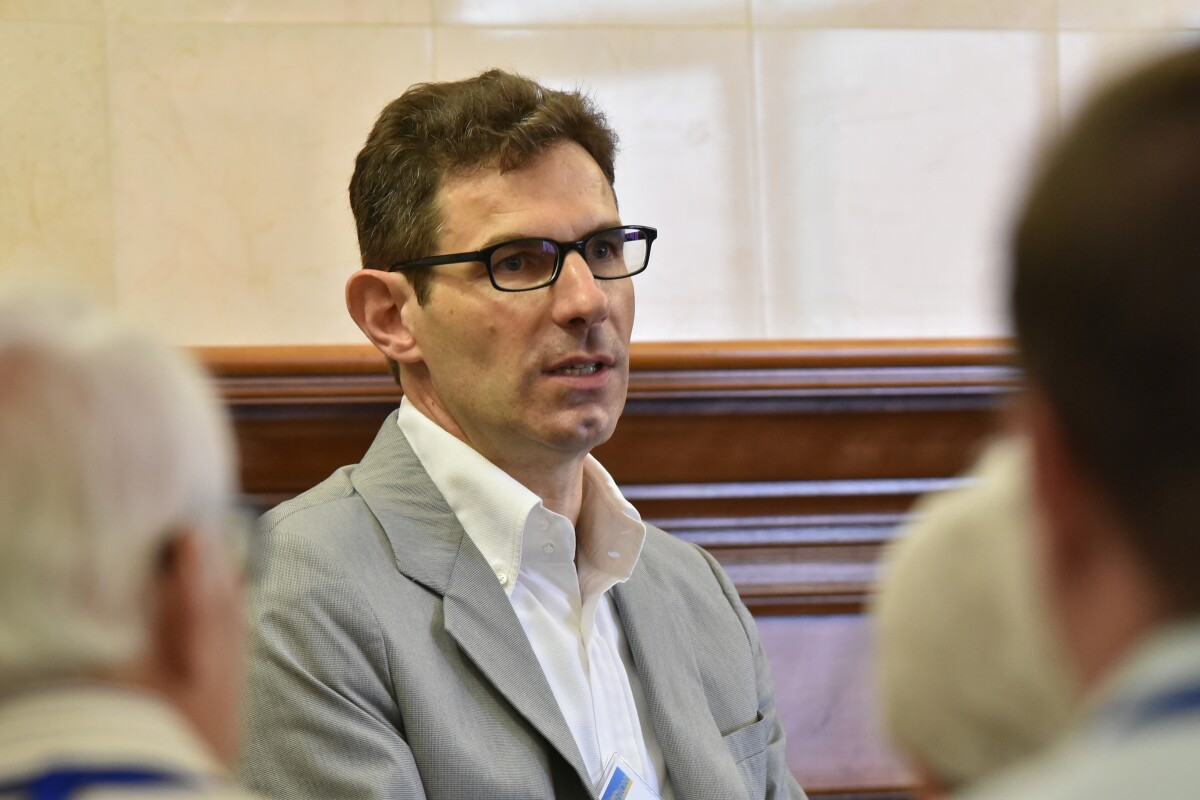
At Amazon Web Services (AWS), we are constantly working to improve the performance of our learning-based classification systems. Performance is typically measured by average error on test data that are representative of future use cases. We scientists get very excited when we can reduce the average error, and we hope that customers will be delighted when they replace the existing system with a new and improved one.
However, it is possible for a new model to significantly improve average performance and yet introduce errors that the old model did not make. Those errors can be rare yet so detrimental as to nullify the benefit of the improved model. In some cases, post-processing pipelines built on top of a model can break. In other cases, users are so accustomed to the behavior of the old system that any introduced error contributes to a perceived “regression” in performance.
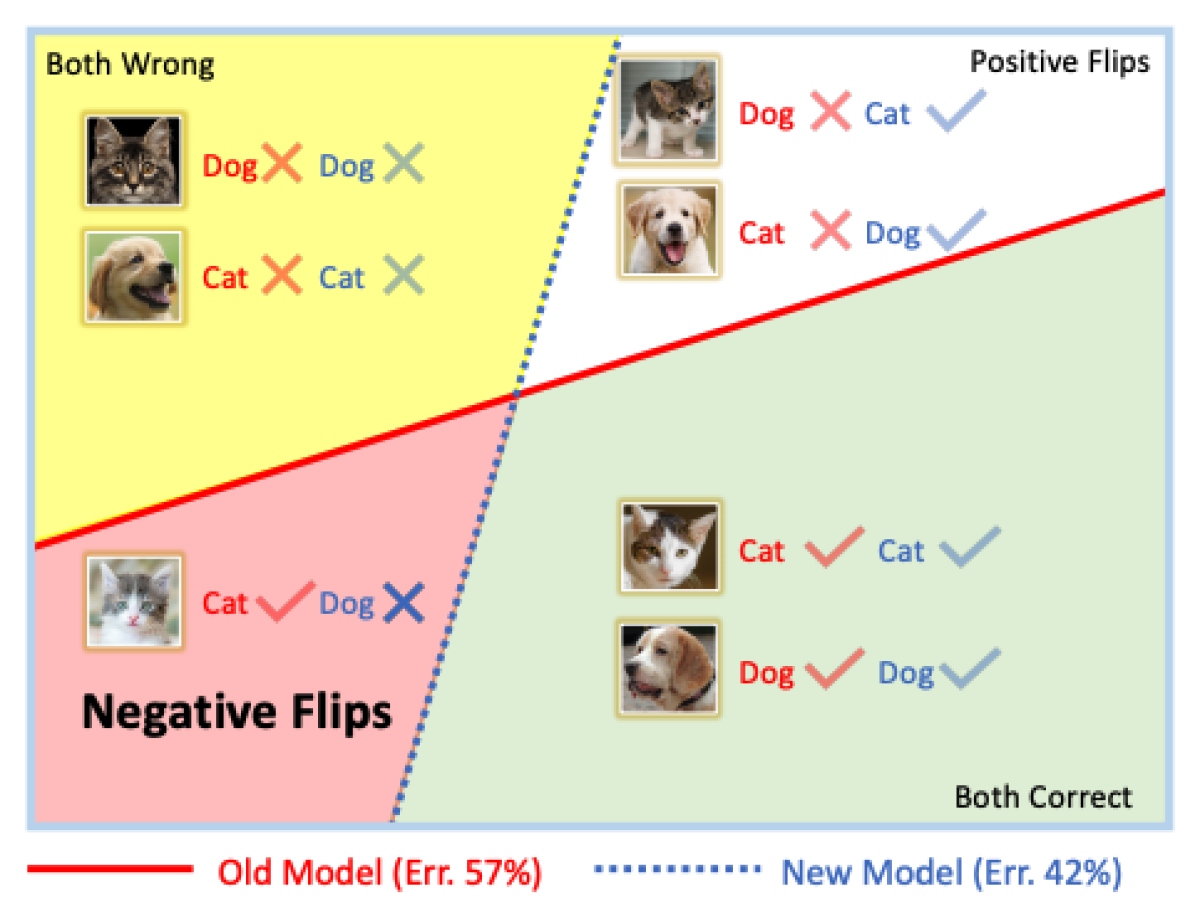
You may have experienced this phenomenon when using the search feature in your photo collection. Occasionally, the provider updates the photo management software, presumably improving it. However, if an image that you were able to retrieve previously suddenly goes missing from the search, the natural reaction is surprise: How is this version any better? Give me the old one back!
When the software update occurs, the search feature is usually unavailable for a period of time; the larger your photo collection, the longer the interruption typically lasts. During this time, the system reprocesses old images to create indices and clusters them based on identities. If the model introduces new mistakes, old images may be left out of searches that used to retrieve them.
Which prompts the question, Why is it necessary to reprocess old data? Can we design and train new learning-based models in a manner that is compatible with previous ones, so that it is not necessary to reprocess the entire gallery?
These questions generally pertain to the need to train machine-learning-based systems, not in isolation, but in reference to other models. Specifically, we want the new models to be compatible with classifiers or clustering algorithms designed for the old models, and we want them to not introduce new mistakes.
Compatible updates
Today, requirements beyond accuracy have begun to drive the machine learning process. These demands include explainability, transparency, fairness, and, now, compatibility and regression minimization. We call the ability to meet those demands “graceful AI”.
We at AWS first faced this challenge when responding to a customer request to reduce the cost of re-indexing data, which can be significant for large photo collections.
At the time, there was no literature on the topic. We trained a deep-learning model to minimize the average error while using the “classifier head” of an old model — the last few layers of the model, which issue the final classification decision. In other words, we forced the data representation computed by the new model to live in the same space as the old one, so the same clustering or decision rules could be used without the need to re-index old data.
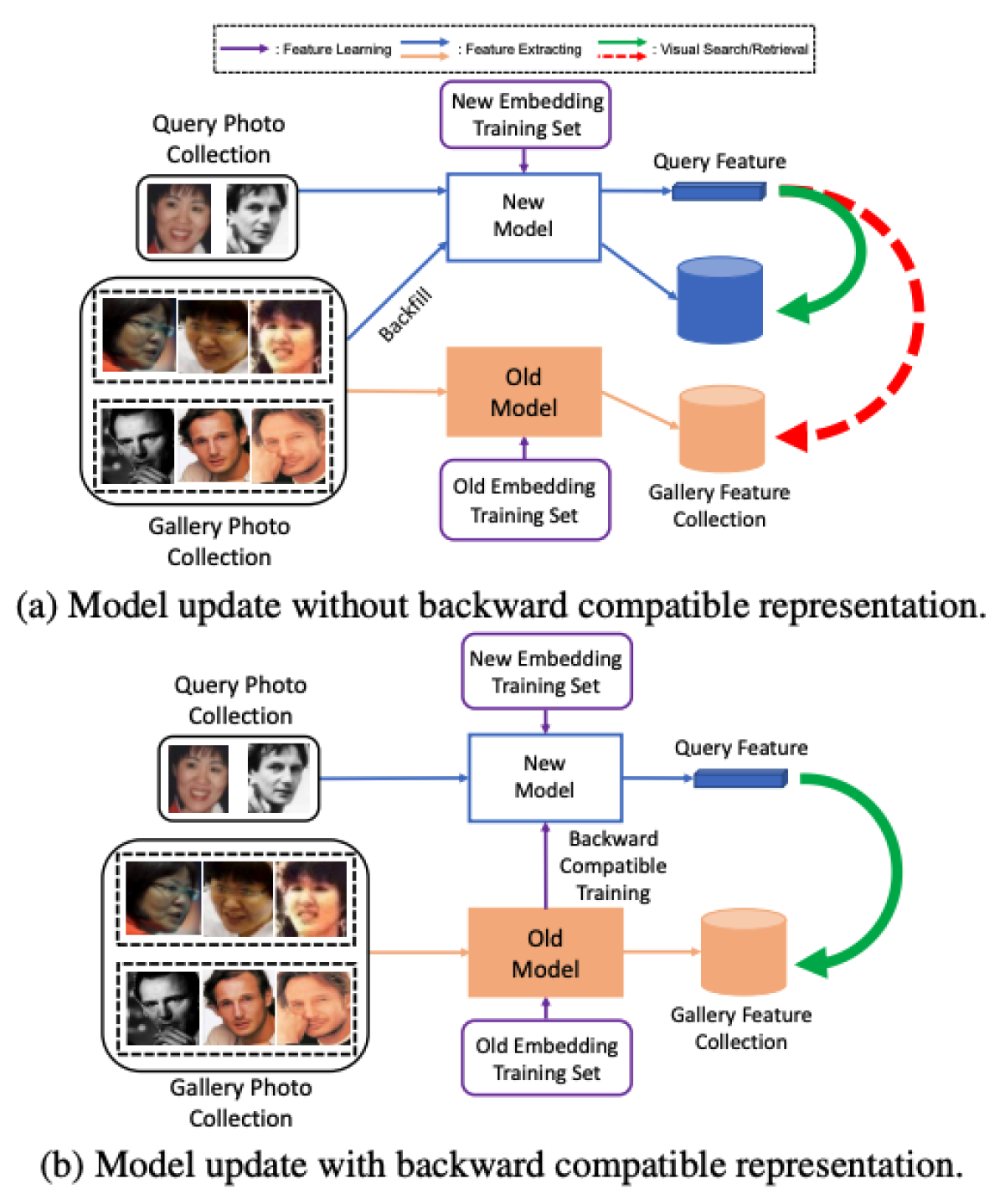
If this approach worked, customers could start using new models immediately, with no re-indexing time or cost, and the old indexed data could be combined with the new. And it did work, as we described in the paper “Towards backward-compatible representation learning”, presented at last year's Conference on Computer Vision and Pattern Recognition (CVPR). It was the first paper in this increasingly important area of investigation in machine learning, around which we are organizing a tutorial at the upcoming International Conference on Computer Vision (ICCV).
For services that require more complex post-processing than clustering, it is paramount to minimize the number of new errors introduced by model updates. In a forthcoming oral presentation at CVPR, our team will present an approach that we call positive-congruent training, or PC training, which aims to train a new classifier without introducing errors relative to the old one. This is a first step towards regression constrained training. PC training is necessary to avoid rare but harmful mistakes that you wish to never make.
PC training is not just a matter of forcing the new model to mimic the old one — a process known as model distillation. Model distillation mimics the old model, including its errors; we want to be close to the old model only when it gets it right.
Even when the average error is reduced to a minimum, it is still possible to reduce what we call the “negative flip rate” (NFR), which measures the percentage of new errors compared to the old model. This can be done by trading errors, keeping the average error rate constant (unless the average error rate is precisely zero, which is almost never the case in the real world). So minimizing the NFR is a separate criterion from the standard error rate, and PC training represents a new branch of research in machine learning.
It is possible for a new model to significantly improve average performance and yet introduce errors that the old model did not make. Those errors can be rare yet so detrimental as to nullify the benefit of the improved model.
Machine-learning-based systems will continue to evolve, and eventually we will do away with the artificial separation of training (when the model parameters are learned from a fixed training dataset) and inference (when new data is presented to elicit a decision or action). As we make steps toward such “lifelong learning”, it is important for new models developed in the meantime to play nicely with existing ones.
We have sown the first seeds of work in this area, but much remains to be done. As models are repeatedly updated, a growing set of compatibility constraints will ultimately weigh negatively on overall performance, much as backward compatibility with all previous versions makes some software so unwieldy.
We are pleased that some of our models at AWS AI Applications are already backward-compatible, which means that customers will be able to upgrade to new models without having to change their processing pipelines or re-index old data. In 2021, any transfer of decision power should occur without drama.
Modified models
Another version of the incompatibility problem arises when one wishes to deploy the same system on different devices with diverse resource constraints. One might, for instance, have a large and powerful model running in the cloud and smaller versions of it running on edge devices such as smartphones.
We’ve found that, to ensure compatibility, it’s not enough for the smaller models to approximate the accuracy of the large model; they also need to approximate its architecture. Again at the next CVPR, we will present a paper on “heterogeneous visual search”, which shows how to enforce this type of compatibility across platforms.
Finally, all of the above would be easier if deep neural networks were linear systems, and training consisted of minimizing a convex loss function. As we all know, this is not the case. The niche literature on linearizing deep neural networks has mostly focused on analyzing those networks’ behavior; their performance has been far below that of the full nonlinear, nonconvex originals.
However, we have recently shown that, if linearization is done right, by modifying the loss function, the model, and the optimization, we can train linear models that perform just as well as their nonlinear counterparts. “LQF: Linear quadratic fine-tuning”, also to be presented at CVPR, describes modifying the architecture of a ResNet backbone by replacing ReLu with leaky ReLu, modifying the loss function from cross-entropy to least-square, and modifying the optimization by preconditioning using Kronecker factorization.
We are excited to continue exploring how these and other developments can lead to more transparent, more interpretable, and more “gracious” AI systems.