As the director of the University of Florida Informatics Institute, George Michailidis, who is also an Amazon Scholar on the Supply Chain Optimization Technologies (SCOT) team, leads a diverse community of data scientists with training in engineering, statistics, applied math, and other sciences. He notes that assortment of backgrounds is important in data science.
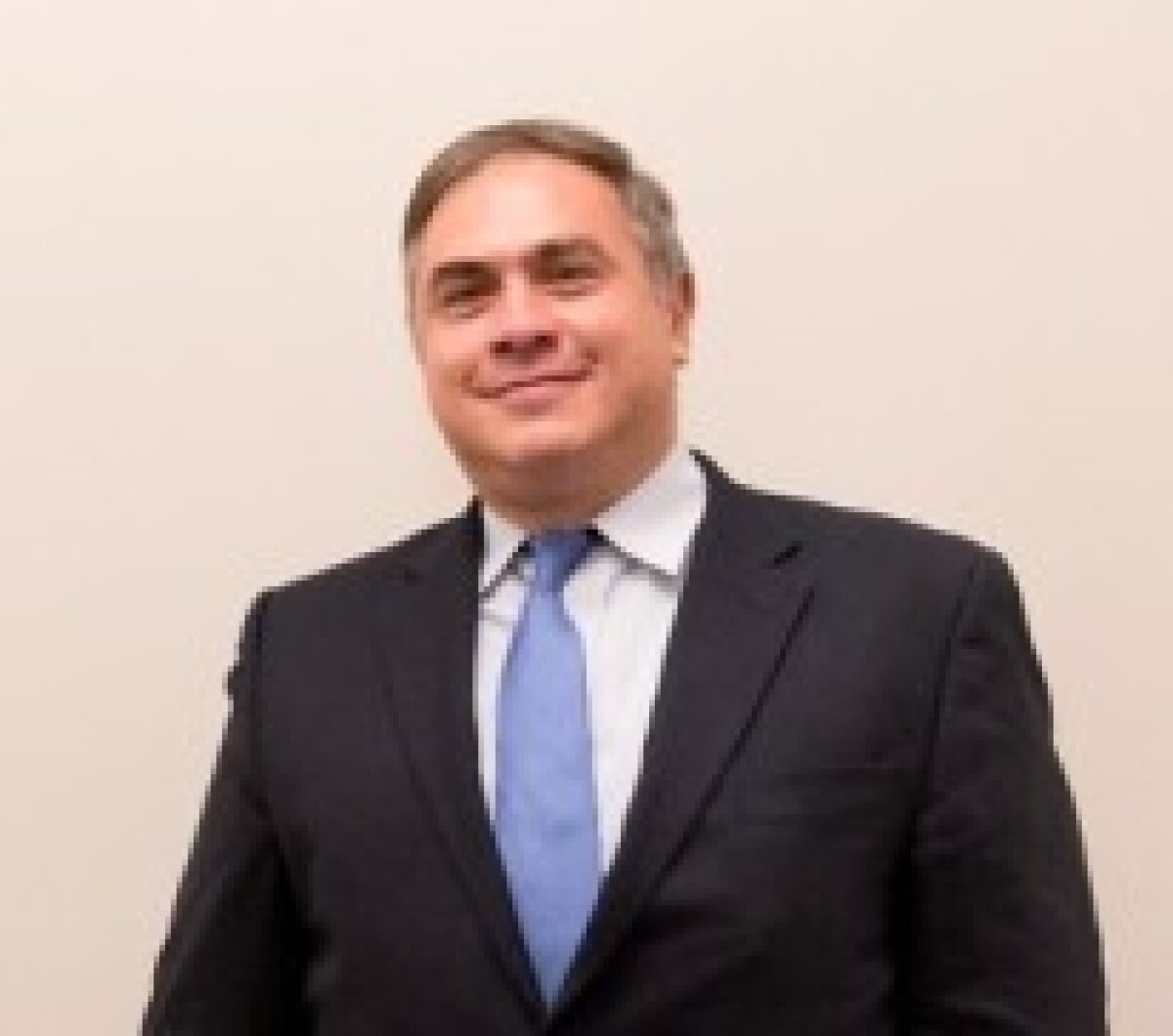
“In addition to statistics, there are a number of other disciplines that data scientists need to be aware of, such as programming, algorithms, optimization, and of course, some subject matter expertise because you don't do data science in a vacuum,” he says.
Michailidis was trained in applied mathematics and statistics, with a PhD thesis focused on optimization problems and its applications to statistical problems. His postdoc was in operations research, which introduced him to a different class of problems. “Some of them come about in Amazon’s supply chain, for example, such as problems of how to schedule the jobs on the machine, or how to route the traffic in the network, and so forth.”
For about 17 years, Michailidis was a faculty member at the University of Michigan in statistics with a joint appointment in electrical engineering. “I combined my statistical training with my interest in engineering types of problems.”
Data integration
Since then, his research agenda at the University of Florida has had strong theoretical components, but he remains very interested in practical applications. One of his current interests is data integration, and its many potential uses. For example, when it comes to the study of diseases, there is a wealth of molecular-level data from patients’ samples. At the same time, there is information on the patient's clinical records and demographics.
“How do you create models to try to identify key drivers, for example, for disease progression by combining all these different data sources,” is one of the questions that motivates Michailidis’ work. With these models, he tries to provide insights both for prognostic or diagnostic purposes, but also for the understanding of the biological mechanisms that lead to that disease.
Another large component of Michailidis’ research relates to a problem known as anomaly detection. “This is an old problem that has been going on for more than 60 years,” he says. To a large extent, it originated in manufacturing, where people were interested in finding defects in the manufacturing process and fixing them. As the technology evolved, similar questions have been arising in many other fields.
This is broadly the theme of a paper published by Michailidis and his colleagues Hossein Keshavarz, a senior data scientist at relationalAI, and Yves Atchadé, a professor of statistics at Boston University, entitled “Sequential change-point detection in high-dimensional Gaussian graphic models.”
Michailidis notes that, as manufacturing processes became more complex, it became necessary to monitor many more metrics.
“A typical example of this complexity is semiconductor manufacturing, where you have to monitor hundreds of little things,” he says.
In more modern applications, the next step is to monitor networks.
“You’re not only monitoring a lot of things. Now these things are interconnected and you're trying to understand how this network, as an object, changes its structure at some point in time,” Michailidis explains. “And you're doing that in an online fashion because this process keeps going. You keep observing the network and you're trying to identify changes as quickly as possible.”
In addition to developing a technique to detect changes, researchers also must establish that their technique is sensitive enough for certain types of changes and determine whether it detects them quickly enough. This is the challenge, in the online realm, that Michailidis and his colleagues attempt to address in their paper. The paper introduces “introduces a novel scalable online algorithm for detecting an unknown number of abrupt changes”.
In the paper, the authors present an application on stock market data, where the network is made of movements of stocks. “We showed how the network changes, for example, during the great financial crisis of 2008, and how the stock market got affected by the European debt crisis in 2012 and so forth.” Michailidis notes that these techniques are especially suited for problems where there are dependencies between observable elements without knowledge of the nature of those dependencies.
“With stocks, whether they are moving together or in different directions, these movements —or lack of movement — is what gives rise to the network structure. And that’s what we are capturing with these graphical models,” he says.
Within the SCOT organization, Michailidis says he has the opportunity to tackle challenging problems at an unprecedented scale. “The problems are much more complex because they're not as clear cut as they are in academia.” In this interview, he discusses his research on anomaly detection and its potential applications.
- Q.
Your paper mentions high dimensional piecewise sparse graphical models. What does that entail and what are some applications?
A.The graphical model is a particular statistical model that tries to capture statistical dependencies between the things that are measured on the nodes. In the stock market example, you're looking at the rate of return of a stock. This is the measurement that you have on every node over time and you're trying to understand, for example, whether the return of one technology stock is correlated with the return of some other technology stock. So that's what the graphical model is trying to capture — the statistical dependencies.
The next step is what we mean by high dimensional. Essentially, it means that the number of nodes, or variables, in your network becomes very large compared to how many observations you have. You may have a short observation period, but with a high number of nodes. What we call high-dimensional statistics became a big field of study 15 to 20 years ago, with a lot of applications. The reason is that, in more classical statistics, we always made the assumption that the sample size in our observations is much larger than the number of variables. In the high-dimensional regime, the relationship flips and you have many more variables than observations and that poses a whole bunch of technical challenges, to the point where you can’t even solve the problem.
So, you need some additional assumptions, and that's where another important term comes in: sparse. This means that this network doesn't have too many connections. If it was very well connected, then we would not be able to solve the problem for technical reasons, because you would not have enough data. So, you make the assumption that these networks are not too connected to compensate for how much data you have.
And the last term we need to understand is piecewise. By piecewise, we mean that, for this period, the network structure stays the same, and then changes abruptly to some other structure. It's not a gradual change — although this may be happening in reality. It heavily depends on the underlying application. It may either be a simplifying assumption in order to do the analysis or, in many cases, that's exactly what happens.
In the neuroscience example, if the subject sits in the scanner without moving, and then you tell them — “raise your hand or read this sentence” — there is an abrupt change because there is a new task after a resting state. This is also possible in the stock market, where new information may create these abrupt changes.
In many applications, there is really an abrupt change and this is the proper setting to use. In some other cases, changes may be a little bit more gradual. But we can still look at them as abrupt changes because it becomes a good working hypothesis and simplifies things. A lot of these techniques that people develop are good working models, and not exactly what's going on, that's fairly standard in a lot of scientific fields. And that explains the high dimensional piecewise sparse graphical model. That's where all the pieces come together.
- Q.
Why is it important to be able to detect these abrupt changes in an online setting?
A.Because you keep collecting the data, and you would like to identify these changes as things evolve. You could solve the same problem, with the same high dimensional sparse piecewise graphical model, in an offline manner. In that case, the difference is that you have already collected these data and would like to explore them in a retrospective manner to see if you can find these types of changes. That's also a problem of interest.
The reason that in this article we focus on online detection is that we have already done work on the offline version, so it was natural to start exploring what is different in an online setting. And it's much, much more challenging, because you don't know the future and you keep getting new information, and you're trying to detect these changes quickly. Online problems in machine learning and other areas are more challenging than offline problems, as a general rule. So, this is for me a natural evolution, since I’ve already used these sparse graphical models in an offline setting.
- Q.
What does the paper demonstrate and how is it applicable to Amazon?
A.The paper does demonstrate that it is possible to detect these changes online, so it’s a positive message. And it also shows a caveat. If, for example, the changes in this connectivity pattern were concentrated on only one node, then we could not detect them with the current technology. Because that's a very localized change, it only involves a very tiny part of the network. And our technique would only be able to detect them by waiting for a very long time. From an applications perspective, that makes it uninteresting. That tells you the limitations, which are important in some settings. We have done most of the work, but we found out that we were missing something. So, we need to go and develop a little bit more.
The results could be applicable to Amazon because these graphical models come up a lot. So far, we have used techniques where we haven't taken the connections into account, we have just looked simply at what is going on in the time series, let's say, of a single node and whether that changes. Obviously, given the fact that Amazon operates in a highly volatile environment, changes are important. In the longer term, given the fact that the team has done work with graphical models, it may be interesting to utilize some of these techniques. The potential is there.
In general, anomaly detection work to date across many disciplines (statistics, signal processing, machine learning, econometrics) has largely focused on parametric models, where with some effort the theoretical properties of anomaly detection procedures can be elucidated analytically and then validated through simulations. The analytical work provides deeper insights into the performance of these anomaly detection procedures and their limitations, and when they do not perform well. With all the advances in deep learning models, they become prime tools to use in anomaly detection problems.
However, the challenge then becomes, to understand the performance limits of such models, beyond relying on numerical work. Such advances may take some time, but once the community makes progress, much more powerful procedures will be available to the practitioners.