At the age of 12, Alex Wiltschko bought his first perfume, Azzaro pour Homme. He’d read about it in his favorite book — Perfumes: The Guide, by Luca Turin — and was thrilled to find it at a knock-down price at his local TJ Maxx. It would be the first in a large collection.
For as long as he can remember, Wiltschko has been obsessed by scent. “It’s how I’m wired,” he says. His other obsession? Computers. “An interest in perfumes and computers was not the recipe for social success as an adolescent,” he adds.
It was, however, the recipe for a life trajectory that took Wiltschko deep into the neuroscience of olfaction and cutting-edge machine learning. This combination has placed Wiltschko at the forefront of the nascent science of digital olfaction — a.k.a. giving computers a sense of smell.
Wiltschko is now the CEO of Osmo, a Google Research spinout based in Cambridge, Massachusetts. In September 2022, the company hit the ground running with $60 million in initial funding, including an investment from the Amazon Alexa Fund.
In the short term, Osmo aims to unlock a new era of commercial fragrance innovation. Longer term, the company envisions its technologies having the potential to save lives through the development of better insect repellents and even digital diagnostic tools for detecting serious illnesses on a person's breath.
The Principal Odor Map
The keystone to all this is the team’s breakthrough advance: the creation of what it calls the Principal Odor Map (POM).
Before vision could be digitized, a map called RGB was required: It shows how every color is made up of varying proportions of red, green, and blue. Before Osmo was spun out, Wiltschko’s team did something similar — and remarkable — with odor. They used machine learning to map the structure of a molecule directly to how humans perceive the smell of that molecule. In other words, they built a model that can tell you what a molecule smells like just by looking at it. This is the POM.
That was an ‘a-ha!’ moment for us, akin to passing a Turing test for odor. We'd built something with real commercial value that was sufficiently validated to bring into the world.
Here’s how they created POM and, crucially, how they proved it worked. They first trained a graph neural network (GNN) on about 5,000 molecules from several flavor and fragrance databases. The smells of all these molecules were well-documented with multiple human-judged odor labels such as beefy, floral, or minty. From this, the model was able to learn connections between molecular structure and odor, without needing any knowledge of what actually happens in the nose or brain of a person sniffing an odor.
That’s great, as far as it goes. The crucial question then was, could POM generalize to predict the smell of molecules it had never seen before, based solely on their molecular structure? And could it do that as well as trained human raters, which is the gold standard for odor characterization? To find out, the team took a diverse set of more than 400 odor molecules previously unseen by POM and had the model blindly predict their characteristics. Then a panel of trained human raters sniffed and labeled those same odors.
When the Osmo team compared the results, they were delighted. Not only had the model successfully predicted the odor of these unseen molecules as well as trained humans, but its predicted odor profiles were closer to the average results of the panel than any of the individual panelists themselves.
“That was an ‘a-ha!’ moment for us, akin to passing a Turing test for odor,” says Jon Hennek, chief product officer at Osmo. “We'd built something with real commercial value that was sufficiently validated to bring into the world.”
Islands of odor
POM is not a map in the typical sense, but it can nevertheless be compared to the RGB map. Pick two points at random on a two-dimensional color map. The closer those two points are to each other, the more similar the color. The same is true for odors in POM, though this map exists in a mind-bending 256 dimensions. All of the tulip-smelling molecules are close to each other, for example. Ditto for the brandy-smelling molecules.
“Zooming out a little, all the flowers are next to each other. There's a whole floral Pangaea in this odor map! We didn't tell it to do that,” says Wiltschko. This sort of grouping is also true for woods, bakery-type smells, alcoholic smells, you name it. Our brain seems to organize smells in nested hierarchies, says Wiltschko, so the rose odor is inside the rose category, inside the flowers category, inside the plants category, inside the pleasant category.
“The fact that we were able to observe this in the POM without telling it is astounding,” he says.
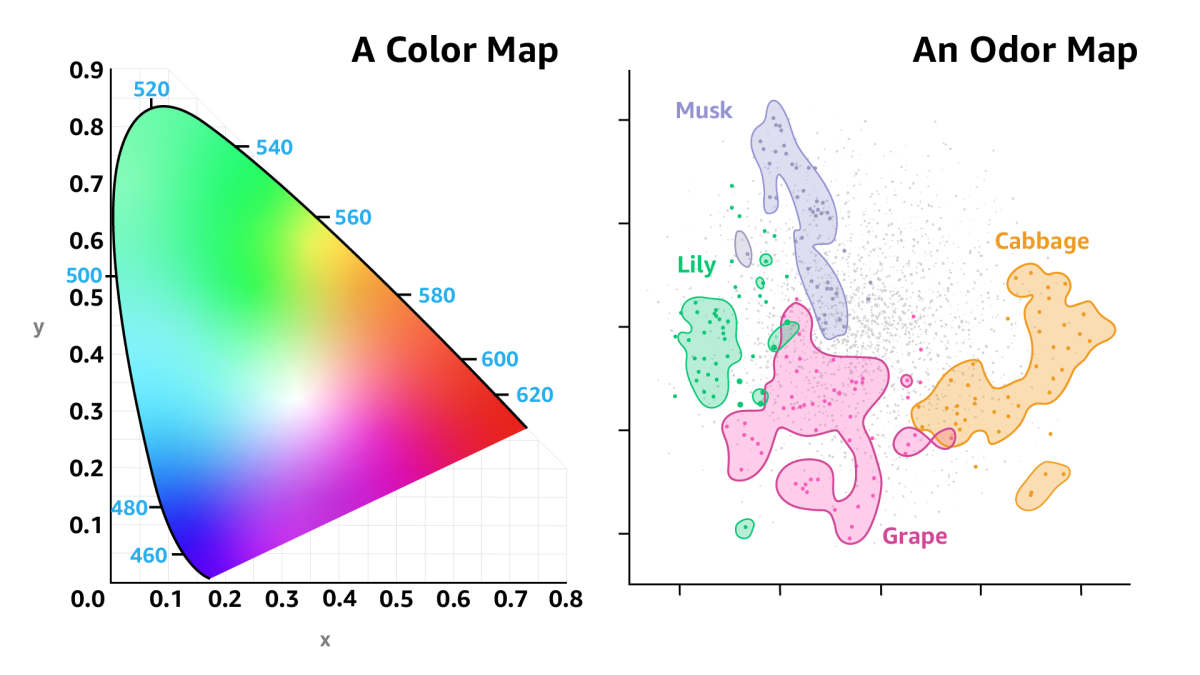
While Wiltschko has bold ideas for the future of Osmo’s technology, the first order of business is putting the company on a solid commercial footing. For now, Osmo is concentrating on developing new ingredients for the global fragrance category.
The Osmo team is using POM to explore the world of odor molecules — several billion of them — and homing in on molecules that POM predicts to have an interesting and strong olfactory character.
“We're much better at that, I believe, than anybody else in the world,” says Hennek. “Because rather than start with rules of thumb and chemical intuition, we are starting with an odor prediction for every molecule we could possibly synthesize. It lets us find molecules that a chemist might never have considered.”
The team is working with advisors, including Christophe Laudamiel, a French master perfumer, and potential customers include fragrance houses and packaged goods companies.
“We've had repeated feedback that our ingredients have the potential to be very successful, commercially,” says Wiltschko. “That smells like product/market fit.” The principal idea is to license those molecules to fragrance houses.
It’s a timely endeavor. The global fragrance category is valued at more than $10 billion and growing steadily. But some traditional ingredients, such as sandalwood oils, can result in over-harvesting or other ecological harm, while the characteristics of other ingredients increasingly fall short as the demand grows for safer, more biodegradable products.
With POM, Osmo is paving the way for palettes of safe, synthetic fragrances that recreate natural odors using environmentally friendly and easily synthesized molecules. To that end Osmo is looking at combinations of just a handful of atoms: carbon, hydrogen, oxygen, nitrogen, phosphorus, and sulfur.
“Then we bring them into our lab for a process akin to a drug discovery pipeline,” says Hennek. “We are working towards regulatory approval of those molecules.”
Rise of the graph neural networks
All of this has only become possible in the last six years or so. The core insight that started this scientific project, says Wiltschko, was that machine learning was “getting really good at molecules,” thanks to the recent rise of GNNs.
Previously, machine learning approaches primarily converted inputs — images or data arrays, say — into rectangles or data grids to process them. Molecules didn’t fit this mold: a molecule might be two atoms, or it might be 20 atoms, with wildly different structure and connectivity. They are simply not reducible to rectangles or grids.
Instead, the atoms in a molecule can be considered as nodes, and the chemical bonds between them as edges, forming a graph structure. This representation allows GNNs to model and process molecular data.
“Some of this technology was developed by friends of mine at Google. So, it was a fantastic, fertile ground to start exploring this idea,” says Wiltschko.
This ongoing exploration is creating some exciting possibilities. Wiltschko reasoned that, just as the sun has shone on Earth since before life began, resulting in many creatures evolving similar visual apparatuses, the composition of the Earth’s atmosphere has been broadly stable over evolutionary time. So could POM also be used to understand the olfactory responses of other species, even those separated from humans by millions of years of evolution?
Life-saving potential
Take mosquitos. Could POM be used to work out what odors repel these disease-carrying insects?
To find out, they augmented POM with additional data sources. The first was a long-forgotten U.S. government report, published in the 1940s, that featured the results of testing 19,000 compounds for their mosquito repellency. The second was information provided by TropIQ, a Dutch company that develops malaria-control technology. The augmented model was soon able to predict entirely new molecules with repellency at least as powerful as DEET, the active ingredient in the most effective mosquito repellents.
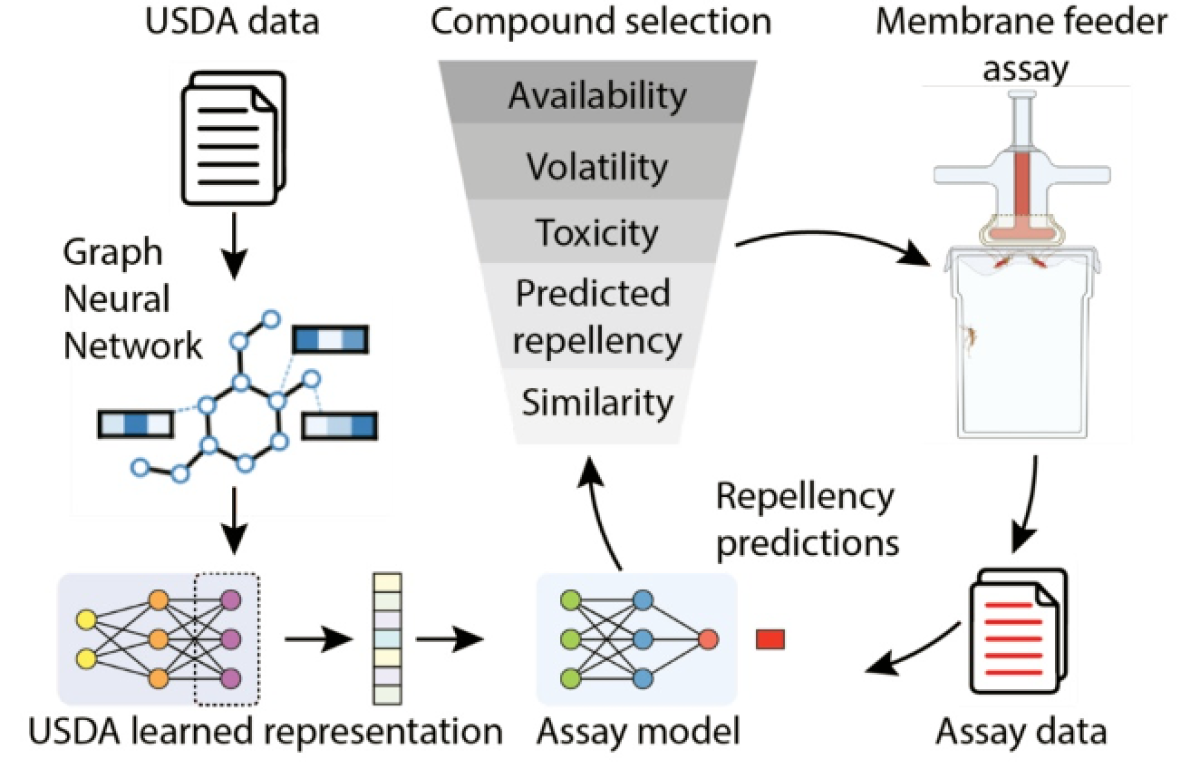
The development of cheaper, more effective, and safer insect repellents could have a huge impact on global health. Wiltschko has nothing to announce yet, but says this research is ongoing in collaboration with the Bill & Melinda Gates Foundation.
Applying POM to mosquitos is also a proof of concept, says Hennek. “We can picture applying our product not just to what mosquitoes don’t like, but to what roaches don’t like. Or any number of agricultural pests.”
Capturing smell forever
Looking further down the road, Wiltschko’s vision is to digitize our sense of smell. The idea is not as far-fetched as it sounds. Consider several hundred years ago. The idea that a visual moment — the fleeting expression on your child’s face or an orchard of apple trees in blossom — could be instantly captured and made available forever more in perfect color would have been nothing short of magical thinking.
By the 1820s came the first photography, and with it, the first steps towards human mastery of the world of light. Today, it feels like a fundamental right to freeze those visual memories and hold on to them forever. And the same goes for the auditory world.
“We know what’s required to digitize a human sense,” says Wiltschko. “And we don't have to wait for any of the inventions that vision did — particularly integrated circuits.”
Indeed, with modern computing power and the harnessing of machine learning, Wiltschko reckons computers will have a “sense of smell” within a decade or two. Three stages are required: “reading” smell, understanding it, and “writing” it. Osmo wants to understand, and ultimately curate a wide palette of safe, synthetic molecules that can recreate the entire human smellscape. The reading (sensing) of odorous molecules currently requires bulky and expensive lab equipment, such as a gas chromatography mass spectrometer, while the writing (producing) of smells on demand remains science fiction at the consumer level, says Wiltschko, for now.
A window to the inside
Sensing and understanding odor at a high level may be sufficient to herald powerful health applications, says Wiltschko. For example, it is well established that serious illnesses, including some cancers, can be detected through their effect on your breath. Being able to take a snapshot of that odor profile — an “Osmograph”, in Wiltschko’s words – could reveal a great deal about what’s going on inside our bodies.
“We don't know if that technology will ultimately have a transformative effect on healthcare, but I am betting that it will,” he says.
It’s very important to Wiltschko that, down the line, Osmo grows to develop clinical diagnostics applications. “That's the North Star for me, and it's very important that we get there. But the sheer cost and the talent that's required is rare and expensive,” he says. “So, it can’t be the first beach that we storm.”
As Osmo grows, it will be looking for similarly passionate people to push the mission forward. “We've been finding that there are people out there who are secret scent lovers, who secretly aspire to work in the field of machine olfaction,” says Wiltschko. “Just to put it out there: there's one place to do this, and it's Osmo.”
Talking to Wiltschko and those inspired to work alongside him, it is clear to see that Osmo is the culmination of his lifelong passions. For him, it’s emotional. “Once you smell a thing, you cannot stop the feelings that you get from it. There's a very fundamental feeling and emotional component,” he says, “and I think that’s beautiful.”