Recommender systems are everywhere. Our choices in online shopping, television, and music are supported by increasingly sophisticated algorithms that use our previous choices to offer up something else we are likely to enjoy. They are undoubtedly powerful and useful, but television and music recommenders in particular have something of an Achilles heel — key information is often missing. They have no idea what you are in the mood for at this moment, for example, or who else might be in the room with you.
Since 2018, Amazon Music customers in the US who aren’t sure what to choose have been able to converse with the Alexa voice assistant. The idea is that Alexa gathers the crucial missing information to help the customer arrive at the right recommendation for that moment. The technical complexity of this challenge is hard to overstate, but progress in machine learning (ML) at Amazon has recently made the Alexa music recommender experience even more successful and satisfying for customers. And given that Amazon Music has more than 55 million customers globally, the potential customer benefit is enormous.
But first, how does it work? There are many pathways to the Amazon Music recommender experience, but the most direct is by saying “Alexa, help me find music” or “Alexa, recommend some music” to an Alexa-enabled device. Alexa will then respond with various questions or suggestion-based prompts, designed to elicit what the customer might enjoy. These prompts can be open-ended, such as “Do you have anything in mind?”, or more guided, such as “Something laid back? Or more upbeat?”
With this sort of general information gathered from the customer in conversational turns, Alexa might then suggest a particular artist, or use a prompt that includes a music sample from the millions of tracks available to Amazon Music subscribers. For example: “How about this? <plays snippet of music> Did you like it?” The conversation ends when a customer accepts the suggested playlist or station or instead abandons the interaction.
Early versions of the conversational recommender were, broadly speaking, based on a rule-based dialogue policy, in which certain types of customer answers triggered specific prompts in response. In the simplest terms, these conversations could be thought of as semi-scripted, albeit a dynamic script with countless possible outcomes.
“That approach worked, but it was very hard to evaluate how we could make the conversation better for the customer,” says Francois Mairesse, an Amazon Music senior machine learning scientist. “Using a rule-based system, you can find out if the conversation you designed is successful or not, thanks to the customer outcome data, but you can’t tell what alternative actions you could take to make the conversation better for customers in the future, because you didn't try them.”
A unique approach
So the Amazon Music Conversations team developed the next-generation of conversation-based music recommender, one that harnesses ML to bring the Alexa music recommender closer to being a genuine, responsive conversation. “This is the first customer-facing ML-based conversational recommender that we know of,” says team member Tao Ye, a senior applied science manager. “The Alexa follow-up prompts are not only responding more effectively for the customer, but also taking into account the customer's listening history.”
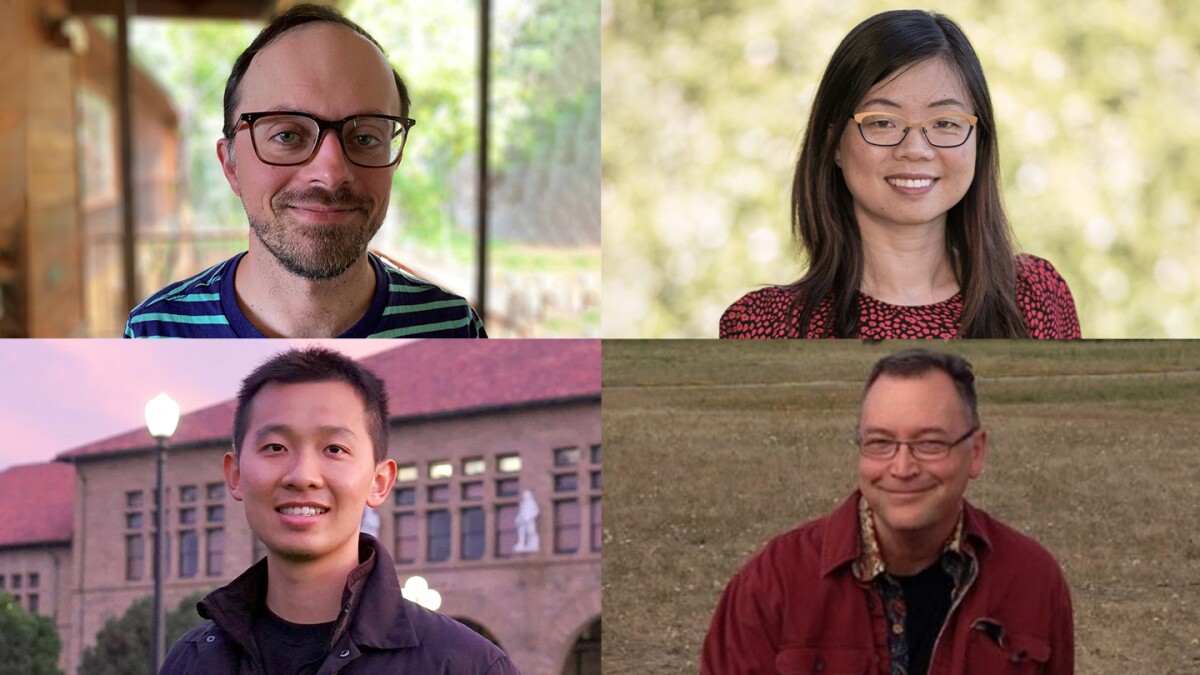
These two aspects — improved conversational efficiency and the power of incorporating the customer’s history — were explored in two ML successive experiments carried out by the Music Conversations team. The work was outlined in a conference paper presented at the 2021 ACM Conference on Recommender Systems in September.
As a starting point, the team crafted a version of the “Alexa, help me find music” browsing experience in which the questions asked by Alexa were partially randomized. That allowed the team to collect entirely anonymized data from 50,000 conversations, with a meaning representation for each user utterance and Alexa prompt. That data then helped the team estimate whether each Alexa prompt was useful or not — without a human annotator in the loop — by assessing whether the music attribute(s) gathered from a question helped find the music that was ultimately played by the user.
From the outset, the team utilized offline reinforcement learning to learn to select the question deemed the most useful at any point in the conversation. In this approach, the ML system aims to optimize scores generated by a customer’s conversation with Alexa, also known as the “reward”. When a given prompt contributed directly to finding the musical content that a customer ultimately selected and listened to, it receives a “prompt usefulness” reward of 1. Prompts that did not contribute to the ultimate success of a conversation receive a reward of 0. The ML system sought ways to maximize these rewards, and created a dialogue policy based on a dataset associating each Alexa prompt with its usefulness.
Continuous improvement
But that was just the first step. Next, the team focused on continuously improving their ML model. That entails working out how to improve the system without exposing large numbers of customers to a potentially sub-optimal experience.
“The whole point of offline policy optimization is that it allows us to take data from anonymized customer conversations and use it to do experiments offline, with no users, in which we are exploring what a new, and hopefully better, dialogue policy might produce,” Mairesse explained.
That leads to a question: How can you evaluate the effectiveness of a new dialogue policy if you only have data from conversations based on the existing policy? The goal: work out counterfactuals, i.e. what would have happened had Alexa chosen different prompts. To gather the data to make counterfactual analysis possible, the team needed to insert randomization into a small proportion of anonymized customer conversation sessions. This meant the system did not become fixated on always selecting the prompt considered to be most effective, and instead, occasionally probed for opportunities to make new discoveries.
“Let's say there's a prompt that the system expects has only a 5% chance of being the best choice. With randomization activated, that prompt might be asked 5% of the time, instead of never being asked at all. And if it delivers an unexpectedly good result, that’s a fantastic learning opportunity,” explains Mairesse.
In this way, the system collects sufficient data to fuel the counterfactual analysis. Only when confidence is high that a new dialogue policy will be an improvement on the last will it be presented to some customers and, if it proves as successful as expected, it is rolled out more broadly and becomes the new default.
An early version of the ML-based system focused on improving the question/prompt selection. When its performance was compared with the Amazon Music rule-based conversational recommender, it increased successful customer outcomes by 8% while shortening the number of conversational turns by 20%. The prompt that the ML system learned to select the most was “Something laid back? Or more upbeat?”
Improving outcomes
In a second experiment, the ML system also considered each customer’s listening history when deciding which music samples to offer. Adding this data increased successful customer outcomes by a further 4%, and the number of conversational turns dropped by a further 13%. In this experiment, which was better tailored to the affinities of individual customers, the type of prompt that proved most useful featured genre-related suggestions. For example, “May I suggest some alternative rock? Or perhaps electronic music?”
“In both of these experiments, we were only trying to maximize the prompt usefulness reward,” emphasizes team member Zhonghao Luo, an Amazon Music applied scientist. “We did not aim to reduce the length of the conversation, but that was an experimental result that we observed. Shorter conversations are associated with better conversations and recommendations from our system.”
The average Alexa music recommender conversation comprises roughly four Alexa prompts and customer responses, but not everyone wants to end the conversation so soon, says Luo. “I've seen conversations in which the customer is exploring music, or playing with Alexa, reach close to 100 turns!”
And this variety of customer goals is built into the system, Ye adds: “It's not black and white, where the system decides it’s asked enough questions and just starts offering music samples. The system can take the lead, or the customer can take the lead. It's very fluid.”
Looking ahead
While the ML-led improvements are already substantial, the team says there is plenty of scope to do more in future. “We are exploring reward functions beyond ‘prompt usefulness’ in a current project, and also which conversational actions are better for helping users reach a successful playback,” says Luo.
The team is also exploring the potential of incorporating sentiment analysis — picking up how a customer is feeling about something based on what they say and how they say it. For example, there’s a difference between a customer responding “Hmm, OK”, “Yes”, “YES!” or “Brilliant, I love it” to an Alexa suggestion.
The conversational experience adapts the response phrasing and tone-of-voice as the conversation progresses to provide a more empathetic conversational experience for the user. “We estimate how close the customer is getting to the goal of finding their music based on a number of factors that include the sentiment of past responses, estimates on how well we understood them, and how confident we are that the sample candidates match their desires,” explained Ed Bueche, senior principal engineer for Amazon Music.
Those factors are rolled into a score that is used to adjust the empathy of the response. “In general, our conversational effort strives to balance cutting edge science and technology with real customer impact,” Bueche said. “We’ve had a number of great partnerships with other research, UX, and engineering teams within Amazon.”